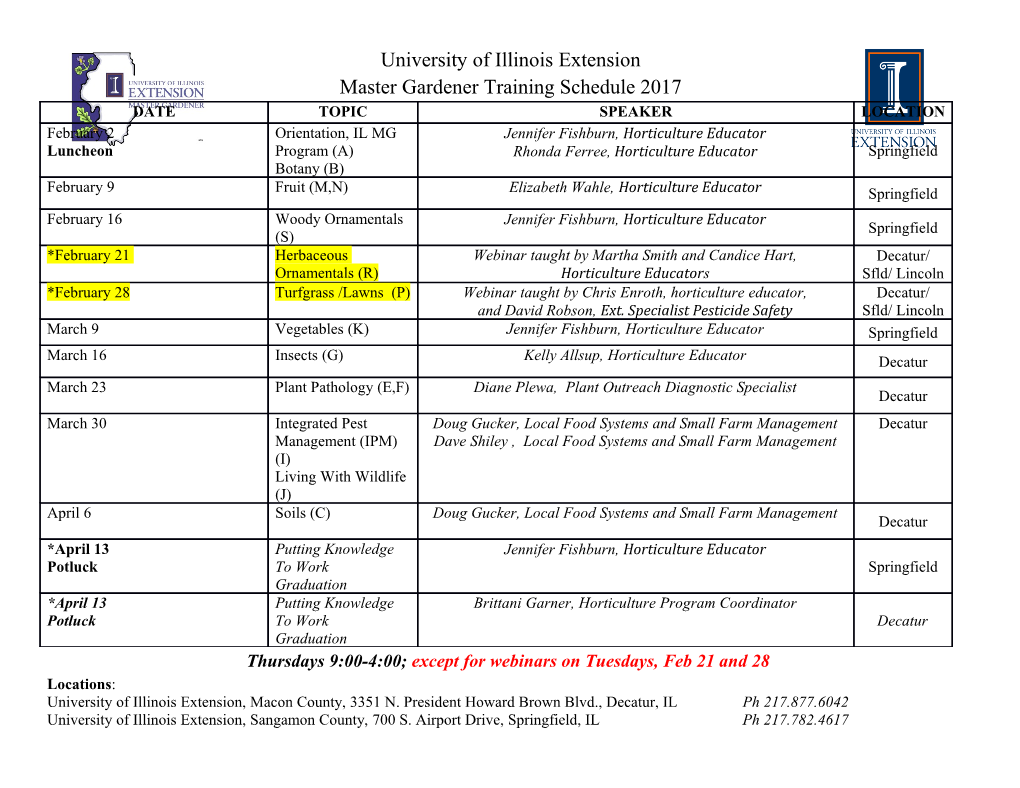
New Developments in Statistical Information Theory Based on Entropy and Divergence Measures Edited by Leandro Pardo Printed Edition of the Special Issue Published in Entropy www.mdpi.com/journal/entropy New Developments in Statistical Information Theory Based on Entropy and Divergence Measures New Developments in Statistical Information Theory Based on Entropy and Divergence Measures Special Issue Editor Leandro Pardo MDPI • Basel • Beijing • Wuhan • Barcelona • Belgrade Special Issue Editor Leandro Pardo Universidad Complutense de Madrid Spain Editorial Office MDPI St. Alban-Anlage 66 4052 Basel, Switzerland This is a reprint of articles from the Special Issue published online in the open access journal Entropy (ISSN 1099-4300) from 2017 to 2019 (available at: https://www.mdpi.com/journal/entropy/special issues/Divergence Measures) For citation purposes, cite each article independently as indicated on the article page online and as indicated below: LastName, A.A.; LastName, B.B.; LastName, C.C. Article Title. Journal Name Year, Article Number, Page Range. ISBN 978-3-03897-936-4 (Pbk) ISBN 978-3-03897-937-1 (PDF) c 2019 by the authors. Articles in this book are Open Access and distributed under the Creative Commons Attribution (CC BY) license, which allows users to download, copy and build upon published articles, as long as the author and publisher are properly credited, which ensures maximum dissemination and a wider impact of our publications. The book as a whole is distributed by MDPI under the terms and conditions of the Creative Commons license CC BY-NC-ND. Contents About the Special Issue Editor ...................................... vii Leandro Pardo Reprinted from: Entropy 2019, 391, 21, doi:10.3390/e21040391 ..................... 1 Abhik Ghosh and Ayanendranath Basu A Generalized Relative (α, β)-Entropy:Geometric Properties and Applications to Robust Statistical Inference Reprinted from: Entropy 2018, 347, 20, doi:10.3390/e20050347 ..................... 8 Yuefeng Wu and Giles Hooker Asymptotic Properties for Methods Combining the Minimum Hellinger Distance Estimate and the Bayesian Nonparametric Density Estimate Reprinted from: Entropy 2018, 20, 955, doi:10.3390/e20120955 ..................... 40 Elena Castilla, Nirian Mart´ın, Leandro Pardo and Kostantinos Zografos Composite Likelihood Methods Based on Minimum Density Power Divergence Estimator Reprinted from: Entropy 2018, 20, 18, doi:10.3390/e20010018 ..................... 60 Michel Broniatowski, Jana Jureˇckov´a, M. Ashok Kumar and Emilie Miranda Composite Tests under Corrupted Data Reprinted from: Entropy 2019, 21, 63, doi:10.3390/e21010063 ..................... 80 Osamah Abdullah Convex Optimization via Symmetrical Holder¨ Divergence for a WLAN Indoor Positioning System Reprinted from: Entropy 2018, 20, 639, doi:10.3390/e20090639 .....................103 Michel Broniatowski, Jana Jureˇckov´a and Jan Kalina Likelihood Ratio Testing under Measurement Errors Reprinted from: Entropy 2018, 20, 966, doi:10.3390/e20120966 .....................117 M. Virtudes Alba-Fern´andez, M. Dolores Jim´enez-Gamero and F. Javier Ariza-L´opez Minimum Penalized φ-Divergence Estimation under Model Misspecification Reprinted from: Entropy 2018, 20, 329, doi:10.3390/e20050329 .....................126 Marianthi Markatou and Yang Chen Non-Quadratic Distances in Model Assessment Reprinted from: Entropy 2018, 20, 464, doi:10.3390/e20060464 .....................141 Maria Kateri φ-Divergence in Contingency Table Analysis Reprinted from: Entropy 2018, 20, 324, doi:10.3390/e20050324 .....................161 Takayuki Kawashima and Hironori Fujisawa Robust and Sparse Regression via γ-Divergence Reprinted from: Entropy 2017, 19, 608, doi:10.3390/e19110608 .....................173 Chunming Zhang and Zhengjun Zhang Robust-BD Estimation and Inference for General Partially Linear Models Reprinted from: Entropy 2017, 19, 625, doi:10.3390/e19110625 .....................194 v Aida Toma and Cristinca Fulga Robust Estimation for the Single Index Model Using Pseudodistances Reprinted from: Entropy 2018, 20, 374, doi:10.3390/e20050374 .....................223 Lei Li, Anand N. Vidyashankar, Guoqing Diao and Ejaz Ahmed Robust Inference after Random Projections via Hellinger Distance for Location-Scale Family Reprinted from: Entropy 2019, 21, 348, doi:10.3390/e21040348 .....................243 Xiao Guo and Chunming Zhang Robustness Property of Robust-BD Wald-Type Test for Varying-Dimensional General Linear Models Reprinted from: Entropy 2018, 20, 168, doi:10.3390/e20030168 .....................283 Kei Hirose and Hiroki Masuda Robust Relative Error Estimation Reprinted from: Entropy 2018, 20, 632, doi:10.3390/e20090632 .....................311 vi About the Special Issue Editor Leandro Pardo has been a Full Professor at the Department of Statistics and Operational Research, Faculty of Mathematics, Complutense University of Madrid, Spain, since 1993. He holds a Ph.D. in Mathematics and has been leading research projects since 1987. He is the author of over 250 research paper in refereed statistical journals, such as the Journal of Multivariate Analysis, Biometrics, Bernoulli, Journal of Statistical Planning and Inference, Entropy, IEEE Transactions on Information Theory, Biometrical Journal, Statistics and Computing, Advances in Data Analysis and Classification, TEST, Psychometrika, Sankhya (The Indian Journal of Statistics), Annals of the Institute of Statistical Mathematics, Statistics & Probability Letters, Journal of Nonparametric Statistics, Australian and New Zealand Journal of Statistics, Statistica Neerlandica, Statistica Sinica, among others. He has published more than 10 academic books and a research book: “Statistical Inference Based on Divergence measures” (Chapman & Hall/CRC). He was the Editor in Chief for TEST between 2005 and 2008 and an Associate Editor for the Journal of Multivariate Analysis, Journal of Statistical Planning and Inference, TEST, Communications in Statistics (Theory and Methods), Communications in Statistics (Simulation and Computation) and Revista Matem´atica Complutense. He has been a scientific visitor to some Universities. In 2004, Professor Pardo was elected as the “Distinguished Eugene Lukacs Professor” at the Booling Green University (Booling, Green, Ohio) https://en.wikipedia.org/wiki/Lukacs Distinguished Professor. He has been the Chair of the Department of Statistics and Operational Research, Faculty of Mathematics, and was the President of the Spanish Society of Statistics and Operations Research (SEIO) between 2013 and 2016. vii entropy Editorial New Developments in Statistical Information Theory Based on Entropy and Divergence Measures Leandro Pardo Department of Satistics and Operation Research, Faculty of Mathematics, Universidad Complutense de Madrid, 28040 Madrid, Spain; [email protected] Received: 28 March 2019; Accepted: 9 April 2019; Published: 11 April 2019 In the last decades the interest in statistical methods based on information measures and particularly in pseudodistances or divergences has grown substantially. Minimization of a suitable pseudodistance or divergence measure gives estimators (minimum pseudodistance estimators or minimum divergence estimators) that have nice robustness properties in relation to the classical maximum likelihood estimators with a not significant lost of efficiency. For more details we refer the monographs of Basu et al. [1] and Pardo [2]. Parametric test statistics based on the minimum divergence estimators have also given interesting results in relation to the robustness in comparison with the classical likelihood ratio test, Wald test statistic and Rao’s score statistic. Worthy of special mention are the Wald-type test statistics obtained as an extension of the classical Wald test statistic. These test statistics are based on minimum divergence estimators instead of the maximum likelihood estimators and have been considered in many different statistical problems: Censoring, see Ghosh et al. [3], equality of means in normal and lognormal models, see Basu et al. [4,5], logistic regression models, see Basu et al. [6], polytomous logistic regression models, see Castilla et al. [7], composite likelihood methods, see Martín et al. [8], etc. This Special Issue focuses on original and new research based on minimum divergence estimators, divergence statistics as well as parametric tests based on pseudodistances or divergences, from a theoretical and applied point of view, in different statistical problems with special emphasis on efficiency and robustness. It comprises 15 selected papers that address novel issues, as well as specific topics illustrating the importance of the divergence measures or pseudodistances in statistics. In the following, the manuscripts are presented in alphabetical order. The paper, “A Generalized Relative (α, β)-Entropy Geometric properties and Applications to Robust Statistical Inference”, by A. Ghosh and A. Basu [9], proposes an alternative information theoretic formulation of the logarithmic super divergence (LSD), Magie et al. [10], as a two parametric generalization of the relative α−entropy, which they refer as the general (α, β)-entropy. The paper explores its relation with various other entropies and divergences, which also generates a two-parameter extension of Renyi entropy measure as a by-product. The paper is primarily focused on the geometric properties of the relative (α, β)-entropy or the LSD measures: Continuity and convexity in both the arguments along with an extended Pythagorean relation
Details
-
File Typepdf
-
Upload Time-
-
Content LanguagesEnglish
-
Upload UserAnonymous/Not logged-in
-
File Pages346 Page
-
File Size-