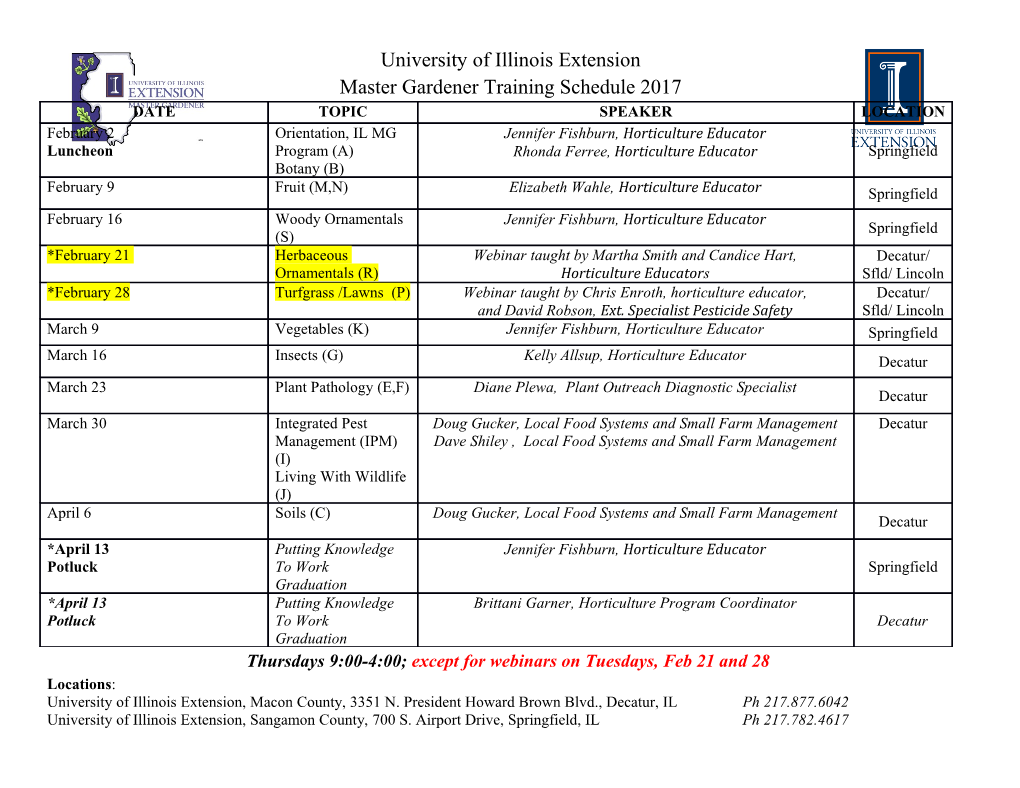
The Pennsylvania State University The Graduate School USING DUAL-POLARIZATION RADAR INFORMATION TO INVESTIGATE CLEAR-AIR BOUNDARY LAYER ATMOSPHERIC PHENOMENA A Thesis in Meteorology by John R. Banghoff c 2019 John R. Banghoff Submitted in Partial Fulfillment of the Requirements for the Degree of Master of Science May 2019 The thesis of John R. Banghoff was reviewed and approved∗ by the following: David J. Stensrud Professor of Meteorology Head of the Department of Meteorology Thesis Co-Advisor Matthew R. Kumjian Assistant Professor of Meteorology Thesis Co-Advisor George S. Young Professor of Meteorology Paul M. Markowski Professor of Meteorology Associate Head of Graduate Program ∗Signatures are on file in the Graduate School. Abstract Dual-polarization radar provides a wealth of new information about the type, size, and orientation of scat- terers in the atmosphere. This radar information has been interrogated for its applications to hazardous weather, but a wealth of clear-air radar data exists that is significantly underutilized. The ability of Na- tional Weather Service WSR-88D radars to detect insects and other biota within the convective boundary layer (CBL) facilitates estimation of boundary layer depth and characterization of horizontal convective rolls (HCRs). Bragg scatter signatures in dual-polarization radar observations, which are defined by low differen- tial reflectivity (ZDR) values, are used as a proxy for CBL depth in 2014 over Central Oklahoma using data from the Twin Lakes (KTLX) WSR-88D. The 243 ZDR Bragg scatter and upper air sounding CBL depth estimates collected during this year have a correlation of 0.90 and a RMSE of 254 m. Additionally, a 10-year climatology of HCRs in Central Oklahoma indicates that HCRs occur on 75% of days during all months of the warm-season (April-September). HCRs typically form in the mid-morning and may persist throughout the day, transition to cellular convection, or develop from cellular convection before dissipating around sun- set. These results should facilitate future studies on convection initiation, HCR formation mechanisms, and model parameterization. The methods used to estimate CBL depth and identify and characterize HCRs are potentially applicable across a variety of geographic locations and seasons, and demonstrate the usefulness of clear-air radar data. iii Table of Contents List of Figures vi List of Tables ix Acknowledgments x Chapter 1 Introduction 1 Chapter 2 Convective Boundary Layer Depth Estimation From S-Band Dual-Polarization Radar 3 2.1 Introduction . 3 2.2 Background . 4 2.3 Rawinsonde estimates of CBL depth . 5 2.4 Weather-radar based estimates of CBL depth . 6 2.5 Verification of WSR-88D-based estimates of CBL depth . 11 2.5.1 Application to locations outside of Oklahoma . 13 2.6 Operational implications and future work . 14 Chapter 3 A 10-year Warm-Season Climatology of Horizontal Convective Rolls and Cellular Con- vection in Central Oklahoma 18 3.1 Introduction . 18 3.2 Methods for Detection of Boundary Layer Circulations . 20 3.2.1 Identifying Boundary Layer Organization . 21 3.2.2 Differentiating HCRs from Cellular Convection . 23 3.2.3 HCR Characteristics . 24 3.3 Results . 24 3.3.1 10-Year Climatology . 24 3.3.2 Results from 2013-2017 . 25 3.4 Summary and Future Work . 29 Chapter 4 Conclusion 46 iv Bibliography 48 v List of Figures 2.1 Vertical profiles from the 0000 UTC KOUN sounding on 26 Sept. 2014 showing refractivity (N), potential temperature (θ), water vapor mixing ratio (q), and virtual potential tempera- ture (θv). The red dashed line represents estimated CBL depth based on maximum vertical gradients in each variable. 6 2.2 Plan Position Indicator (PPI) of differential reflectivity (in dB, shaded according to scale) at 4:44◦ elevation on 11 May 2015 at 2345 UTC from the KTLX radar. Note the clear ring of lower ZDR values (blue color) indicative of a Bragg scatter layer. The radar is located at the origin. 7 2.3 Quasi-vertical profiles of Z and ZDR for 20-21 May 2014 at KTLX. Note the daytime evolution of the CBL top characterized by reduced ZDR and the biota bloom overnight characterized by large Z and ZDR.......................................... 9 2.4 Representation of Bragg scatter ZDR contamination by biota. For a given insect ZDR, an increase in the amount of insects leads to an increase in ZDR of Bragg scatter from 0 dB. The black contour in each plot represents the ratio between horizontal reflectivities for bugs and Bragg scatter resulting in a decrease in the ZDR of bugs by 0.5 dB. Such a difference is detectable by the algorithm used in the present study to estimate CBL depth from QVPs of ZDR................................................... 10 2.5 Time-height depiction of quasi-vertical profiles (QVP) of ZDR (in dB, shaded according to scale) at the 4:5◦ elevation angle for (a) 4 June 2014 and (b) 21 October 2014 at KTLX. Note the region of Bragg scatter characterized by low ZDR values. The estimated time series of minimum ZDR is represented by a white line (solid when clearly defined and dashed when interpolation is required). 11 2.6 Comparison of radar- and rawinsonde-derived CBL depth estimations for all usable days in 2014. 12 2.7 ZDR QVP for 9 February 2017 in Minneapolis, MN (KMPX), 11 March 2017 Fairbanks, AK (PAPD), 7 May 2017 in Portland, OR (KRTX), 11 June 2017 in Albany, NY (KENX), 17 August 2017 in Tucson, AZ (KEMX), 4 September 2017 in Riverton, WY (KRIW), 2 October 2017 in Wilmington , OH (KILN), and 21 December 2016 in Tampa, FL (KTBW). The time series of minimum ZDR is manually traced with a white line. Rawinsonde estimates of CBL depth at 23 UTC are indicated by the black dot outlined in yellow. White shading indicates regions outside the temporal and spatial range of radar data. 16 2.8 ZDR QVP (left) for 15 July 2014 and 30 November 2014 in Central Oklahoma (KTLX) showing a double layer of Bragg scatter. Legend is the same as Figure 2.7 excluding the daytime CBL depth estimate for clarity. Vertical profiles of refractivity, potential tempera- ture, mixing ratio, and virtual potential temperature (right) demonstrate vertical gradients of moisture/temperature characteristic of Bragg scatter signatures on radar. 17 vi 3.1 Equivalent reflectivity factor at 1.4◦ (a) at 2142 UTC on 16 June 2014 at KTLX, showing a classic HCR signature with clearly-defined linear echoes and (b) at 1836 UTC on 29 September 2014 at KTLX, showing a classic cellular convection signature with clearly-defined circular echoes. Such echoes occur as the radar scans insects lofted in updrafts associated with HCRs. The associated absence of echoes represent locations of downdrafts where insects are vertically suppressed. 21 3.2 0.5◦ Z (dBZ), 0.5◦ V (m s−1), and velocity azimuth display (VAD) computed between 35 and 45 km from KTLX during (a-c) a supercell thunderstorm on 1 June 2016, (d-f) a nocturnal boundary layer on 11 June 2016, and (g-i) HCRs on 22 June 2016. The variance (σ2) of residual radial velocity (observed winds minus VAD-estimated winds) is listed on each VAD plot. Notice the residual radial velocity variance (RRVV) is largest for the precipitation case and lowest for the HCR case. Lower variance implies increased uniformity of boundary layer winds. 33 3.3 Time series of of residual radial velocity variances (RRVVs, m2 s−2) from VAD analysis for 29 July 2014 at KTLX. Velocity azimuth displays and RRVVs are calculated for range rings of 10, 20, 30, and 40 km distance from the radar (beam heights of 0.27, 0.53, 0.80, and 1.08 km respectively). The red bar indicates the algorithm-identified time range of boundary layer organization. Organization is defined when the variance among the 4 VAD RRVVs drops below 1 m4 s−4 implying a convergence of the various time series. The black bar indicates manually-identified boundary layer organization. 34 3.4 Comparison of VAD RRVV analysis and manual analysis for start and end times of boundary layer organization. Organization departure is computed as TRRV V -Tmanual where T<0 indicates RRVV analysis times are earlier than manually-identified times. Note that timing from the RRVV method over-estimates the range of actual organization (i.e. RRVV analysis has earlier start times and later end times than found from the manual analysis). Departures for (a) start times and (b) end times from 134 days with organization in 2014 are included. 35 3.5 (a) Cumulative 10-year warm-season climatology of HCRs in Central Oklahoma separated by month showing the percentage of days that exhibit boundary layer organization, widespread precipitation, and the absence of boundary layer organization (null cases), along with cases deemed indiscernible (unclear). (b) For each month, boundary layer organization days are separated into HCRs (left columnn) and cellular convection (right column). Transition cases (both HCRs and cells, so doubly represented), pure HCRs/cells and a mix of organization and precipitation are delineated. Note that a majority of days in each month have HCRs, and nearly 75% of all days have boundary layer organization. 36 3.6 As in Figure 3.5, but separated by year. Data are based on visual observation of base re- flectivity between 1200 UTC and 0000 UTC from the Twin Lakes, OK (KTLX) WSR-88D radar. 37 3.7 HCR duration for April-September of 2008-2017 at KTLX. Durations are broken down in (a) all HCR cases, (b) days where HCRs are the only boundary layer organization, (c) days when HCRs undergo transition to cellular convection, and (d) days when HCRs are formed via transition from cellular convection.
Details
-
File Typepdf
-
Upload Time-
-
Content LanguagesEnglish
-
Upload UserAnonymous/Not logged-in
-
File Pages64 Page
-
File Size-