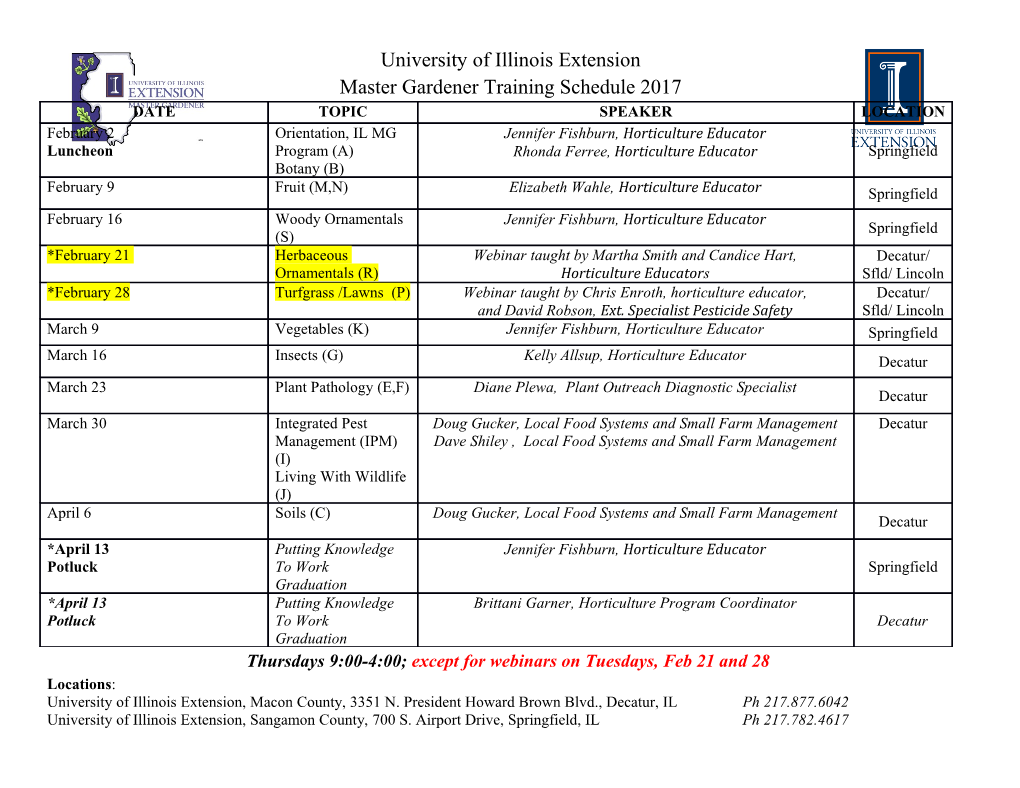
Commonsense-Based Topic Modeling Dheeraj Rajagopal Daniel Olsher Erik Cambria NUS Temasek Laboratories NUS Temasek Laboratories NUS Temasek Laboratories Cognitive Science Programme Cognitive Science Programme Cognitive Science Programme 117411, Singapore 117411, Singapore 117411, Singapore [email protected] [email protected] [email protected] Kenneth Kwok NUS Temasek Laboratories Cognitive Science Programme 117411, Singapore [email protected] ABSTRACT Firstly, in such models words in a document are considered Topic modeling is a technique used for discovering the ab- to be independent from each other, which is a very uncom- stract ‘topics’ that occur in a collection of documents, which mon scenario in practice. For example, the multi-word ex- is useful for tasks such as text auto-categorization and opin- pression “score home run”, taken as a unit, is clearly related ion mining. In this paper, a commonsense knowledge based to baseball, but word-by-word conveys totally di↵erent se- algorithm for document topic modeling is presented. In con- mantics. Similarly, in a bag-of-words model, the phrase“get- trast to probabilistic models, the proposed approach does ting fired” [25] would not convey the meaning “fired from a not involve training of any kind and does not depend on job”. Secondly, removing stopwords from documents often word co-occurrence or particular word distributions, making leads to the loss of key information, e.g., in the case of the the algorithm e↵ective on texts of any length and composi- concept “get the better of”, which would become “get better” tion. ‘Semantic atoms’ are used to generate feature vectors with all stopwords removed, or the multi-word expression for document concepts. These features are then clustered us- “let go of the joy”, which would be reduced to simply “joy”, ing group average agglomerative clustering, providing much reversing the meaning. improved performance over existing algorithms. The alternative approach we put forth here draws on knowl- Categories and Subject Descriptors edge of word meanings, encoded in a commonsense knowl- I.2.7 [Artificial Intelligence]: Natural Language Process- edge database structured in the INTELNET formalism [32], ing—Text Analysis to provide enhanced topic modeling capabilities. In contrast to probabilistic models, our approach does not involve train- ing of any kind and does not depend on word co-occurrence General Terms or particular word distributions, making the algorithm ef- Algorithms fective on texts of any length and composition. Keywords Commonsense knowledge, defined as the basic understand- AI, NLP, KR, Topic Modeling, Commonsense Knowledge ing of the world humans acquire through day-to-day life, includes information such as “one becomes elated when one sees one’s ideas become reality” and “working to be healthy 1. INTRODUCTION is a positive goal”. Statistical text models can identify email Topics, defined as distributions over words [7], facilitate spam and find syntactic patterns in documents, but fail to keyword-based text mining, document search, and meaning- understand poetry, simple stories, or even friendly e-mails. based retrieval [4]. Probabilistic topic models, such as la- Commonsense knowledge is invaluable for nuanced under- tent Dirichlet allocation [7], are used to facilitate document standing of data, with applications including textual af- queries [39], document comprehension [27], and tag-based fect sensing [24], handwritten text recognition [38], story recommendations [22]. However, typical bag-of-words prob- telling [23], situation awareness in human-centric environ- abilistic models have several shortcomings. ments [20], casual conversation understanding [12], social media management [14], opinion mining [9], and more. Permission to make digital or hard copies of all or part of this work for per- sonal or classroom use is granted without fee provided that copies are not This paper proposes a method for using commonsense knowl- made or distributed for profit or commercial advantage and that copies bear edge for topic modeling. Instead of the ‘bag-of-words’ model, this notice and the full citation on the first page. Copyrights for components we propose the bag-of-concepts [8], which considers each lex- of this work owned by others than ACM must be honored. Abstracting with ical item as an index to a set of ‘semantic atoms’ describing credit is permitted. To copy otherwise, to republish, to post on servers or to the concept referred to by that lexical item. A ‘semantic redistribute to lists, requires prior specific permission and/or a fee. atom’ is a small piece of knowledge about a particular con- WISDOM ’13, August 11 2013, Chicago, USA. Copyright 2013 ACM 978-1-4503-2332-1/13/08 ...$15.00. cept, perhaps that it tends to have a particular size or be associated with other known concepts. Taken together, the word’s creation is attributable to one of the document’s top- set of semantic atoms for a concept provides an excellent ics. In particular, for a set of N topics: picture of the practical meaning of that concept. Access to N k this knowledge permits the creation of ‘smart’ topic mod- p (w)= p (wn zn; β) p (zn ✓) p(✓; ↵)d✓ (3) eling algorithms that take semantics into account, o↵ering ✓ | | Z n=1 zn=1 ! improved performance over probabilistic models. Y X where ↵ is the Dirichlet prior parameter per document topic The paper is organized as follows: Section 2 describes re- distribution and β is the Dirichlet prior parameter per topic lated work in the field of topic modeling; Section 3 illus- word distribution. Specifically, the LDA algorithm [5] oper- trates how the bags-of-concepts are extracted from natural ates as follows: language text; Section 4 discusses the proposed topic model- ing algorithm; Section 5 presents evaluation results; finally, Section 6 concludes the paper and suggests directions for 1. For each topic, future work. (a) Draw a distribution over words !βv Dirv (⌘) ⇠ 2. For each document, 2. RELATED WORK (a) Draw a vector of topic proportion ✓d Dir ( ↵ ) In topic modeling literature, a document is usually repre- ⇠ ! sented as a word vector W = w1,w2,w3......., wn and the (b) For each word, { } corpus as a set of documents C = W1,W2,W3......., Wn , { } i. Draw a topic assignment Zd,n Mult !✓d , while the distribution of a topic z across C is usually referred ⇠ as ✓. Common approaches include mixture of unigrams, la- Zd,n 1,......K ⇣ ⌘ 2{ } tent semantic indexing (LSI), and latent Dirichlet allocation ii. Draw a word Wd,n Mult −−βz −! , (LDA). ⇠ d,n Wd,n 1,......V ⇣ ⌘ 2{ } 2.1 Mixture of Unigrams The mixture-of-unigrams model [30] is one of the earliest To perform topic modeling for a particular task, posterior in- probabilistic approaches to topic modeling. It assumes that ference is perfomed using any one of the following methods: each document covers only a single topic (an assumption mean field variational [6], expectation propagation [28], col- that does not often hold). The probability of a document in lapsed Gibbs sampling [15] , distributed sampling [29], col- this model, constrained by the aim of generating N words lapsed variational inference [37], online variational inference independently, is defined as: [18] and/or factorization-based inference [1]. k N Although LDA overcomes shortcomings such as overfitting p (W )= p (wn z) p (z)(1) | and the presence of multiple topics within documents, it still z=1 "n=1 # X Y uses the bag-of-words model and, hence, wrongly assumes where p (W ) is the probability of the document, p (wn z)is that every word in the document is independent. For this the probability of the word conditional on topic, and |p (z) reason, model performance is heavily dependent on topic is the probability of the topic. This model has the serious diversity and corpus volume. LDA and other probabilistic shortcoming of attempting to fit a single topic for the whole models, in fact, fail to deal with short texts, unless they document. are trained extensively with all the documents covering the scope of the test document. The proposed commonsense- 2.2 Latent Semantic Indexing based model, instead, does not involve training of any kind A subsequent major development in topic modeling has been and does not depend on word co-occurrence or particular achieved through LSI, most commonly in the form of Hof- word distributions, making the algorithm e↵ective on texts mann’s pLSI algorithm[19], given as: of any length and composition. k p (W, wn)=p (W ) p (wn z) p (z W )(2) | | 3. SEMANTIC PARSING z=1 X The first step to commonsense-based topic modeling is bag- where p (z d) is the probability of the topic conditional on of-concepts extracton. This is performed through a graph- | document. In pLSI, each document is modeled as a bag- based approach to commonsense concept extraction [34], of-words and each word is assumed to belong to a particu- which breaks sentences into chunks first and then extracts lar topic. The algorithm overcomes the shortcoming of the concepts by selecting the best match from a parse graph mixture-of-unigrams model by assuming that a document that maps all the multi-word expressions contained in the can cover multiple topics. However, it su↵ers from issues commonsense knowledge base. related to data overfitting. 3.1 From Sentence to Verb and Noun Chunks 2.3 Latent Dirichlet Allocation Each verb and its associated noun phrase are considered in LDA [7] is the state-of-the-art approach to topic modeling. turn, and one or more concepts is extracted from these. As It is a mixed-membership model, which posits that each doc- an example, the clause “I went for a walk in the park”, would ument is a mixture of a small number of topics and that each contain the concepts go walk and go park.
Details
-
File Typepdf
-
Upload Time-
-
Content LanguagesEnglish
-
Upload UserAnonymous/Not logged-in
-
File Pages8 Page
-
File Size-