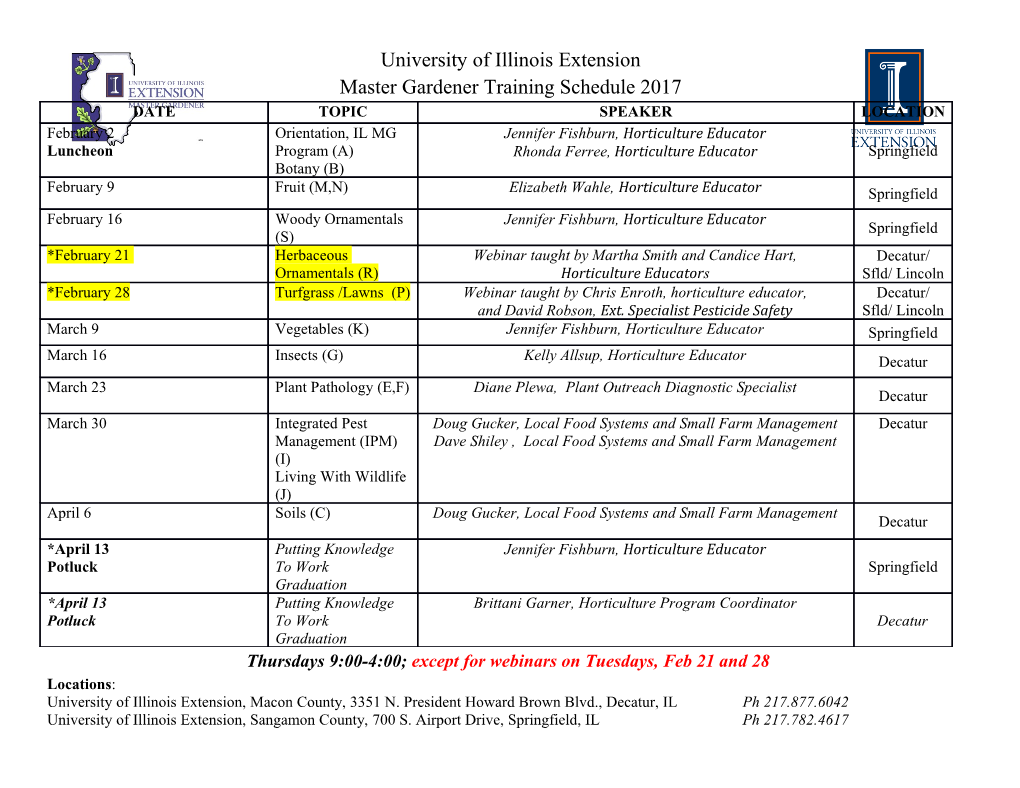
Beyond Our Behavior: The GDPR and Humanistic Personalized Recommendation Travis Greene & Galit Shmueli [email protected],[email protected] Institute of Service Science, National Tsing Hua University Hsinchu, Taiwan ABSTRACT longer acceptable given the potential effects RS can have on the Personalization should take the human person seriously. This re- person. We thus propose humanistic personalization (HP) as a novel quires a deeper understanding of how recommender systems can guide for thinking about and designing personalized RS. The HP shape both our self-understanding and identity. We unpack key paradigm of mutual, dialogic participation obliges RS designers to European humanistic and philosophical ideas underlying the Gen- promote and respect the unique capacities of persons to create and eral Data Protection Regulation (GDPR) and propose a new par- modify personal narratives over time. adigm of humanistic personalization. Humanistic personalization Our paper builds on but differs from recent work on RS value- responds to the IEEE’s call for Ethically Aligned Design (EAD) and alignment [99], self-actualization [65], qualitative evaluation met- is based on fundamental human capacities and values. Humanistic rics [76], and the epistemic and ethical problems of over-reliance personalization focuses on narrative accuracy: the subjective fit be- on behavioral data [35]. tween a person’s self-narrative and both the input (personal data) Most notably, we use the General Data Protection Regulation and output of a recommender system. In doing so, we re-frame the (GDPR) as the basis for ethical and legal norms instead of adding to distinction between implicit and explicit data collection as one of the current morass of competing principles, guidelines, and frame- nonconscious (“organismic”) behavior and conscious (“reflective”) works for Ethical AI/ML (see e.g., [79]). Tying ethical principles action. This distinction raises important ethical and interpretive to legal norms via the GDPR is valuable because, in principle, the issues related to agency, self-understanding, and political partici- GDPR applies to any data controller processing the personal data pation. Finally, we discuss how an emphasis on narrative accuracy of data subjects residing in the EU [52]. Further, law is institutionally- can reduce opportunities for epistemic injustice done to data sub- backed to achieve greater behavioral compliance via the state’s mo- jects. nopoly on the legitimate use of physical force [18, 56]. Thus our conception of HP is likely to foster greater compliance by industry 1 INTRODUCTION and researchers around the globe, even if some minor details of the GDPR have not yet been fully worked-out. In sum, we believe Machine learning-backed personalized services, among them rec- the GDPR provides a solid grounding for deep humanistic design ommender systems (RS), have become a permanent fixture in our principles relevant to RS, a view shared by a number of European increasingly digital lives. Personalization relies on vast quantities legal scholars [59, 60]. of behavioral big data (BBD): the personal data generated when hu- Second, we re-frame [35]’s distinction between implicit/explicit mans interact with apps, devices, and social networks [94]. Besides feedback as one between conscious action and non-conscious be- supplying bases for recommendations and “hypernudges” [112] to- havior and perception. This has philosophical and moral implica- wards these recommendations, BBD are the essential raw material tions for RS users. of our digital representations. But to what extent do these logged Third, we cast doubt on the meaning of the structural regularity digital behaviors truly reflect who we are as persons? of said observed behavioral data, which are encoded and parame- As life steadily moves online, the digital representations of per- terized by the machine learning (ML) algorithms used to generate arXiv:2008.13404v1 [cs.CY] 31 Aug 2020 sons take on legal and moral importance [106]. Influential Euro- recommendations. We suggest these regularities may more reflect pean legal theorists and philosophers have even written an Onlife the structure of platform affordances rather than a person’s under- Manifesto, shaping the discourse around what it means to be hu- lying preferences. In this way, debates in the philosophy of soci- man in the digital age [40]. At the same time, the IEEE has artic- ology (e.g., the structure-agency debate) may provide insights into ulated a vision of Ethically Aligned Design (EAD) that empowers areas where personalization is currently weak. A final and absolute “individuals to curate their identities and manage the ethical impli- determination of person’s “true” preferences or interests may not cations of their data” [4]. be possible–a conclusion which dovetails with postmodern theory The current domain of RS is largely focused on problems of and complexity theory [58]. The upshot of this diagnosis is that “practical identity,” which include various methods for construct- giving data subjects a constructive voice in the meaning and inter- ing, identifying, and linking various data representations of users pretation of digitally recorded events may be our best option. We [27, 70]. In contrast, philosophers and social scientists are con- introduce the legal basis supporting such a conclusion. cerned with issues of moral, social, and increasingly, the narrative identities of persons. This separation of spheres of activity is no 1.1 The Recommender Systems View 3rd FAccTRec Workshop: Responsible Recommendation, Sept 2020, 2020. ACM ISBN 978-x-xxxx-xxxx-x/YY/MM...$15.00 Conventional collaborative filtering (CF)–the dominant paradigm https://doi.org/10.1145/nnnnnnn.nnnnnnn in personalized recommendation–relies primarily on implicit data 1 3rd FAccTRec Workshop: Responsible Recommendation, Sept 2020, Greene and Shmueli collection to drive recommendations [23]. CF leverages implicit precisely capta, or the “units that have been selected and harvested data to determine a focal user’s “nearest neighbors” [16] and rec- from the sum of all potential data” [64]. ommends items bought or consumed by the “neighbors,” but not Feature sets of narrowly defined and observed (logged) behav- yet by the focal user. In many large-scale production systems, im- iors within an app or device constitute our digital representations. plicit data take the form of browsing history, rating metadata, search Yet the meaning of observed behaviors are under-determined since queries, and social graphs. Implicit data collection relieves users of they could spring from a variety of possible mental states. By leav- the task of explicitly rating items and can be easily automated and ing out mental states, social and moral identity, and narrative as- scaled [80]. Implicit data are a type of BBD. pects of human experience, BBD-based models of persons are rep- During personalization, a user is represented roughly as follows. resentations merely by “stipulative fiat” [17] of RS designers, not Database representations of a person–tabularized collections of because they capture essential aspects of a person. logged behaviors in apps or on devices–are converted into feature vectors, permitting the computation of various proximity metrics 1.3 Two Philosophical Views of Persons and between pairs of vectors. Next, “neighborhoods” are derived from Personal Data the set of neighbors in feature space deemed closest to a given user, The act of inferring personal interests through digital behavior– according to a distance metric. A 10-dimensional feature vector the ostensible goal of personalization–leads to deep philosophical represents a person as an array of 10 numbers, obtained from mea- questions. For instance a) What kind of objects are our data rep- surements of observed behavior, thereby replacing the person with resentations? and b) What is the relation between parts (measure- a single point in 10-dimensional feature space. To reduce compu- ments) and wholes (persons), especially when a global objective tational costs, the dimensions of the original user-item matrix are function is optimized? often reduced into a lower “latent space” by techniques such as Two influential accounts of how persons and personal data re- singular value decomposition (SVD). Recently, deep learning ap- late exist in the philosophy of information and marketing litera- proaches to RS have used stacked denoising autoencoders to gen- ture. Yet we find both views to be legally and morally problematic. erate latent representations of users [109]. Digital representations Our purpose in introducing these two points of view is not to at- of persons thus take the form of feature sets of narrow, observed tempt to hash out their metaphysical details, but instead to intro- behaviors within an app or device and exclude their unique mental duce readers to a new way of thinking about the complexities of states, social and moral identities and personal narratives. technologically-mediated being. We describe each view next. You (Really) are Your Data. The Oxford philosopher of information 1.2 A Humanist Critique of BBD-based RS Luciano [39] endorses what we term a “realist” view of personal data. He maintains, “You are your information,’ so that anything The behavioral focus of CF personalization exposes it to the same done to your information is done to you, not to your belongings.” criticisms of behaviorism. Behaviorism rejects the study of causal To Floridi, personal data do not represent
Details
-
File Typepdf
-
Upload Time-
-
Content LanguagesEnglish
-
Upload UserAnonymous/Not logged-in
-
File Pages15 Page
-
File Size-