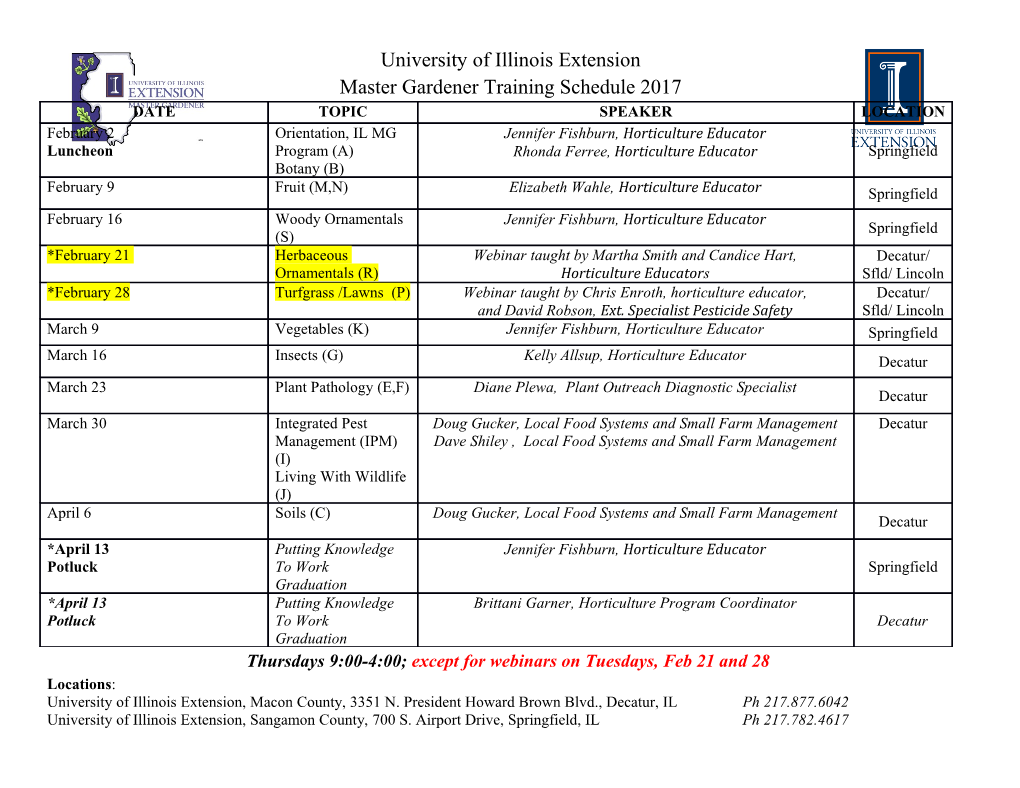
1 SEMIDEFINITE PROGRAMMING RELAXATIONS OF NON-CONVEX PROBLEMS IN CONTROL AND COMBINATORIAL OPTIMIZATION Stephen Boyd and Lieven Vandenb erghe Information Systems Laboratory, Stanford University In celebration of Tom Kailath's contributions, on his 60th birthday. DedicatedtoTom Kailath: mentor, model, col league, teacher, friend. ABSTRACT We p oint out some connections b etween applications of semide nite programming in control and in combinatorial optimization. In b oth elds semide nite programs arise as convex relaxations of NP-hard quadratic optimization problems. We also show that these relaxations are readily extended to optimization problems over bilinear matrix inequaliti es. 1 SEMIDEFINITE PROGRAMMING In a semide nite program SDP we minimize a linear function of a variable m x 2 R sub ject to a matrix inequality: T minimize c x 1.1 sub ject to F x 0 where m X x F : F x = F + i i 0 i=1 1 2 Chapter 1 m The problem data are the vector c 2 R and m + 1 symmetric matrices nn F ;:::;F 2 R . The inequality sign in F x 0 means that F xis 0 m n T p ositive semide nite, i.e., z F xz 0 for all z 2 R .We call the inequality F x 0a linear matrix inequality LMI. Semide nite programs can b e regarded as an extension of linear programming where the comp onentwise inequalities b etween vectors are replaced by matrix inequalities, or, equivalently, the rst orthant is replaced by the cone of p osi- tive semide nite matrices. Semide nite programming uni es several standard problems e.g., linear and quadratic programming, and nds many applica- tions in engineering and combinatorial optimization see [Ali95], [BEFB94 ], [VB96]. Although semide nite programs are much more general than linear programs, they are not much harder to solve. Most interior-p oint metho ds for linear programming have b een generalized to semide nite programs. As in lin- ear programming, these metho ds have p olynomial worst-case complexity, and p erform very well in practice. 2 SEMIDEFINITE PROGRAMMING AND COMBINATORIAL OPTIMIZATION Semide nite programs playavery useful role in non-convex or combinatorial optimization. Consider, for example, the quadratic optimization problem minimize f x 0 1.2 sub ject to f x 0;i=1;:::;L i T T where f x= x Ax+2b x+c , i =0;1;:::;L. The matrices A can b e i i i i i inde nite, and therefore problem 1.2 is a very hard, non-convex optimization problem. For example, it includes all optimization problems with p olynomial ob jective function and p olynomial constraints see [NN94, x6.4.4], [Sho87]. For practical purp oses, e.g., in branch-and-b ound algorithms, it is imp ortantto have go o d and cheaply computable lower b ounds on the optimal value of 1.2. Shor and others have prop osed to compute suchlower b ounds by solving the Semide nite programming relaxations 3 semide nite program with variables t and i maximize t A b A b A b 0 0 1 1 L L sub ject to + + + 0 1 L T T T b c t b c b c 0 1 L 0 1 L 0;i=1;:::;L: i 1.3 One can easily verify that this semide nite program yields lower b ounds for 1.2. Supp ose x satis es the constraints in the nonconvex problem 1.2, i.e., T x A b x i i f x= 0 i T 1 b c 1 i i for i =1;:::;L, and t, ,..., satisfy the constraints in the semide nite 1 L program 1.3. Then T x A b A b A b x 0 0 1 1 L L 0 + + + 1 L T T T 1 b c t b c b c 1 0 1 L 0 1 L = f x t + f x+ + f x 0 1 1 L L f x t: 0 Therefore t f x for every feasible x in 1.2, as desired. Problem 1.3 can 0 also b e derived via Lagrangian duality; for a deep er discussion, see Shor [Sho87], or Poljak, Rendl, and Wolkowicz [PRW94]. Most semide nite relaxations of NP-hard combinatorial problems seem to b e related to the semide nite program 1.3, or the related one, T minimize TrXA +2b x+ c 0 0 0 T sub ject to TrXA +2b x+c 0;i=1;:::;L i i i 1.4 X x 0; T x 1 k k k T where the variables are X = X 2 R and x 2 R . It can b e shown that 1.4 is the semide nite programming dual of Shor's relaxation 1.3; the two problems 1.3 and 1.4 yield the same b ound. Note that the constraint X x 0 1.5 T x 1 4 Chapter 1 T is equivalentto X xx . The semide nite program 1.4 can therefore b e directly interpreted as a relaxation of the original problem 1.2, which can b e written as T minimize TrXA +2b x+ c 0 0 0 T sub ject to TrXA +2b x+c 0;i=1;:::;L 1.6 i i i T X = xx : The only di erence b etween 1.6 and 1.4 is the replacement of the non- T T convex constraint X = xx with the convex relaxation X xx . It is also interesting to note that the relaxation 1.4 b ecomes the original problem 1.6 if we add the nonconvex constraint that the matrix on the left hand side of 1.5 is rank one. As an example, consider the 1; 1-quadratic program T T minimize x Ax +2b x 1.7 2 sub ject to x =1;i=1;:::;k; i which is NP-hard. The constraint x 2f1;1gcan b e written as the quadratic i 2 2 equality constraint x = 1, or, equivalently,astwo quadratic inequalities x 1 i i 2 T and x 1. Applying 1.4 we nd that the semide nite program in X = X i and x T minimize TrXA +2b x sub ject to X =1;i=1;:::;k ii 1.8 X x 0 T x 1 yields a lower b ound for 1.7. In a recent pap er on the MAX-CUT problem, which is a sp eci c case of 1.7 where b = 0 and the diagonal of A is zero, Go emans and Williamson have proved that the lower b ound from 1.8 is at most 14 sub optimal see [GW94] and [GW95]. This is much b etter than any previously known b ound. Similar strong results on semide nite programming relaxations of NP-hard problems have b een obtained by Karger, Motwani, and Sudan [KMS94]. The usefulness of semide nite programming in combinatorial optimization was recognized more than twentyyears ago see, e.g., Donath and Ho man [DH73]. Many p eople seem to have develop ed similar ideas indep endently.We should however stress the imp ortance of the work by Grotschel, Lov asz, and Schrij- ver [GLS88, Chapter 9], [LS91] who have demonstrated the p ower of semidef- inite relaxations on some very hard combinatorial problems. The recent de- velopment of ecientinterior-p oint metho ds has turned these techniques into Semide nite programming relaxations 5 powerful practical to ols; see Alizadeh [Ali92b, Ali91, Ali92a], Kamath and Kar- markar [KK92, KK93], Helmb erg, Rendl, Vanderb ei and Wolkowicz [HRVW94]. For a more detailed survey of semide nite programming in combinatorial opti- mization, we refer the reader to the recent pap er by Alizadeh [Ali95]. 3 SEMIDEFINITE PROGRAMMING AND CONTROL THEORY Semide nite programming problems arise frequently in control and system theory; Boyd, El Ghaoui, Feron and Balakrishnan catalog many examples in [BEFB94 ]. We will describ e one simple example here. Consider the di erential inclusion dx = Axt+But; yt=Cxt; ju tj jy tj;i=1;:::;p 1.9 i i dt l p p where xt 2 R , ut 2 R , and y t 2 R . In the terminology of control theory, this is describ ed as a linear system with uncertain, time-varying, unity- b ounded, diagonal feedback. We seek an invariant ellipsoid, i.e., an ellipsoid E such that for any x and u that satisfy 1.9, xT 2E implies xt 2E for all t T . The existence of such an ellipsoid implies, for example, that all solutions of the di erential inclusion 1.9 are b ounded. T T The ellipsoid E = fx j x Px 1g, where P = P > 0, is invariant if and T only if the function V t=xt Pxt is nonincreasing for any x and u that satisfy 1.9. In this case wesay that V is a quadratic Lyapunov function that proves stability of the di erential inclusion 1.9. We can express the derivativeof V as a quadratic form in xt and ut: T T d xt A P + PA PB xt V xt = : 1.10 T ut B P 0 ut dt We can express the conditions ju tj jy tj as the quadratic inequalities i i T T xt c c 0 xt i 2 2 i t y t= 0; i =1;:::;p; u i i ut 0 E ut ii 1.11 6 Chapter 1 where c is the ith rowofC, and E is the matrix with all entries zero except i ii the ii entry, whichis1.
Details
-
File Typepdf
-
Upload Time-
-
Content LanguagesEnglish
-
Upload UserAnonymous/Not logged-in
-
File Pages10 Page
-
File Size-