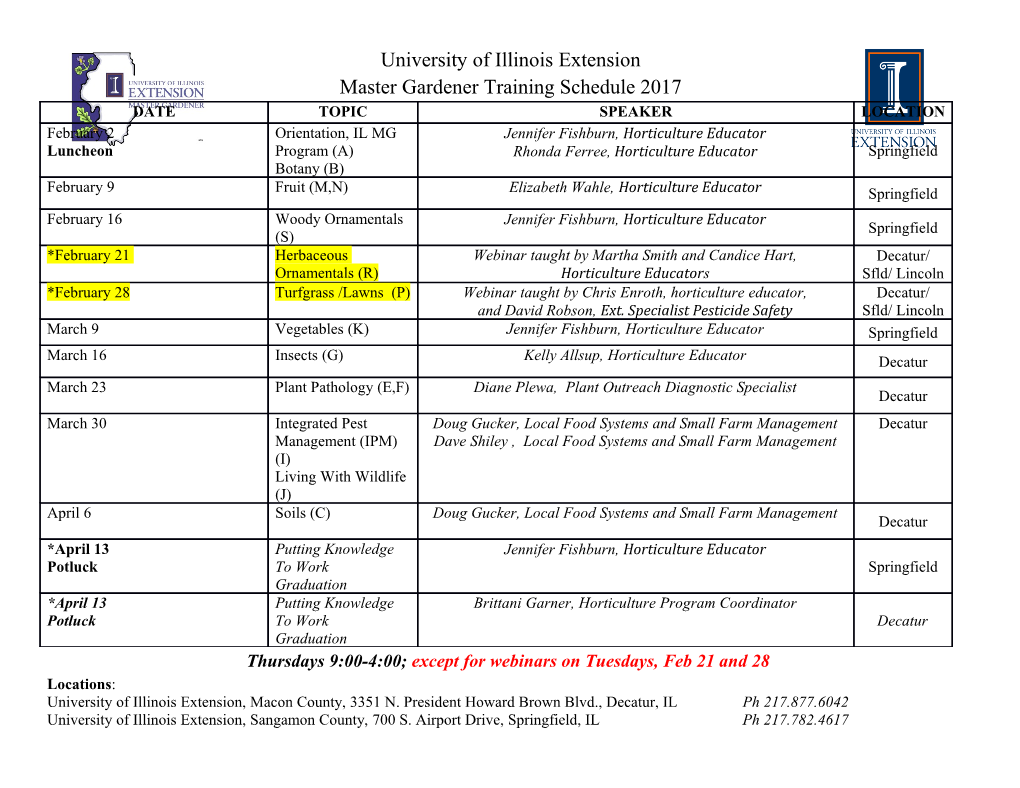
Bilateral filtering Bilateral Filters Input Gaussian Log(Intensity) Digital Visual Effects Smoothing Bilateral Smoothing Yung-Yu Chuang with slides by Fredo Durand, Ramesh Raskar, Sylvain Paris, Soonmin Bae [Ben Weiss, Siggraph 2006] Imaggge Denoising A Wide Ranggpe of Options • Diffusion, Bayesian, Wavelets… – All have their pros and cons. • Bila tera l filter – not always the best result [Buades 05] but often good – easy to understand, adapt and set up noisy image naïve denoising better denoising Gaussian blur edge-preserving filter Smoothing an image without blurring its edges. Basic denoising Basic denoising Noisy input Median 5x5 Noisy input Bilateral filter 7x7 window Tone Mapping Tone Mapping [Duran d 02] [Duran d 02] HDR input output Photographic Style Transfer Photographic Style Transfer [Bae 06] [Bae 06] input output Cartoon Rendition Cartoon Rendition [Winnemöller 06] [Winnemöller 06] 6 pppapers at SIGGRAPH’07 input output Gaussian Blur input per-pixel multiplication input * output average box average Gaussian blur 1 x2 G (x) exp Equation of Gaussian Blur Gaussian Profile 2 2 2 pixel Same idea: weighted average of pixels. weight GB[I]p G ||pq|| Iq qS normalized Gaussian function 1 pixel position unrelated uncertain related uncertain unrelated 0 pixels pixels pixels pixels pixels Spatial Parameter How to set • Depends on the application. input GB[I]p G || p q || Iq qS • Common strategy: proportional to image size size of the window – e.g. 2% of the image diagonal – property: independent of image resolution small large limited smoothing strong smoothing Properties of Gaussian Blur Properties of Gaussian Blur • Weights independent of spatial location • Does smooth images input • But smoothes too much: – linear convolution edges are blurred. – Only spatial distance matters – well-known operation –No edge term – efficient computation (recursive algorithm, FFT…) output GB[I]p G || p q || Iq qS space Blur Comes from Averaging across Edges Bilateral Filter No[Aurich Averaging 95 , Smith 97, Tomasi across 98] Edges input * output input * output * * * * SGiklSame Gaussian kernel everyw here. The kerne l s hape depen ds on the image con ten t. Illustration a 1D Image Bilateral Filter Definition •1D imaggpe = line of pixels Same idea: weighted average of pixels. new not new new 1 BF [I ] G || p q || G | I I | I • Better visualized as a plot p s r p q q Wp qS normaliza tion space weihtight range weihtight pixel factor I intensity pixel position Gaussian Blur and Bilateral Filter Bilateral Filter on a Height Field Gaussian blur 1 BF [I ] G || p q || G | I I | I p s r p q q p Wp qS q GB[I]p G || p q || Iq qS space space Bilateral filter [Aurich 95, Smith 97, Tomasi 98] p r a nge 1 BF [I ] G || p q || G | I I | I p s r p q q q W qS p space range output input normalization space reproduced from [Durand 02] Space and Range Parameters Influence of Pixels Only pixels close in space and in range are considered. 1 BF [I ] G || p q || G | I I | I space p s r p q q Wp qS • space s : spatial extent of the kernel, size of range the considered neighborhood. p •range r : “minimum” amplitude of an edge Exploring the Parameter Space Varying the Range Parameter r = r = r =01= 0.1 r =025= 0.25 (Gauss ian blur ) r =01= 0.1 r =025= 0.25 (Gauss ian blur ) input input s = 2 s = 2 s = 6 s = 6 s = 18 s = 18 input r = 0.1 r = 0.25 r = (Gauss ian blur ) Varying the Space Parameter r = input r =01= 0.1 r =025= 0.25 (Gauss ian blur ) input s = 2 s = 6 s = 18 s = 2 s = 6 s = 18 How to Set the Parameters Depends on the application. For instance: • space parameter: proportional to image size – e.g., 2% of image diagonal • range parameter: proportional to edge amplitude – e.g., mean or median of image gradients • independent of resolution and exposure Iterating the Bilateral Filter input I(n1) BF[I(n) ] • Generate more piecewise-flat images • Often not needed in computational photo, but could be useful for applications such as NPR. 1 iteration 2 iterations 4 iterations Advantages of Bilateral Filter • Easy to understand – We ig hte d mean of nearby pilixels • Easy to adapt – Distance between pixel values • Easy to set up – Non-iterative Hard to Compute But Bilateral Filter is Nonlinear 1 • Nonlinear BF [I ] G || p q || G | I I | I p s r p q q Wp qS • Slow but some accelerations exist: • Complex, spatially varying kernels – [Elad 02]: Gauss-Seidel iterations – Cannot be precomputed, no FFT… • Only for many iterations – [Durand 02, Weiss 06]: fast approximation • No formal understanding of accuracy versus speed • [Weiss 06]: Only box function as spatial kernel • Brute-force implementation is slow > 10min Definition of Bilateral Filter A Fast Approximation • [Smith 97, Tomasi 98] ItInput RltResult of the Bila tera l Filter • Smoothes an image and preserves edges using a Signal Processing • Weighted average Approach of neighbors •Weights Sylvain Paris and Frédo Durand – Gaussian on space distance Computer Science and Artificial Intelligence Laboratory – Gaussian on range distance Massachusetts Institute of Technology –sum to 1 space range Contributions Intuition on 1D Signal • Lin k with linear filtering • Fast and accurate approximation BF Basic idea Basic idea 1D Gaussians 1D Gaussians 1 1 BF [I ] Gq;p, G I ;I , I BF [I ] Gq;p, GI ;I , I p W s q p r q p W s q p r q p qS p qS 2D Gaussians 1 BF [I ] Gq, I ;p, I , , I p W q p s r q,I 'q p q,I 'q S ' a special 2D image Intuition on 1D Signal Intuition on 1D Signal Weighted Average of Neighbors weights p applied to pixels BF • Near and similar pixels have influence. • Far pixels have no influence. • Pixels with different value have no influence. Link with Linear Filtering 1. Handling the Division Formalization: Handling the Division p • Normalizing factor as homogeneous coordinate sum of weights • Multiply both sides by Handling the division with a projective space. Link with Linear Filtering Formalization: Handling the Division space: 1D Gaussian 2. Introducing a Convolution range: 1D Gaussian combination: 2D Gaussian p with Wq=1 q • Similar to homogeneous coordinates in projective space • Division delayed until the end • Next step: Adding a dimension to make a convolution appear space range Link with Linear Filtering space: 1D Gaussian Link with Linear Filtering 2. Introducing a Convolution range: 1D Gaussian 2. Introducing a Convolution combination: 2D Gaussian p q sum all values black = zero space-range GiGaussian space x range Corresponds to a 3D Gaussian on a 2D image. sum all values multiplied by kernel convolution Link with Linear Filtering Link with Linear Filtering 2. Introducing a Convolution 2. Introducing a Convolution result of the convolution result of the convolution space-range GiGaussian space-range GiGaussian Reformulation: Summary higher dimensional functions wiw i w Gaussian convolution 1. Convolution in higher dimension • expensive but well understood (linear, FFT, etc) 2. Division and slicing division • nonlinear but simple and pixel-wise slicing Exact reformulation higher dimensional functions higher dimensional functions wiw i w wiw i w D O W N S A M P L E Low-pass filter Gaussian convolution Gaussian convolution U P S A M P L E division division slicing slicing Fast Convolution by Downsampling Accuracy versus Running Time • Finer sampling increases accuracy. • Downsampling cuts frequencies •More ppprecise than previous work. above Nyquist limit – Less data to process PSNR as function of Running Time – But induces error Digital photograph 1200 1600 • Evaluation of the approximation Straightforward – Precision versus running time implementation is – Visual accuracy over 10 minutes. Visual Results Conclusions • Comparison with previous work [Durand 02] – running time = 1s for both techniques hig her dimens ion “be tter ” computa tion 1200 1600 itinput exact BF our result prev. work Practical gain Theoretical gain • Interactive running time • Link with linear filters • Visually similar results • Separation linear/nonlinear • Simple to code (100 lines) • Signal processing framework difference 0.1 with exact computation (intensities in [0:1]) 0 Ansel Adams Two-scale Tone Management for Photoggpraphic Look Soonmin Bae, Sylvain Paris, and Frédo Durand MIT CSAIL Ansel Adams, Clearing Winter Storm An Amateur Photographer A Variety of Looks Goals Aspects of Photographic Look • Control over photographic look • Subject choice • Transfer “look” from a model photo •Framing and composition Specified by input photos For example , Input • Tone distribution and contrast we want Modified based on model photos with the look of Model Tonal Aspects of Look Tonal aspects of Look - Global Contrast Ansel Adams Kenro Izu Ansel Adams Kenro Izu High Global Contrast Low Global Contrast Tonal aspects of Look - Local Contrast Overview Model Input Image Result Ansel Adams Kenro Izu • Transfer look between photographs Variable amount of texture Texture everywhere – Tonal aspects Overview Global contrast Overview Global contrast Split Careful combination Input Input PtPost- Result process Image Image Local contrast • Separate glbllobal an dld loca l contrast Local contrast Result Overview Global contrast Split Global vs. Local Contrast • Naïve decomppgqyosition: low vs. high frequency – Problem: introduce blur & halos Split Careful combination Halo Input PtPost- process Image Blur Local contrast Low frequency High frequency Result Global contrast Local contrast Bilateral Filter Bilateral Filter •Edge-ppgreserving smoothin g[]g [Tomasi 98] •Edge-ppgreserving
Details
-
File Typepdf
-
Upload Time-
-
Content LanguagesEnglish
-
Upload UserAnonymous/Not logged-in
-
File Pages38 Page
-
File Size-