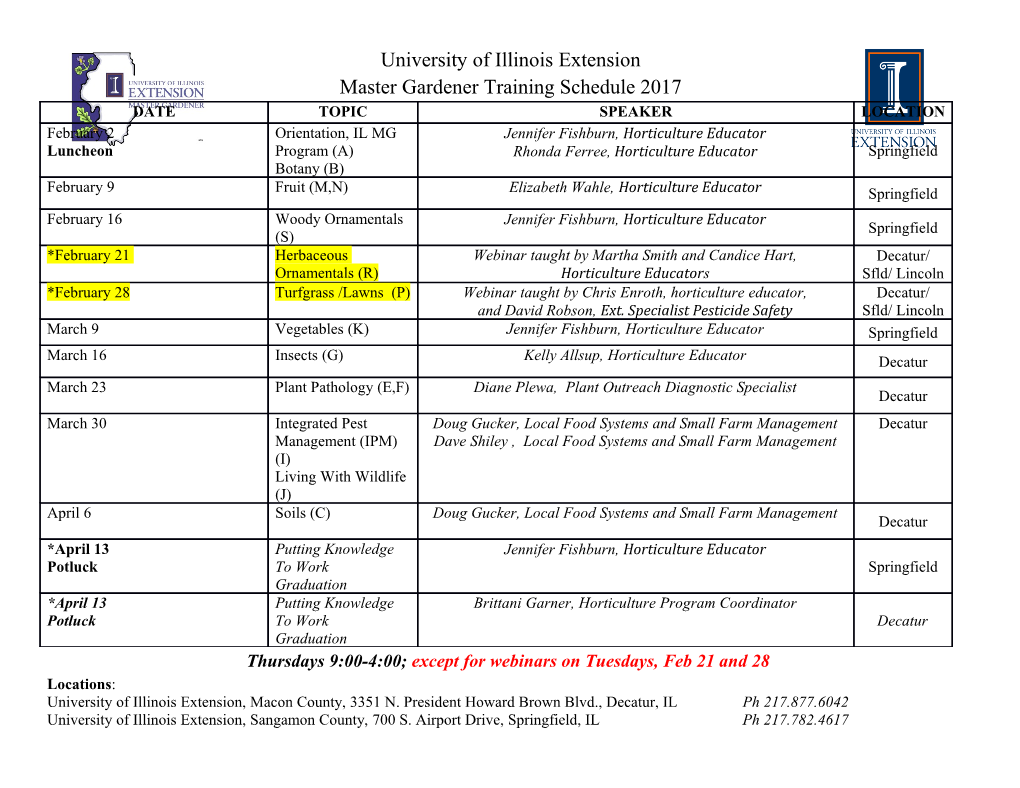
Rice Science, 2005, 12(3): 207-212 207 http://www.ricescience.org Geostatistical Analysis on the Temporal Patterns of the Yellow Rice Borer, Tryporyza incertulas 1 2 1 YUAN Zhe-ming , WANG Zhi , HU Xiang-yue (1Bio-safety Science and Technology College, Hunan Agricultural University, Changsha 410128, China; 2Department of Life Science, Hunan Arts and Science University, Changde 415000, China) Abstract: In order to comprehend temporal pattern of the larvae population of the yellow rice borer, Tryporyza incertulas, and provide valuable information for its forecast model, the data series of the population for each generation and the over-wintered larvae from 1960 to 1990 in Dingcheng District, Changde City, Hunan Province, were analyzed with geostatistics. The data series of total number, the 1st generation, the 3rd generation and the over-wintered larvae year to year displayed rather better autocorrelation and prediction. The data series of generation to generation, the 2nd generation and the 4th generation year to year, however, demonstrated poor autocorrelation, especially for the 4th generation, whose autocorrelation degree was zero. The population dynamics of the yellow rice borer was obviously intermittent. A remarkable cycle of four generations, one year, was observed in the population of generation to generation. Omitting the certain generation or interposing the over-wintered larvae only resulted in a less or slight change of autocorrelation of the whole data series generation to generation. Crop system, food, climate and natural enemies, therefore, played more important roles in regulating the population dynamics than base number of the larvae. The basic techniques of geostatistics applied in analyzing temporal population dynamics were outlined. Key words: Tryporyza incertulas; geostatistics; temporal patterns; population; forecast Generally, population dynamics is durative. successive observations were obtained at different Based on the relativities among successive time intervals. Therefore, these data should be observations obtained at uniform time intervals, time processed with mathematic interpolation before using series analysis seeks after the rule of population for further analysis. Krigle estimates, which uses the dynamic and hereby carries through forecast [1]. semivariogram in calculating estimates of the surface Geostatistics is a branch of applied statistics at the grid nodes, is considered to be the optimum developed by George Matheron. A unique aspect of method among several mathematic interpolation geostatistics is the use of regionalized variables which methods because of its minimum variance and linear are variables that fall between random variables and unbiased estimates[9]. Secondly, auto-regression (AR) completely deterministic variables. As one of the most model in time series analysis usually presumes that important elements of regionalized variable theory, the current observation yt is the linear combination of semivariance expresses degree of spatial dependence itself last p observations, yt-1, yt-2,…, yt-i, …, and between samples. Geostatistics has been applied to yt-p (i=1, 2, …, p). The upper limit of p value can be spatial patterns analysis of insect populations widely. conducted from the aftereffect time range given by However, it seldom involved in the temporal patterns semivariance model and the weight of yt-i can be analysis [2–9]. Its potential applications in temporal given by Kriging[1, 9]. So, geostatistics can be used for patterns analysis can be summarized as follows at AR modeling. Thirdly, geostatistics can determine least. Firstly, the systemic data for forecasting should whether the time series data is stochastic or be intact and sampled at uniform time intervals. The deterministic, and if the data is deterministic, how certain year or generation data, unfortunately, were strong the autocorrelation degree is [10]? Obviously, omitted in some systemic data. Similarly, some not all successive observations are fit for time series analysis and forecast, if and only if the time series Received: 22 June 2005; Accepted: 28 August 2005 data display considerable autocorrelation forecast Corresponding author: YUAN Zhe-ming ([email protected]) values given by AR model based on Kriging are 208 Rice Science, Vol. 12, No. 3, 2005 reasonable. Thus, geostatistics can make the points minus time lag. suggestion that whether the year to year data or the Semivariance curve usually can be simulated by generation to generation data should be adopted for spherical model, exponential model or linear model. AR modeling. Fourthly, geostatistics can determine To spherical model A: whether the time series data is seasonal or not? If yes, how long is the cycle? [9] And the last, geostatistics can help to comprehend the effect of every generation Where c0 is nuggets, c is sills, a is aftereffect larvae and the over-wintered larvae on the time range. In practice, a better simulation usually can autocorrelation degree of the whole data series from be resulted from spherical model B: generation to generation by the omitting or interposing 2 3 r(h)=c0+c1h+c2h +c3h . analysis. In this study, semivariances were calculated with Temporal pattern is essentially different from a Visual Basic procedure programmed by ourselves spatial pattern for its one-dimension and uniaxial and the parameters of spherical model B were given orientation. Therefore, geostatistics need to be by Data Process System (DPS) with Marquart method. developed in theory and technique while it is used for Based on the given parameters, the partial maximum temporal patterns analysis. Furthermore, temporal value c of r(h) and its corresponding h (i.e. aftereffect pattern is more flexible in dividing up time series data, time range a) were also calculated by another Visual and both natural interval (e.g. year to year) and Basic program [3–6]. physiological & ecological interval (e.g. generation to Typical time series data usually contains generation) are permissible. The results of temporal long-term trend, periodicity and random element. The patterns analysis of the yellow rice borer, Tryporyza former two characteristics are just the deterministic incertulas, were reported and the geostatistics element of geostatistics model. The semivargram methods for temporal patterns analysis were displays a beeline with positive slope when the data established in the current paper. contains long-term trend mainly, or a seasonal fluctuation when the data contains periodicity mainly, MATERIALS AND METHODS especially for the h exceeding aftereffect time range [9]. Only 31 years data were involved in this research and Data resources the upper limit of h should be the half-length of time T. incertulas occurs four generations every year series, 15 years. Based on the period analysis in Dingcheng District, Changde, Hunan Province. The computed by DPS, their cycles of these data from year peak values of each generation larvae and to year were all beyond 8 years and no semivargram over-wintered larvae from 1960 to 1990 in Dingcheng displayed upwards of two intact cycles in the research. district were provided by Station of Plant Protection Therefore, only the data from generation to generation [1] and Quarantine of Hunan Province. was carried through period analysis in the research . Geostatistics analysis Krigle interpolation Suppose Z(X ) is the value to be estimated. There Considering regular time series data interval (h) 0 are n known points X (i=1, 2, … , n), which apart, the semivariance r(h) can be estimated for i corresponding observation value is Z(X ), in the intervals that are multiple of (h): i neighborhood of X0 within the range. Li is the weight of Xi. Then, Z(X0)=∑Li(Xi)(i=1, 2, 3, …, n), Where Z(Xi) is the measurement of a regionalized To make the expectation variance minimum in X0 variable taken in time Xi, Z(Xi+h) is another and to be satisfied with ∑Li=1, Li can be calculated measurement taken h intervals away, and N(h) is the by semivariance model. The interpolation interval was number of separating time, expresses as number of half generation in the research [8]. YUAN Zhe-ming, et al. Geostatistical Analysis on Temporal Patterns of Yellow Rice Borer, Tryporyza incertulas 209 16.1%. Here would rather explore for research RESULTS methods than interpolate for application. Semivariance analysis for total larvae, every Semivariance analysis and Krigle interpolation for generation larvae and the over-wintered larvae T. incertulas larvae population from generation to among years generation Total larvae, the sum of every generation except The peak values of every generation larvae of the over-wintered larvae, express the rampancy degree T. incertulas (total 124 generations) from 1960 to all-year. According to Table 1 and Fig. 2, the total 1990 in Dingcheng district, their semivariagram curve larvae, the 1st generation, the 3rd generation and the and the parameters for semivariance model were over-wintered larvae displayed rather better demonstrated or listed in Fig. 1, Fig. 2 and Table 1, autocorrelation. The ratios of deterministic elements respectively. The series data from generation to generation presented more stochastic than to total variances were 57.5%, 60.0%, 77.1% and deterministic and the ratios of stochastic elements to 75.0% respectively, and the aftereffect time range total variances were 83.9% and 16.1%, respectively. were 4.7, 2.9, 5.9 and 4.1 years respectively. Taken the Its aftereffect time range was 16.7 generations. Taken analysis
Details
-
File Typepdf
-
Upload Time-
-
Content LanguagesEnglish
-
Upload UserAnonymous/Not logged-in
-
File Pages6 Page
-
File Size-