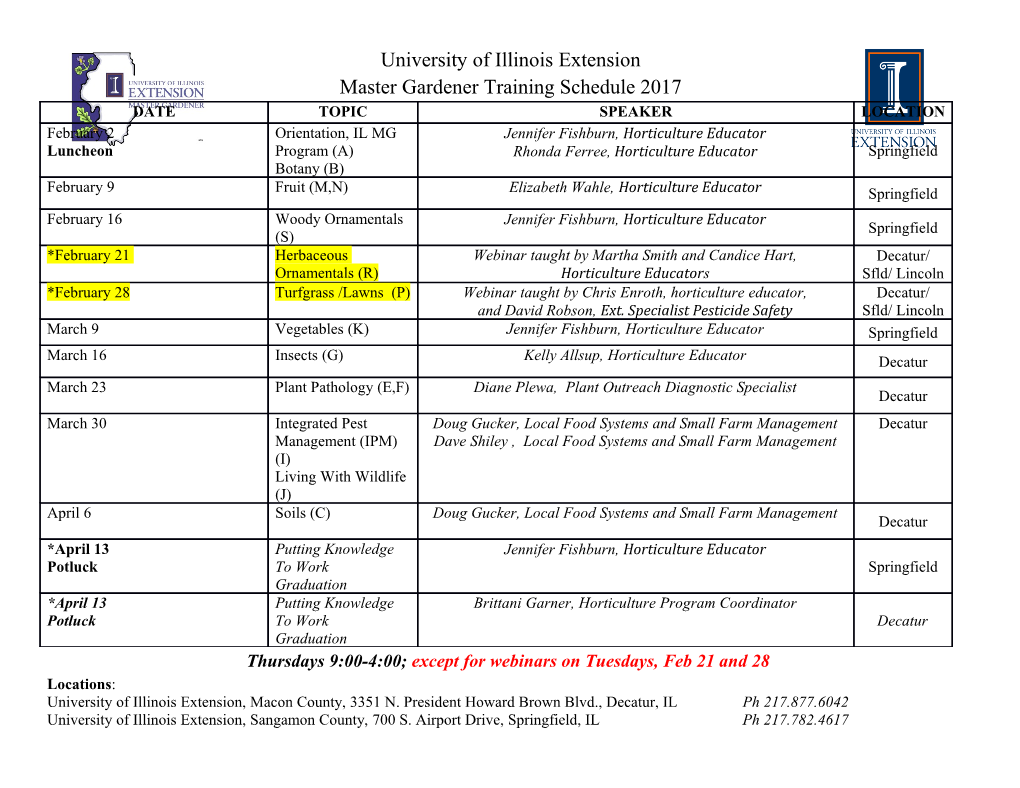
EVALUATING ALTERNATIVE STRATEGIES FOR STRATIFICATION FACTORS IN RANDOMIZED CONTROLLED TRIAL ANALYSIS by Stuart Alan Gansky Department of Biostatistics University of North Carolina Institute of Statistics Mimeo Series No. 2168T August 1996 EVALUATING ALTERNATIVE STRATEGIES FOR STRATIFICATION FACTORS IN RANDOMIZED CONTROLLED TRIAL ANALYSIS by Stuart Alan Gansky A Paper submitted to the faculty of the University ofNorth Carolina at Chapel Hill in partial fulfillment ofthe requirements for the degree ofDoctor ofPublic Health in the Department ofBiostatistics Chapel Hill 1996 Approved by: Advisor Reader ----:::::r~c:....::....::.=.o....-=+---.::..::..-=s._ +-=~~::::::::::::~~~ Reader 111 EVALUATING ALTERNATIVE STRATEGIES FOR STRATIFICATION FACTORS IN RANDOMIZED CONTROLLED TRIAL ANALYSIS Stuart Alan Gansky (under the direction ofGary Grove Koch) ABSTRACT In randomized controlled trials, traditionally there are a set of strata and within each stratum patients are randomized to a treatment condition. Stratified designs are often used to address concerns about study generalizability to traditionally underrepresented patient subgroups. For many studies, the stratification applied during design is much more extensive than can be fully incorporated in analyses. One potential strategy for such situations is to ignore one or more stratification factors; another is to account for one or more factors with nonparametric covariance adjustment. Consequences ofignoring strata or adjusting strata as covariates, relative to fully stratified procedures are not completely known and deserve further investigation, since trialists frequently perform analyses not fully accounting for stratification. This research evaluates properties of strategies that ignore strata or adjust strata as covariates using both design- and model-based methods, relative to fully stratified analyses, in tenns ofvalidity and power. Derivations, simulations and actual examples illustrate the perfonnance in various scenarios. Sparse strata situa- tions are examined; particular emphasis is on multicenter trials with not all treatments assigned within all centers (i.e. incomplete centers). The situation concerning many small centers (one or two patients) is explored in depth with modelling and minim,al ~ssumption methods. Using a crossover trial paradigm, estimators are developed with a priori weights to incorporate incomplete center data into the usual complete data estimator. Various patient accrual mechanisms are considered, including one involving selection bias. Power and bias are assessed for methods combining data from complete and incomplete IV centers. Finally, these methods are extended to multicenter trials with more than two patients per center; bias is assessed for the extensions as well. v ACKNOWLEDGMENTS I cannot thank Dr. Gary Koch enough for his guidance, support, and motivation. His students appreciate his patience, persistence, dedication, expertise and advice more than he can imagine. Moreover, I would like to thank Drs. Kant Bangdiwala, Harry Guess, Ronnie Homer, and Dana Quade not only for their constructive comments, suggestions, and insights concerning this dissertation, but also for their instruction and guidance in coursework and projects. In addition, I am very thankful to Amy Cutrell for kindly providing the herpes zoster data. Moreover, I sincerely appreciate the financial support from the United States National Institute of Environmental Health Science Traineeship, Veterans Affairs Office of Academic Affairs Health Services Research Predoctoral Fellowship, and UNC Biometric Consulting Laboratory Graduate Research Assistantship which have generously funded my graduate education and research. Also, Drs. Jerome Wilson, Jane Weintraub, and Joanne Harrell have presented me the opportunities to work on interesting, relevant public health problems, for which I am grateful. My colleagues and friends in the Biometric Consulting Laboratory, formerly "The Trailer", have helped me a great deal over the years in a challenging, yet relaxed learning atmosphere. Dr. Craig Turnbull deserves much credit for introducing me to biostatistics. Thanks also go to Mark Pinzur who showed me that "numbers are your friends" and to Dr. Ed Dwyer who taught me that you can never edit too much. I am extremely thankful to my parents Rose and Jack Gansky, who instilled in me a penchant for learning and who encouraged me by saying I could do anything ifI set my mind to it. Finally, my most sincere thanks go to my loving and supportive wife Karen Leder Gansky for her continual help and motivation, as well as her boundless understanding and patience. VI TABLE OF CONTENTS Page LIST OF TABLES x LIST OF FIGURES .xi LIST OF ABBREVIATIONS xii Chapter 1. Introduction 1 1.1. General Issues ' .1 1.2. Stratified Randomization 3 1.3. Restricted Randomization 5 1.3.1. Permuted-Block Randomization 6 1.3.2. MinimizationlDynamic Allocation 7 1.3.3. Adaptive Randomization 9 1.4. Usual Analysis Approaches 10 1.4. 1. Ignoring Stratification Factor(s) .1 0 1.4.2. Covariance Adjusting Stratification Factor(s) .11 1.5. Examples 12 1.5.1. Example 1: Analgesics and Pain Relief 12 1.5.2. Example 2: Respiratory Ailment.. .13 1.5.3. Example 3: Multicenter Herpes Zoster Study 13 1.5.4. Conceptual Examples .13 1.6. Purpose 14 2. A Review ofSome Statistical Methods for Randomized Controlled Trial Analyses 16 2.1. Introduction .16 2.2. Design-based Methods 17 2.2.1. Fisher's Exact Test. .17 w 2.2.2. Extended Mantel-Haenszel Test ~ .18 2.2.3. Stratified Randomization Analysis ofCovariance 23 2.3. Model-based Methods .24 2.3.1. Logistic Regression 24 2.3.2. Ordinal Logistic Regression .25 2.3.3. Two Sample t-Test. 26 2.3.4. Paired t-Test 26 2.3.5. General Linear Univariate Model.. 27 2.3.6. Random/Mixed Effects Model. 29 2.3.7. Weighted Least Squares 31 2.3.8. Generalized Estimating Equations 33 2.3.9. Survey Data Regression 35 3. Unstratified Analyses for Stratified Designs .37 3.1. Introduction .37 3.2. Assessments with Example RCTs .37 3.2.1. Example 1: Dental Pain Trial.. 37 3.2.2. Example 2: Respiratory Ailment Study .40 3.3. Simulations -. :42 3.3 .1. Normally Distributed Response :43 3.3.2. Non-Normally Distributed Response ..45 3.3.3. Simulation Results 49 3.4. Summary and Conclusions 53 4. A Random Effects Model for Incomplete Pairs in Multicenter Trials 54 4.1. Introduction 54 4.2. General model. 57 4.3. Random effects model. 61 Vlll 4.4. Example: Multicenter Herpes Zoster Study 75 4.5. Summary and Conclusions 80 5. Finite Population Framework for Incomplete Pairs in Multicenter Trials 81 5.1. Introduction 81 5.2. Fixed Patient Accrual and Random Treatment Allocation 85 5.3. Random Accrual Independent ofTreatment Allocation 92 5.4. Accrual Dependent on Allocation: Selection Bias 97 5.4.1. General Selection Bias 98 5.4.2. Selection Bias in Treatment Sequences .105 5.4.3. Notes on Selection Bias 109 5.5. Summary and Conclusions 110 6. A Random Effects Model for Incomplete Blocks in Multicenter Trials .112 6.1. Introduction .112 6.2. General ModeL 114 6.3. Random Effects Model... 115 6.3.1. Three Patient Maximum per Center. .115 6.3.2. Four Patient Maximum per Center 123 6.4. Summary and Conclusions 129 7. Finite Population Framework for Incomplete Blocks in Multicenter Trials 130 7.1. Introduction .130 7.2. Fixed Patient Accrual and Random Treatment Allocation .!33 7.3. Random Accrual Independent ofTreatment Allocation .139 7.4. Notes on Combining Centers .143 7.5. Summary and Conclusions 144 8. Summary and Future Research 146 8.1. Summary .146 IX 8.2. Recommendations 148 8.3. Future Research 148 8.3.1. Unequal Treatment Allocation in Multicenter Trials 148 8.3.2. Stratified Incomplete Multicenter Extensions 149 8.3.3. Alternative Multicenter Designs .l50 8.3.4. Other Extensions 151 REFERENCES .153 x LIST OF TABLES Table Page 3.1 P-values for testing each active treatment group versus placebo for summary measures and hourly dental pain reliefwith centers as strata 38 • 3.2 P-values for testing each active treatment group versus placebo for summary measures and hourly dental pain reliefwith centers x permuted blocks as strata 39 3.3 P-values for testing treatment differences for ordinal respiratory measures with centers as strata A1 3.4 Comparison ofnormal and non-normal distributions 47 3.5 Distributional parameter values and measures ofcentral tendency and dispersion .48 3.6 Empirical bias estimates based on 2100 simulations: Rejection rate (standard error).. 50 3.7 Empirical power estimates based on 1000 normal simulations: Rejection rate (standard error) 52 4.1 WLS results for 7 day standard and test treatments 17 4.2 WLS results for 7 and 14 day test treatments 78 5.1 Assumptions needed for unbiasedness with fixed accruaL 88 5.2 Unbiasedness assumptions with random accrual independent ofallocation 94 5.3 Large sample unbiasedness with random accrual dependent on allocation (general selection bias) 101 5.4 Unbiasedness with random accrual dependent on allocation (treatment allocation selection bias) .107 7.1 Assumptions needed for unbiased treatment difference with fixed accruaL 137 7.2 Assumptions for unbiased treatment difference with random accrual.. .l41 Xl LIST OF FIGURES Figure Page 2.1 Cross-tabulation ofthe hth stratum 19 3.1 Nonnal distribution simulations (2 treatments and 2 strata) A4 • 3.2 Non-nonnal distribution simulations (2 treatments and 2 strata) A6 4.1 Multicenter data structure : 62 4.2 Variance as a function ofratio ofsingles to pairs (fap), intraclass correlation (PI), and weights
Details
-
File Typepdf
-
Upload Time-
-
Content LanguagesEnglish
-
Upload UserAnonymous/Not logged-in
-
File Pages170 Page
-
File Size-