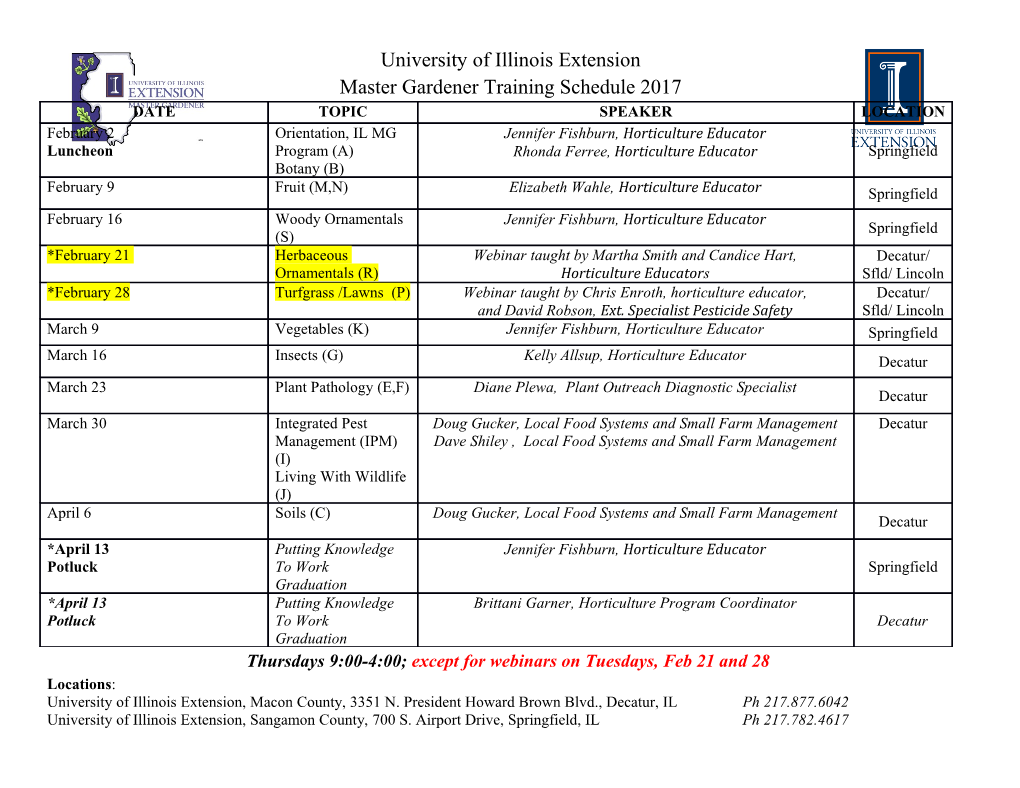
Module 4: Point Estimation Statistics (OA3102) Professor Ron Fricker Naval Postgraduate School Monterey, California Reading assignment: WM&S chapter 8.1-8.4 Revision: 1-12 1 Goals for this Module • Define and distinguish between point estimates vs. point estimators • Discuss characteristics of good point estimates – Unbiasedness and minimum variance – Mean square error – Consistency, efficiency, robustness • Quantify and calculate the precision of an estimator via the standard error – Discuss the Bootstrap as a way to empirically estimate standard errors Revision: 1-12 2 Welcome to Statistical Inference! • Problem: – We have a simple random sample of data – We want to use it to estimate a population quantity (usually a parameter of a distribution) – In point estimation, the estimate is a number • Issue: Often lots of possible estimates – E.g., estimate E(X) with x , x , or ??? • This module: What’s a “good” point estimate? – Module 5: Interval estimators – Module 6: Methods for finding good estimators Revision: 1-12 3 Point Estimation • A point estimate of a parameter q is a single number that is a sensible value for q – I.e., it’s a numerical estimate of q – We’ll use q to represent a generic parameter – it could be m, s, p, etc. • The point estimate is a statistic calculated from a sample of data x – The statistic is called a point estimator – Using “hat” notation, we will denote it as qˆ – For example, we might use to estimate m, so in this case mˆ x Revision: 1-12 4 Definition: Estimator An estimator is a rule, often expressed as a formula, that tells how to calculate the value of an estimate based on the measurements contained in a sample Revision: 1-12 5 An Example • You’re testing a new missile and want to estimate the probability of kill (against a particular target under specific conditions) – You do a test with n=25 shots – The parameter to be estimated is pk, the fraction of kills out of the 25 shots • Let X be the number of kills – In your test you observed x=15 • A reasonable estimator and estimate is X x 15 estimator: pˆ estimate: pˆ 0.6 k n k n 25 Revision: 1-12 6 A More Difficult Example • On another test, you’re estimating the mean time to failure (MTTF) of a piece of electronic equipment • Measurements for n=20 tests (in units of 1,000 hrs): • Turns out a normal distribution fits the data quite well • So, what we want to do is to estimate m, the MTTF • How best to do this? Revision: 1-12 7 Example, cont’d • Here are some possible estimators for m and their values for this data set: 1 20 ˆ (1) m X, so x xi 27.793 20 i1 27.94 27.98 (2) mˆ Xx, so 27.960 2 min(XX ) max( ) 24.46 30.88 (3) mˆ Xx ii, so 27.670 ee22 1 18 ˆ (4) m Xtr(10), so x tr (10) x ( i ) 27.838 16 i3 • Which estimator should you use? – I.e., which is likely to give estimates closer to the true (but unknown) population value? Revision: 1-12 8 Another Example • In a wargame computer simulation, you want to 2 estimate a scenario’s run-time variability (s ) • The run times (in seconds) for eight runs are: • Two possible estimates: n 1 2 ˆ 2 2 2 (1) s S Xi X , so s 0.25125 n 1 i1 n 1 2 ˆ 2 (2) s XXi , so estimate 0.220 n i1 • Why prefer (1) over (2)? Revision: 1-12 9 Bias • Definition: Let q ˆ be a point estimator for a q – is an unbiased estimator if E qq ˆ – If E q ˆˆ q , q is said to be biased • Definition: The bias of a point estimator is given by BEqˆˆ q q • E.g.: Revision: 1-12 10 * Figures from Probability and Statistics for Engineering and the Sciences, 7th ed., Duxbury Press, 2008. Proving Unbiasedness • Proposition: Let X be a binomial r.v. with parameters n and p. The sample proportion p ˆ X n is an unbiased estimator of p. • Proof: Revision: 1-12 11 Remember: Rules for Linear Combinations of Random Variables • For random variables X1, X2,…, Xn – Whether or not the Xis are independent E a1 X 1 a 2 X 2 ann X a1 E X 1 a 2 E X 2 ann E X – If the X1, X2,…, Xn are independent Var a1 X 1 a 2 X 2 ann X 2 2 2 a1Var X 1 a 2 Var X 2 ann Var X Revision: 1-12 12 Example 8.1 • Let Y1, Y2,…, Yn be a random sample with 2 E(Yi)=m and Var(Yi)=s . Show that n 2 1 2 SYY' i n i1 is a biased estimator for s2, while S2 is an unbiased estimator of s2. • Solution: Revision: 1-12 13 Example 8.1 (continued) Revision: 1-12 14 Example 8.1 (continued) Revision: 1-12 15 Another Biased Estimator • Let X be the reaction time to a stimulus with X~U[0,q], where we want to estimate q based on a random sample X1, X2,…, Xn • Since q is the largest possible reaction time, ˆ consider the estimator q1 max XXX 1 , 2 , , n • However, unbiasedness implies that we can observe values bigger and smaller than q • Why? ˆ • Thus, q 1 must be a biased estimator Revision: 1-12 16 Fixing the Biased Estimator • For the same problem consider the estimator ˆ n 1 q2 max XXX 1 , 2 , , n n • Show this estimator is unbiased Revision: 1-12 17 Revision: 1-12 18 One Criterion for Choosing Among Estimators • Principle of minimum variance unbiased estimation: Among all estimators of q that are unbiased, choose the one that has the minimum variance – The resulting estimator q ˆ is called the minimum variance unbiased estimator (MVUE) of q ˆˆ Estimator qq12 is preferred to Revision: 1-12 19 * Figure from Probability and Statistics for Engineering and the Sciences, 7th ed., Duxbury Press, 2008. Example of an MVUE • Let X1, X2,…, Xn be a random sample from a normal distribution with parameters m and s. Then the estimator m ˆ X is the MVUE for m – Proof beyond the scope of the class • Note this only applies to the normal distribution – When estimating the population mean E(X)=m for other distributions, X may not be the appropriate estimator – E.g., for Cauchy distribution E(X)=! Revision: 1-12 20 How Variable is My Point Estimate? The Standard Error • The precision of a point estimate is given by its standard error • The standard error of an estimator is its standard deviation sq Var ˆ ˆ qˆ q • If the standard error itself involves unknown parameters whose values are estimated, substitution s of these estimates into q ˆ yields the estimated standard error – The estimated standard error is denoted by s ˆ or s qˆ qˆ Revision: 1-12 21 Deriving Some Standard Errors (1) • Proposition: If Y1, Y2,…, Yn are distributed iid with variance s2 then, for a sample of size n, Var Yn s 2 . Thus ss Y n . • Proof: Revision: 1-12 22 Deriving Some Standard Errors (2) • Proposition: If Yi~Bin(n,p), i=1,…,n, then s pˆ pq n , where q=1-p and pˆ Y n . • Proof: Revision: 1-12 23 Expected Values and Standard Errors of Some Common Point Estimators Target Point Standard Parameter Sample Estimator ˆ Error ˆ E q s q Size(s) q qˆ s m n m Y n Y p n pˆ p pq n n ss22 m -m n and n YY m -m 12 1 2 1 2 12 1 2 nn 12 p1 q 1 p 2 q 2 independent p1-p2 n1 and n2 ppˆˆ12 p1-p2 If populations are populations If nn 12 Revision: 1-12 24 However, Unbiased Estimators Aren’t Always to be Preferred • Sometimes an estimator with a small bias can be preferred to an unbiased estimator • Example: • More detailed discussion beyond scope of course – just know unbiasedness isn’t necessarily required for a good estimator Revision: 1-12 25 Mean Square Error • Definition: Theq ˆmean square error (MSE) of a point estimator is 2 MSEqˆˆ E q q • MSE of an estimator is a function of both its variance and its bias – I.e., it can be shown (extra credit problem) that 2 2 MSEqˆ E q ˆ q Var q ˆ B q ˆ – So, for unbiased estimators MSE qqˆˆ Var Revision: 1-12 26 Error of Estimation • Definition: Theq ˆerror of estimation e is the distance between an estimator and its target parameter: e qˆ q – Since is a random variable, so it the error of estimation, e – But we can bound the error: Pr qˆˆ q b Pr b q q b Pr q bb qˆ q Revision: 1-12 27 Bounding the Error of Estimation • Tchebysheff’s Theorem. Let Y be a random variable with finite mean m and variance s2. Then for any k > 0, Pr Yms k 1 1 k 2 – Note that this holds for any distribution – It is a (generally conservative) bound – E.g., for any distribution we’re guaranteed that the probability Y is within 2 standard deviations of the mean is at least 0.75 • So, for unbiased estimators, a good bound to use on the error of estimation is b 2s qˆ Revision: 1-12 28 Example 8.2 • In a sample of n=1,000 randomly selected voters, y=560 are in favor of candidate Jones.
Details
-
File Typepdf
-
Upload Time-
-
Content LanguagesEnglish
-
Upload UserAnonymous/Not logged-in
-
File Pages41 Page
-
File Size-