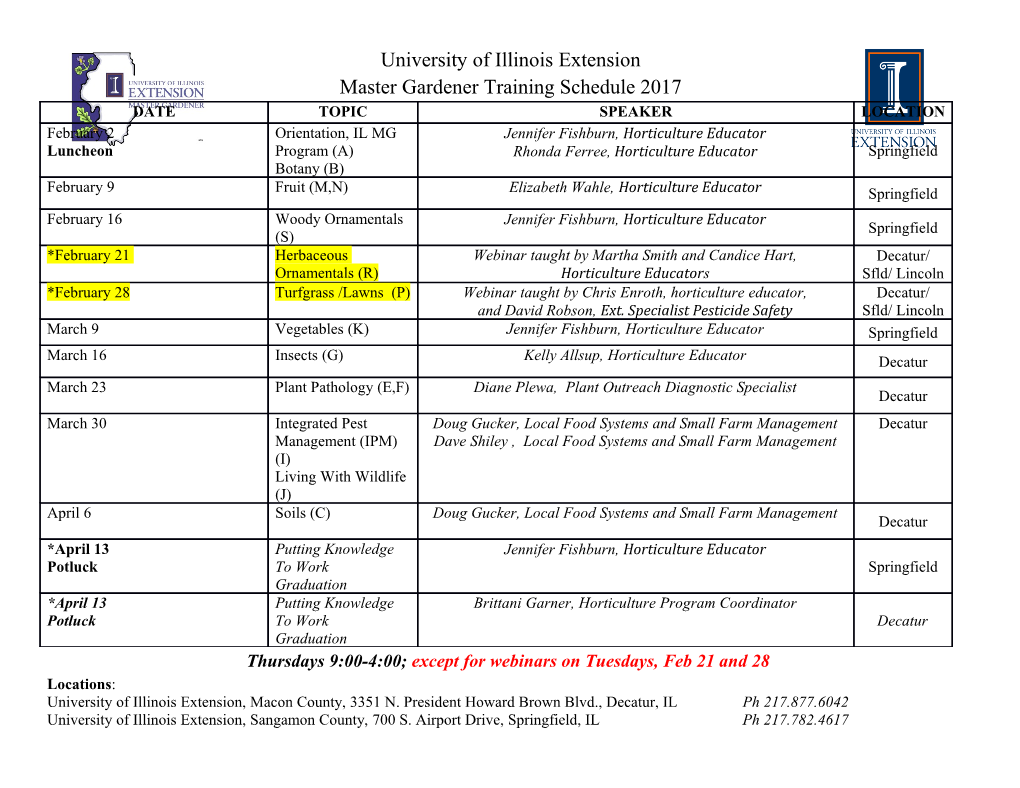
Francis Atkinson George Papadatos Chemogenomics Group EMBL-EBI High Throughput Screening • Screens involving large numbers of compounds • Hundreds of thousands to millions of samples • HTS collections designed to find hits for very diverse targets • Hit rate can be very low for any given target • Tend to be the preserve of Big Pharma Harper et al, Comb. Chem. High • Large compound collection needed Throughput Screen., 7, 63 (2004) • Significant investment in infrastructure • Sample handling, assay optimization, screening platforms, IT etc. • There are alternative strategies for lead finding… • SME and academic groups • Projects not allocated HTS or to supplement HTS 2 12/12/2013 Resources for Computational Drug Discovery Focused screening • Screen relatively few compounds Valler et al, Drug Disc. • Tens to thousands Today, 14, 286 (2000). • Compounds often bought-in specially • Selected from databases of molecules offered by vendors • Subsets of a corporate collection • Select set likely to be enriched in active compounds • Exploit knowledge from related targets (‘systems approach’) • Docking, pharmacophore searching, QSAR models etc. • Prefer those that would make good leads • Actives are only starting points for optimization 3 12/12/2013 Resources for Computational Drug Discovery Systems knowledge: Protein Kinases • Kinase inhibitors are often active at multiple target • Known actives might thus be leads for new targets • Similar compounds may be found in databases • Libraries of analogues can be synthesized Nat. Biotech. 2008, 26, 127. http://kinase.com/human/kinome/ 4 12/12/2013 Resources for Computational Drug Discovery Systems knowledge: Protein Kinases • Inhibitors often include a ‘hinge-binding’ moiety… • Substructures can be used to search databases • Libraries can be built around cores bearing motif Hajduk et al, Drug Disc. Today, 14, 291 (2009). 5 12/12/2013 Resources for Computational Drug Discovery Vendor Catalogues • Many vendors offer targeted libraries Prien, ChemBioChem, • Basic filtering often performed 6, 500 (2005) • ZINC allow searching across multiple vendor catalogues • Also performs extra filtering Irwin et al, J. Chem. Inf. Model., (2012) • Provides focused subsets DOI: 10.1021/ci3001277 6 12/12/2013 Resources for Computational Drug Discovery Filtering a compound set • Screening duplicates can waste resources • Different molecular representations in different databases • Different salt forms but same bioactive species • Lack of novelty (IP issues) • ChEMBL can help identify literature compounds • SureChEMBL for patent data • Undesirable molecular features Lipinski, Ann. Rep. Comp. • Structural Alerts Chem., 1, 155 (2005) • Compounds with developability issues • Likely to fail during lead optimisation or drug development • Representative / diverse compounds only 7 12/12/2013 Resources for Computational Drug Discovery Duplicate Removal • The same molecule can have different representations • The salt may be represented as neutral or charged • Nitro group can be charge- separated or hypervalent • The SMILES for these differ… [NH4+].[O-]C(=O)Cc1nc(O)cc(-c2cc3[nH]cnc3c([N+]([O-])=O)c2)c1 N.O=C(O)Cc1[nH]c(=O)cc(-c2cc3nc[nH]c3c([N+]([O-])=O)c2)c1 • The InChI correctly identifies these as duplicates… InChI=1S/C14H10N4O5.H3N/c19-12-4-7(1-9(17-12)5- 13(20)21)8-2-10-14(16-6-15-10)11(3-8)18(22)23;/h1- 4,6H,5H2,(H,15,16)(H,17,19)(H,20,21);1H3 http://www.inchi-trust.org/ 8 12/12/2013 Resources for Computational Drug Discovery Salts • Counter ions should not affect activity in assays • InChIs for different salts aren’t the same 1 2 InChI=1S/C14H10N4O5.H3N/c19 -12-4-7(1-9(17-12)5-13(20)21)8-2-10-14(16-6-15-10)11(3-8)18(22)23;/h1-4,6H,5H2,(H,15,16)(H,17,19)(H,20,21);1H3 1 2 InChI=1S/C14H10N4O5.C4H12N/c19-12-4-7(1-9(17-12)5-13(20)21)8-2-10-14(16-6-15-10)11(3-8)18(22)23;1-5(2,3)4/h1-4,6H,5H2,(H,15,16)(H,17,19)(H,20,21);1-4H3/q;+1/p-1 • Counter ions can be removed before InChI generation • Compare only the biologically-relevant components • This is sometimes done in databases A. Gaulton et al, Nucl. Acids Res., Database • ChEMBL contains both salt and parent forms Issue, 8 (2011). 9 12/12/2013 Resources for Computational Drug Discovery Structural Alerts • Substructures marking molecules as ‘of concern’ • Toxicophores • e.g. electrophiles implicated in DNA damage Blagg, Ann. Rep. Med. • CYP450 inhibitors and substrates Chem., 41, 353 (2006). • Particularly if metabolism generates reactive species • Compounds that interfere with assays • Promiscuous compounds • Association with poor solubility or permeability • Chemotypes that have repeatedly failed in development 10 12/12/2013 Resources for Computational Drug Discovery Example filter sets 11 12/12/2013 Resources for Computational Drug Discovery Developability • Bias efforts towards molecules more likely to succeed • Assuming activity against therapeutic target • A wide range of techniques are available • From simple property ranges to synthetic feasibility • Interpretability by non-specialists is important Ritchie et al, Drug Disc. Today, 14, 1011 (2009). Developability Increasing Decreasing 12 12/12/2013 Resources for Computational Drug Discovery Physico-chemical parameters pIC50 binding data ~200K compounds Molecular Weight Molecular Drugs Rat bioavailability ~ 3.6K compounds Gleeson et al, Nature Rev. Drug Disc., 10, 197 (2011). ALogP (a computed lipophilicity) 13 12/12/2013 Resources for Computational Drug Discovery Druglikeness: Lipinski’s Rule of Five Lipinski et al, Adv. Drug • Old, but widely known and still used Deliv. Rev., 23, 3 (1997) • Hugely important in raising awareness of issues • Based on analysis of drugs vs. candidates that failed • A compound is less likely to be well absorbed if… • The molecular weight is greater than 500 • The logP (a measure of lipophilicity) is greater than 5 • The number of hydrogen bonds acceptors is greater than 10 • The number of hydrogen bond donors is greater than 5 • Many alternative schemes have since been published Kenny et al, J. Comput. Aided. • Can be useful but not infallible Mol. Des., 27, 1 (2013) • Best used alongside other metrics in compound progression 14 12/12/2013 Resources for Computational Drug Discovery Leadlikeness • Leads are the starting points for drug discovery • Optimization increases size and complexity Oprea et al, J. Chem. Inf. Comput. Sci., 41, 1313 (2001) • Ideally, leads should be smaller and simpler than drugs • Leadlikeness scores have thus been proposed… • Inspired by RO5, but emphasizing smallness & simplicity more 15 12/12/2013 Resources for Computational Drug Discovery Fragments: Rule of Three • Start with very small ‘scaffold-like’ molecules • Detect binding via crystallography, biophysical methods or high-concentration screening Carr et al, Drug Disc. Today, 7, 522 (2002). • A fragment is more likely to be successful if… • The molecular weight is less than 300 Congreave et al, Drug Disc. Today, • The logP is less than 3 8, 876 (2003). • The number of hydrogen bonds acceptors is less than 3 • The number of hydrogen bond donors is less than 3 16 12/12/2013 Resources for Computational Drug Discovery Diversity Selection (1) • Filtering alone may not reduce set to a tractable size • Similar compounds tend to have similar activities • Screening many close analogues won’t add much information • Screen only a ‘representative’ subset of compounds • Screen analogues of actives as a follow up step • Many algorithms for subset selection available… Ashton et al, Quant. Struct.-Act. Relat., 21, 598 (2002). 17 12/12/2013 Resources for Computational Drug Discovery Diversity Selection (2) • Map compounds into a ‘chemical space’ • Defined by properties or structural features • Generally high dimensional • Form ‘clusters’ of similar compounds • Pick representative(s) from clusters • Pick compounds that best ‘span’ the space 18 12/12/2013 Resources for Computational Drug Discovery Further Reading 19 12/12/2013 Resources for Computational Drug Discovery Using Open-Source Software • These ideas can be easily implemented using OSS • Python is one popular choice • Full suite of tools for stats, machine-learning, plotting etc. • We use RDKit for chemistry • Tools such as IPython simplify development • Scripts can also be run on a cluster for higher throughput 20 12/12/2013 Resources for Computational Drug Discovery On to KNIME… • A workflow tool such as KNIME is another option • especially good for non-programmers • Various options for chemistry, including RDKit 21 12/12/2013 Resources for Computational Drug Discovery .
Details
-
File Typepdf
-
Upload Time-
-
Content LanguagesEnglish
-
Upload UserAnonymous/Not logged-in
-
File Pages21 Page
-
File Size-