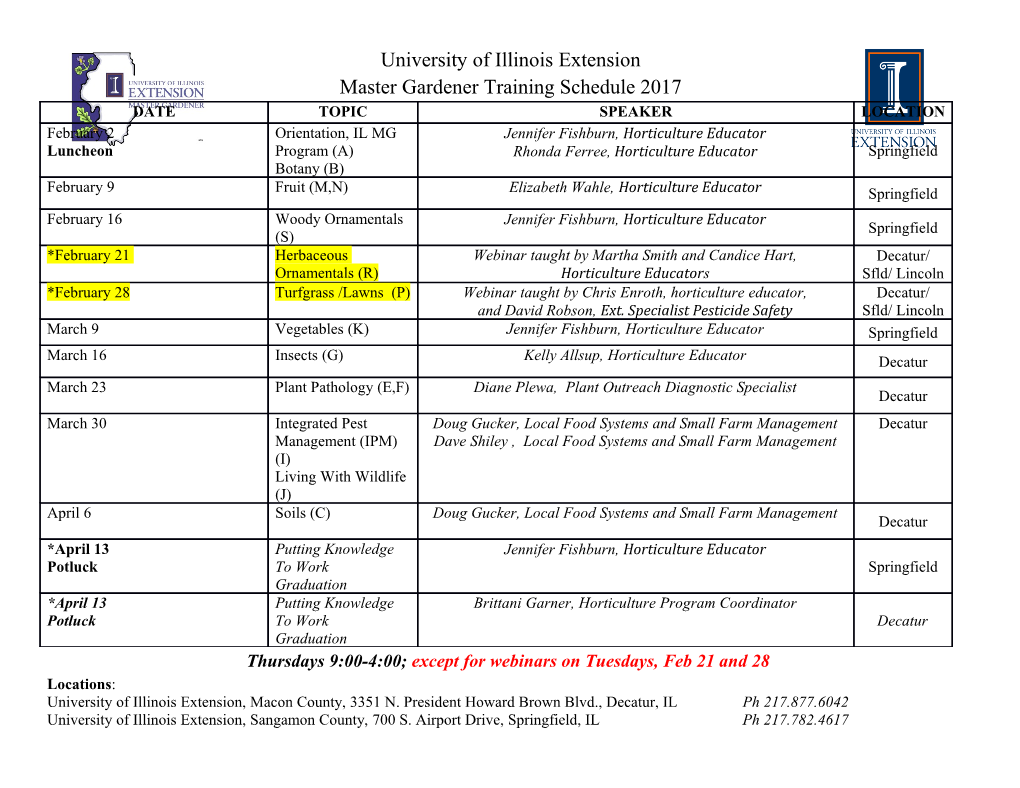
Journal of Nuclear Medicine, published on October 18, 2019 as doi:10.2967/jnumed.119.230797 Predictive Value of 18F-Florbetapir and 18F-FDG PET for Conversion from Mild Cognitive Impairment to Alzheimer Dementia Ganna Blazhenets1, Yilong Ma2, Arnd Sörensen1, Florian Schiller1, Gerta Rücker3, David Eidelberg2, Lars Frings1, 4 and Philipp T. Meyer1 for the Alzheimer Disease Neuroimaging Initiative* 1Department of Nuclear Medicine, Medical Center – University of Freiburg, Faculty of Medicine, University of Freiburg, Freiburg, Germany 2Center for Neurosciences, The Feinstein Institute for Medical Research, Manhasset, NY, USA 3Institute of Medical Biometry and Statistics, Medical Center – University of Freiburg, Faculty of Medicine, University of Freiburg, Freiburg, Germany 4Center for Geriatrics and Gerontology Freiburg, Medical Center - University of Freiburg, Faculty of Medicine, University of Freiburg, Freiburg, Germany Short running title: Florbetapir and FDG PET predict conversion to AD Word count: 4207 Corresponding Author: G. Blazhenets, Hugstetter Str. 55, 79106, Freiburg, Germany. https://orcid.org/0000-0003-2419-7373 [email protected], Tel: +0049 761 270 38861. *Data used in preparation of this article were obtained from the Alzheimer’s Disease Neuroimaging Initiative (ADNI) database (adni.loni.usc.edu). As such, the investigators within the ADNI contributed to the design and implementation of ADNI and/or provided data but did not participate in analysis or writing of this report. A complete listing of ADNI investigators can be found at: http://adni.loni.usc.edu/wp- content/uploads/how_to_apply/ADNI_Acknowledgement_List.pdf ABSTRACT 1 The present study examines the predictive values of amyloid PET, 18F-fluorodeoxyglucose 2 (FDG) PET and non-imaging predictors (alone and in combination) for development of 3 Alzheimer dementia (AD) in a large population of patients with mild cognitive impairment 4 (MCI). 5 Methods: 319 patients with MCI from the Alzheimer’s disease neuroimaging initiative database 6 were included. In a derivation dataset (n = 159), the following Cox proportional hazard models 7 were constructed, each adjusted for age and sex: i) amyloid PET using 18F-Florbetapir: pattern 8 expression score (PES) of an amyloid-beta AD conversion-related pattern (Aβ-ADCRP) 9 constructed by principle components analysis (PCA); ii) 18F-FDG PET: PES of a previously 10 defined 18F-FDG-based ADCRP, constructed by PCA; iii) non-imaging model: functional 11 activities questionnaire, apolipoprotein E and mini-mental state examination score; iv) 18F-FDG 12 PET + amyloid PET model, v) amyloid PET + non-imaging model, vi) 18F-FDG PET + non- 13 imaging model, and vii) amyloid PET + 18F-FDG PET + non-imaging model. In a second step, 14 the results of Cox regressions were applied to a validation dataset (n = 160) to stratify subjects 15 according to the predicted conversion risk. 16 Results: Based on the independent validation dataset, the 18F-FDG PET model yielded a 17 significantly higher predictive value than the amyloid PET model. However, both were inferior to 18 the non-imaging model and significantly improved by adding non-imaging variables. Best 19 prediction accuracy was reached by combining 18F-FDG PET, amyloid PET and non-imaging 20 variables. The combined model yielded five-year free-of-conversion rates of the low-, medium- 21 and high-risk groups of 100%, 64% and 24%, respectively. 2 1 Conclusions: 18F-FDG PET, amyloid PET and non-imaging variables represent complementary 2 predictors of conversion from MCI to AD. Especially in combination, they enable an accurate 3 stratification of patients according to their conversion risks which is of great interest for patient 4 care and clinical trials. 5 Keywords: Mild cognitive impairment, amyloid load, PCA, Cox model, 18F-Florbetapir, 18F- 6 FDG, PET 3 INTRODUCTION 1 Amyloid PET using 18F-Florbetapir (or 18F-AV-45) and 18F-fluorodeoxyglucose (FDG) PET are 2 established biomarkers of amyloid-beta (Aβ) pathology and neuronal injury in Alzheimer’s 3 disease, respectively (1). Both modalities have shown variable performances when adopted to 4 predict progression to Alzheimer dementia (AD) (2,3). 5 Stratifying mild cognitive impairment (MCI) subjects according to their conversion risk is 6 of great interest for clinical practice and clinical trials (e.g. patient counseling, initiation of 7 pharmacological and non-pharmacological treatments, and inclusion in trials). A recent study of 8 our group has evaluated 18F-FDG PET by voxel-wise principle components analysis (PCA) and 9 validated a PCA-derived AD conversion-related pattern (ADCRP) that showed high accuracy in 10 prediction of conversion from MCI to AD (4). This study was in contrast to other studies (5-7). 11 Difference in the results can be explained by methodology (PCA vs. conventional 18F-FDG PET 12 analysis) and/or patient populations included (highly selected research vs. real-life clinical 13 population (5)). Conversely, the aforementioned studies favor amyloid PET over 18F-FDG PET 14 for predicting conversion (5,7). 15 In the present study, we extended our previous work by also including amyloid PET and 16 explored the predictive values of 18F-FDG PET, amyloid PET and non-imaging variables, alone 17 and in combination, in their ability to stratify MCI patients according to their conversion risk. As 18 in our previous study, we took advantage of the very large patient dataset of the Alzheimer 19 disease neuroimaging initiative (ADNI) database, which enables proper validation by 20 independent derivation and validation datasets. For a fair comparison of modalities and in 21 additional to conventional volume-of-interest analyses (yielding continuous and binary measures 4 1 of amyloid load), we also applied voxel-wise PCA to the amyloid PET data to assess the Aβ- 2 based AD conversion-related pattern, termed Aβ-ADCRP. 5 MATERIAL AND METHODS Cohort 1 The present data were obtained from the ADNI database (ClinicalTrials.gov Identifier: 2 NCT00106899; for further information on the ADNI project see www.adni-info.org). The study 3 was approved by ADNI and written informed consent was obtained by ADNI from all subjects 4 and before protocol-specific procedures are carried out (see ADNI protocols). Of the 544 subjects 5 with MCI (suspected incipient Alzheimer disease with subjective and objective memory deficits) 6 used in our previous study (4) we included 319 patients in whom 18F-Florbetapir PET at baseline 7 visit was also available. Participants were evaluated at baseline, and in 6 to 12-months intervals 8 following initial evaluation for up to 10 years. Initial inclusion criteria were: diagnosis of MCI, 9 mini-mental state examination (MMSE) score of at least 24 points at the time of PET imaging, 10 minimal follow-up time of at least 6 months, no bidirectional change of diagnosis (MCI to AD, 11 and back to MCI) within the follow-up time window. The subjects were dichotomized into MCI 12 patients who converted to AD (MCI converters, MCI-c) and those who did not (MCI non- 13 converters, MCI-nc). 14 The data were randomly split into derivation and validation datasets (Table 1). Age, sex, 15 MMSE, functional activities questionnaire (FAQ) sum score and median follow-up time did not 16 differ significantly among the two datasets (p > 0.1). As would be expected, prevalence of high- 17 risk apolipoprotein E (APOE) genotypes (3/4 and 4/4) was significantly different for MCI-c 18 versus MCI-nc in each of the datasets (p < 0.01) without evidence for any interaction between 19 subgroups and datasets (p > 0.1). 6 PET Analysis 1 PET acquisition details have been described in study protocols of the ADNI project online. 2 In case of 18F-FDG PET, dynamic 3-dimensional scans with six 5-min frames were acquired 30 3 minutes after injection of FDG. All frames were motion-corrected to the first frame and added 4 into a sum file. 18F-FDG PET scans were spatially normalized to an in-house 18F-FDG PET 5 template in Montreal Neurological Institute (MNI) space (8) and smoothed with an isotropic 6 Gaussian kernel of 12 mm full-width at half maximum (FWHM). We assessed the pattern 7 expression score (PES) of the previously validated ADCRP as described before (4). 8 In case of 18F-Florbetapir PET, dynamic 3-dimensional scans with four 5-min frames 9 acquired 50 to 70 minutes after injection were used for analysis (for details see ADNI acquisition 10 protocols). Individual datasets were motion-corrected and summed to create single-image files, 11 followed by spatial normalization to an in-house 18F-Florbetapir PET template in MNI space, 12 constructed of both amyloid positive (n = 9) and negative (n = 7) normal elderly controls scans. 13 Smoothing with an isotropic Gaussian kernel of 12 mm FWHM was applied. For assessment of 14 amyloid load with 18F-Florbetapir PET, we performed voxel-wise PCA on the combined group of 15 MCI-c and MCI-nc of the derivation dataset. To identify a significant pattern, the best 16 combination of the principal components (PCs) that account for the maximal variability in the 17 data was selected by a logistic regression analysis with group (MCI-c and MCI-nc) as the 18 dependent variable and subject scores as the independent variable (as previously described (9)). 19 The obtained Aβ-ADCRP represents spatially covariant voxels associated with the conversion to 20 AD, with each voxel being specifically weighted towards its relative contribution. For the 21 derivation and the validation datasets, PES of Aβ-ADCRP was evaluated by topographic profile 22 rating algorithm (10). 7 1 Additionally, we calculated the standardized uptake value ratio (SUVR) in regions with 2 the highest beta-amyloid burden in AD (Pittsburgh Compound B VOI mask taken from (11)) 3 using the cerebellum as reference region, yielding continuous SUVR values. As a common, 4 clinically used measure, we also defined a binary amyloid status (0 - amyloid negative, 1 - 5 amyloid positive) based on a SUVR cutoff value of 1.3.
Details
-
File Typepdf
-
Upload Time-
-
Content LanguagesEnglish
-
Upload UserAnonymous/Not logged-in
-
File Pages27 Page
-
File Size-