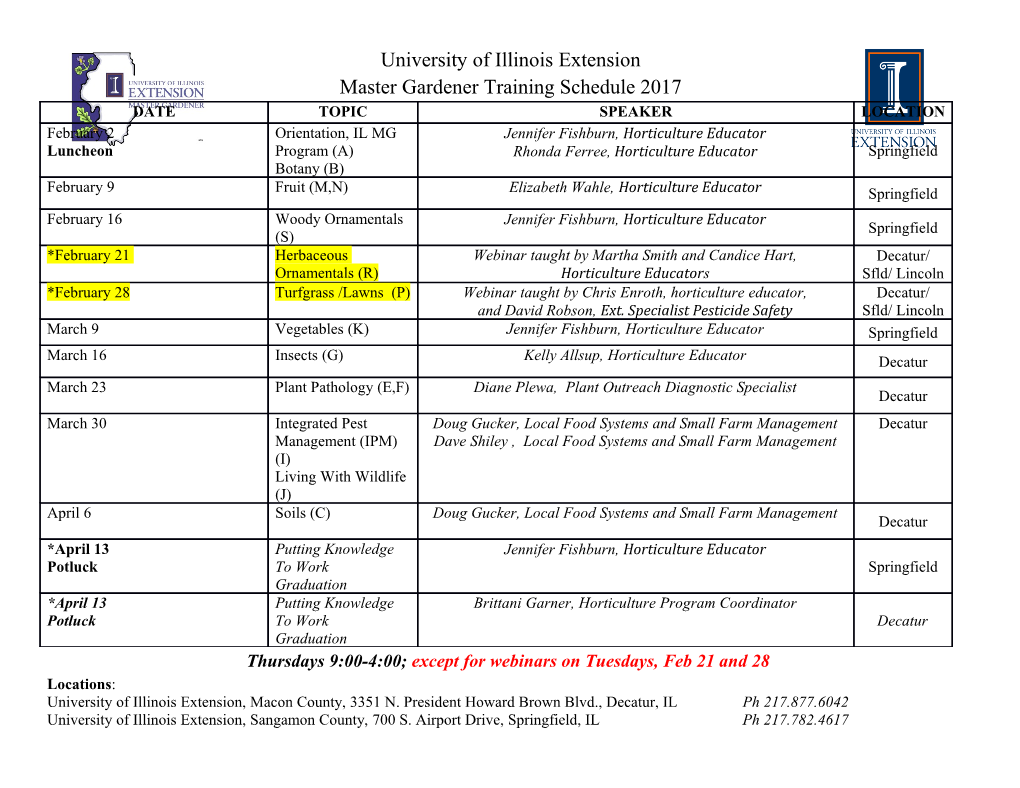
Mandrik 1 The Impact of Hitters on Winning Percentage and Salary Using Sabermetrics Zach Mandrik Professor Deprano 4/11/2017 Economics 490 Directed Research Abstract This paper addresses the question if advanced sabermetrics and traditional statistics are efficient predictors in estimating a hitter’s salary entering free agency. In addition the research also looks to answer if these same statistics can significantly predict a team’s winning percentage. Using data from 1985-2011, the models found that both traditional and advanced baseball metrics are significant in relation to salary and winning percentage. However, all of the regression models have low explanatory power in their ability to forecast results. I suggest that MLB clubs use these models as a checkpoint for free agent player salaries and/or ability to contribute to a team (winning percentage), rather than as an absolute determination of these variables. Mandrik 2 Overview Introduction Literature Review Statistics Review wOBA wRC+ BsR Regression Models The Data What are the outputs telling us? Forecasts and Analysis Conclusion Suggestions for the Sabermetrics Community References Appendix; Graphs and Tables Mandrik 3 Introduction One of the major goals for a baseball franchise, or any professional sports franchise in general, is to ultimately win a championship to bring in fans. Winning as a result typically brings an inflow of revenue, which is an owner’s desire. A portion of building a winning baseball team is centered on statistics and analytics. Thanks to the works of Bill James and many other baseball analysts, the development of sabermetrics has revolutionized the way business is done in baseball. Major League Baseball (MLB) front offices year in and year out use analytics to sign players in the off-season to bolster their rosters. It’s essentially their job to maximize the efficiency of these investments to ensure their team can compete and win more games. The goal of this research is to develop statistical models that predict a hitter’s impact on winning along with forecasting the salary they deserve using both advanced and traditional statistics. The invention of advanced metrics including WAR, weighted runs created (wRC+), weighted on base average (wOBA), and more, provide baseball analysts a deeper understanding of a player’s abilities. Using all of the necessary and available data, I want to test two separate relationships (salary and winning percentage) through regressing a multitude of hitting variables. I hypothesize that the statistics chosen will have a strong relationship with both salary and winning percentage. In addition, I hypothesize these models will enable teams to accurately validate if a player is worth the investment. Mandrik 4 Before diving straight into the results of this paper, I plan to introduce similar studies that address my question in a different manner. This leads directly into a statistics review of the advanced statistics chosen, followed by my reasons why I choose these variables. I’ll explain the origins of the data then reveal the results of the experiment. Lastly, I’ll provide conclusions about sabermetrics and their relationship to salary and winning percentage tied to players entering or continuing free agency. Literature Review Past researchers have analyzed the relationships between a variety of baseball performance variables related to pay and winning. A couple of scholarly articles including Miceli and Huber’s (2009) article in the Journal of Quantitative Analysis in Sports, explain how there is indeed a significant relationship between performance and winning. They also concluded that there isn’t a strong relationship between pay and performance at the team level. To test this hypothesis, Nicholas Miceli and Alan Huber used a factor analysis to distinguish which team-level variables should be included in their regressions. The hitting variables chosen based on their analysis included hits, strikeouts, homeruns, and walks. After running their models they found that pay and performance are not strongly related at the team level. They did however find a statistically significant relationship between performance variables and individual pay, but the practical importance of the relationships (the R squared) was extremely low. Mandrik 5 Miceli and Huber’s models and methods focus on the team level rather than the player level to determine where a team should focus it’s spending. This limits their regression models to using traditional statistics as independent variables to measure predicted salary and winning percentage. My models focus on the use of advanced sabermetrics to test the relationships on salary and winning percentage. In another academic paper, Chang and Zenilman’s “Study of Sabermetrics in Major League Baseball…”(2013) focuses on the impact of sabermetrics on free agents. They created a hedonic pricing model, which included contract length, player height, stolen bases, On-Base plus slugging percentage (OPS), ground into double plays (GDP), and Wins Above Replacement (WAR). With their model, they found that the Moneyball theory1 has tangible and lasting impact on MLB player valuations. Chang and Zenilman (2013) ran regressions using player salary as the dependent variable with all of the previously mentioned independent variables for 3 different time periods. These time periods were labeled as pre-moneyball (before 2000), post- moneyball (2005), and post post-moneyball (2011). As a result, they found increasing significance in certain variables including WAR. As time has passed, WAR has showed an increasing trend in monetary value as well as statistical significance. Although this paper focuses its attention on multiple variables to create a pricing model, these authors revealed the impact of WAR over time on salaries. I’d assume if 1 Chang and Zenilman’s reference to “Moneyball theory” essentially means sabermetrics. Mandrik 6 WAR has had an increasing impact on salary, then other advanced statistics will carry a similar trend. It’s another reason why I analyze the effects of the other advanced statistics available on salary and winning percentage. Statistics Review wOBA Tom Tango, the author of The Book, created weighted on base average (wOBA), which essentially goes beyond standard rate statistics like OPS (on base plus slugging) or batting average (AVG). The purpose of wOBA is to measure a hitter’s overall offensive value based on the relative values of each distinct offensive event (Tango 2007). Unlike On-Base Percentage (OBP) or AVG, wOBA treats each offensive outcome with linear weights to credit the hitter based on the outcome (ex. HR has weight of 2.1). I included wOBA in my models because it’s easy to comprehend because it is scaled similarly to OBP. In addition, the formula is based on a continually changing weight system according to the league average, keeping the statistic current with each passing year. The weight system that creates the seasonal constants is a part of building wOBA. It requires calculating run expectancy matrices for each year to correspond to each year’s player wOBA. In general, “run expectancy measures the average number of Mandrik 7 runs scored (through the end of the current inning) given the current base-out state” (Weinberg 2016). These run expectancies essentially derive the weights, which are scaled based on base percentage (OBP). A further explanation on weights and scaling used for wOBA can be found in Weinberg’s Fangraphs article, “The Beginner’s Guide to Deriving wOBA” (2016). The formula for wOBA can be found in the appendix as formula 1. It basically multiplies each statistic by its corresponding weights in the numerator; unintentional walks (uBB), hit by pitch (HBP), singles, doubles, triples, and home runs. The denominator is simply at bats (AB) plus walks (BB) minus intentional walks (IBB) plus sacrifice flies (SF) plus hit by pitches (HBP). Since weights associated with each variable change annually, the formula included in the appendix does not always contain the same weights in formula 1. wRC+ In baseball, the only way to win is to score more runs than the opposing team. The sabermetrics community improved Bill James’ runs created metric, which measures a hitter’s abilities to provide runs called weighted runs created plus (wRC+) (FanGraphs 2017). Similar to wOBA, wRC+ is a rate statistic that credits a hitter based on the run value of each offensive outcome, but also controls for run environment (ballpark and league). A further detailed explanation on how these factors are calculated can be found at Fangraphs website, www.fangraphs.com (2017). Mandrik 8 Park factors make wRC+ a highly regarded statistics because it values the hitter based on the ballpark they play in. Every ballpark has different distances, altitudes, and other factors, which is why it may be useful to provide this additional context in a statistic. For instance, a hitter who plays at Coors Field (a hitters park due to thinner air) won’t be credited as strongly versus one who hits in Petco Park (pitcher’s park). Because wRC+ is another metric that attempts to capture a player’s overall offensive abilities, I used it as a variable for my models. wRC+ is appealing because it’s measured on an average scale set at 100. For instance, if a player has a wRC+ of 150 that means they are 50 percentage points above the average player in their ability to create runs for their team. It’s an efficient and easy to understand statistic to compare players offensive abilities. The rule of thumb is located in the appendix (Rule of Thumb 1) and indicates the rating scale for wRC+. The formula for wRC+ is from FanGraphs website and can be located in the appendix (Formula 2). In general terms, wRC+ essentially looks at league average metrics compared to the target player while also including park and league factors calculated by FanGraphs. BsR Hitting isn’t the entirety of a player’s ability to score or drive in runs.
Details
-
File Typepdf
-
Upload Time-
-
Content LanguagesEnglish
-
Upload UserAnonymous/Not logged-in
-
File Pages30 Page
-
File Size-