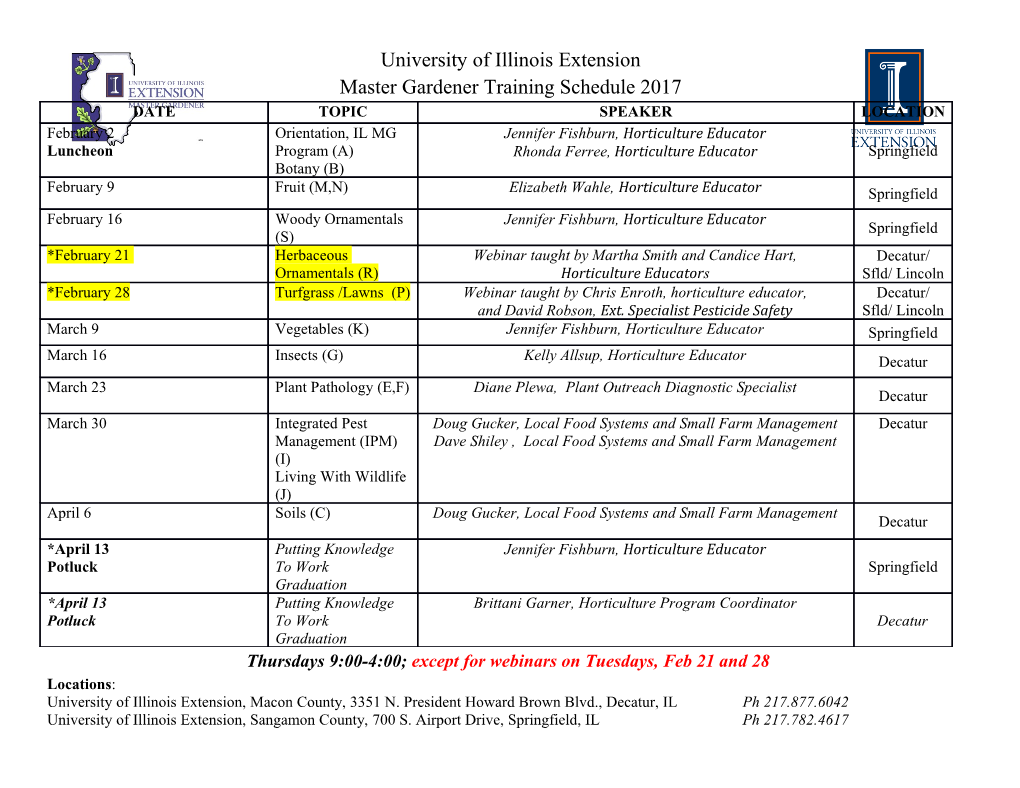
Some Challenging Problems in Mining Social Media Huan Liu Joint work with Shamanth Kumar Ali Abbasi Reza Zafarani Fred Morsta?er Jiliang Tang Arizona State University Data Mining and Machine Learning Lab Some Challenging Problems in Mining Social Media April 22, 2014 1 Social Media Mining by Cambridge University Press hp://dmml.asu.edu/smm/ Arizona State University Data Mining and Machine Learning Lab Some Challenging Problems in Mining Social Media April 22, 2014 22 TradiIonal Media and Data Broadcast Media One-to-Many Communicaon Media One-to-One Tradional Data Arizona State University Data Mining and Machine Learning Lab Some Challenging Problems in Mining Social Media April 22, 2014 3 Social Media: Many-to-Many • Everyone can be a media outlet or producer • Disappearing communicaon barrier • DisHnct characterisHcs – User generated content: Massive, dynamic, extensive, instant, and noisy – Rich user interacHons: Linked data – Collaborave environment, and wisdom of the crowd – Many small groups (the long tail phenomenon) – AenHon is expensive Arizona State University 4 Data Mining and Machine Learning Lab Some Challenging Problems in Mining Social Media April 22, 2014 4 Unique Features of Social Media • Novel phenomena observed from people’s interacons in social media • Unprecedented opportuniHes for interdisciplinary and collabora(ve research – How to use social media to study human behavior? • It’s rich, noisy, free-form, and definitely BIG – With so much data, how can we make sense of it? • Pung “bricks” into a useful (meaningful) “edifice” • Developing new methods/tools for social media mining Arizona State University Data Mining and Machine Learning Lab Some Challenging Problems in Mining Social Media April 22, 2014 5 Some Challenges in Mining Social Media • Evaluaon Dilemma – How to evaluate without convenHonal test data? • Sampling Bias – O`en we get a small sample of (sHll big) data. How can we ensure if the data can lead to credible findings? • Noise-Removal Fallacy – How do we remove noise without losing too much? • Studying Distrust in Social Media – Is distrust simply the negaon of trust? Where to find distrust informaon with “one-way” relaons? Arizona State University Data Mining and Machine Learning Lab Some Challenging Problems in Mining Social Media April 22, 2014 6 Evaluaon Dilemma • Evaluaon is important in data mining – TradiHonal test data is o`en not available in social media mining • Can we evaluate our findings without ground truth? • A case study of Migra(on in Social Media – Users are a primary source of revenue – New social media sites need to aract users – ExisHng sites need to retain their users – CompeHHon for precious aenHon Arizona State University Data Mining and Machine Learning Lab Some Challenging Problems in Mining Social Media April 22, 2014 77 MigraIon in Social Media • What is migraon? – Migraon can be described as the movement of users away from one locaon toward another, either due to necessity, or aracHon to the new environment. • Two types of migraon Site 2 Aer Site 2 Site 3 – me t Site migraon Site 1 Site 3 Site 2 Site 2 – AQenHon migraon Aer me t Site 1 Site 3 Site 1 Site 3 Arizona State University Data Mining and Machine Learning Lab Some Challenging Problems in Mining Social Media April 22, 2014 8 Obtaining User MigraIon Pa?erns • Goal: IdenHfying trends of aenHon migraon of users across the two phases of the collected data. • Process Arizona State University Data Mining and Machine Learning Lab Some Challenging Problems in Mining Social Media April 22, 2014 9 Paerns from Observaon Arizona State University Data Mining and Machine Learning Lab Some Challenging Problems in Mining Social Media April 22, 2014 10 Facing an EvaluaIon Dilemma • Important to know if they are some meaningful paerns – If yes, we invesHgate further how we use the paerns for prevenHon or promoHon – If not, why not? And what can we do? • We would like to evaluate migraon paerns, but without ground truth • How? – User study or AMT? Arizona State University Data Mining and Machine Learning Lab Some Challenging Problems in Mining Social Media April 22, 2014 11 Evaluang Paerns’ Validity • One way is to verify if these paerns are fortuitous • Null Hypothesis: Migra(on in social media is a random process – Generang another similar dataset for comparison • PotenHal migrang populaon includes overlapping users from Phase 1 and Phase 2 • Shuffled datasets are generated by picking random acHve users from the potenHal migrang populaon • The number of random users selected for each dataset is the same as the real migrang populaon Arizona State University Data Mining and Machine Learning Lab Some Challenging Problems in Mining Social Media April 22, 2014 12 A Significance Test Coefficients Shuffled dataset LogisHc Regression of user aributes Comparing and Chi Square StasHc Sig. Test Observed Coefficients migraon LogisHc Regression of user dataset aributes Arizona State University Data Mining and Machine Learning Lab Some Challenging Problems in Mining Social Media April 22, 2014 13 EvaluaIon Results • Significant differences observed in StumbleUpon, TwiQer, and YouTube • Paerns from other sites are not stasHcally significant. PotenHal cause: – Insufficient Data? Arizona State University Data Mining and Machine Learning Lab Some Challenging Problems in Mining Social Media April 22, 2014 14 Summary • MiHgang or promoHng migraon by targeHng high net-worth individuals – IdenHfying users with high value to the network, e.g., high network acHvity, user acHvity, and external exposure • Social media migraon is first studied in this work • Alternave evaluaon approaches can help address the evaluaon dilemma Understanding User Migraon Paerns in Social Media, S. Kumar, R. Zafarani, and H. Liu, AAAI’2010 Arizona State University Data Mining and Machine Learning Lab Some Challenging Problems in Mining Social Media April 22, 2014 15 Some Challenges in Mining Social Media • Evaluaon Dilemma • Sampling Bias • Noise-Removal Fallacy • Studying Distrust in Social Media Arizona State University Data Mining and Machine Learning Lab Some Challenging Problems in Mining Social Media April 22, 2014 16 Sampling Bias in Social Media Data • TwiQer provides two main outlets for researchers to access tweets in real Hme: – Streaming API (~1% of all public tweets, free) – Firehose (100% of all public tweets, costly) • Streaming API data is o`en used to by researchers to validate hypotheses. • How well does the sampled Streaming API data measure the true acHvity on TwiQer? Arizona State University Data Mining and Machine Learning Lab Some Challenging Problems in Mining Social Media April 22, 2014 1717 Facets of Twi?er Data • Compare the data along different facets • Selected facets commonly used in social media mining: – Top Hashtags – Topic Extracon – Network Measures – Geographic DistribuHons Arizona State University Data Mining and Machine Learning Lab Some Challenging Problems in Mining Social Media April 22, 2014 18 Preliminary Results Top Hashtags Topic Extracon • No clear correlaon between • Topics are close to those Streaming and Firehose data. found in the Firehose. Network Measures Geographic Distribuons • Found ~50% of the top • Streaming data gets >90% of tweeters by different the geotagged tweets. centrality measures. • Consequently, the distribuHon • Graph-level measures give of tweets by conHnent is very similar results between the similar. two datasets. Arizona State University Data Mining and Machine Learning Lab Some Challenging Problems in Mining Social Media April 22, 2014 19 How are These Results? • Accuracy of streaming API can vary with analysis to be performed • These results are about single cases of streaming API • Are these findings significant, or just an arHfact of random sampling? • How do we verify that our results indicate sampling bias or not? Arizona State University Data Mining and Machine Learning Lab Some Challenging Problems in Mining Social Media April 22, 2014 20 Histogram of JS Distances in Topic Comparison • This is just one streaming dataset against Firehose • Are we confident about this set of results? • Can we leverage another streaming dataset? • Unfortunately, we cannot rewind as we have only one streaming dataset Arizona State University Data Mining and Machine Learning Lab Some Challenging Problems in Mining Social Media April 22, 2014 21 Verificaon • Created 100 of our own “Streaming API” results by sampling the Firehose data. Genera2ng&Random&Samples& 60" 50" 40" 30" Numer&of&tweets&(k)& 20" 10" 0" Firehose" Streaming" Random"1" Random"2" …" Random"100" Arizona State University Data Mining and Machine Learning Lab Some Challenging Problems in Mining Social Media April 22, 2014 22 Comparison with Random Samples Arizona State University Data Mining and Machine Learning Lab Some Challenging Problems in Mining Social Media April 22, 2014 23 Summary • Streaming API data could be biased in some facets • Our results were obtained with the help of Firehose • Without Firehose data, it’s challenging to figure out which facets might have bias, and how to compensate them in search of credible mining results F. Morstaer, J. Pfeffer, H. Liu, and K. Carley. Is the Sample Good Enough? Comparing Data from TwiAer’s Streaming API and Data from TwiAer’s Firehose. ICWSM, 2013. Fred Morstaer, Jürgen Pfeffer, Huan Liu. When is it Biased? Assessing the Representa(veness of TwiAer's Streaming API, WWW Web Science 2014. Arizona State University Data Mining and Machine Learning Lab Some Challenging Problems in Mining Social Media April 22, 2014 24 Some
Details
-
File Typepdf
-
Upload Time-
-
Content LanguagesEnglish
-
Upload UserAnonymous/Not logged-in
-
File Pages53 Page
-
File Size-