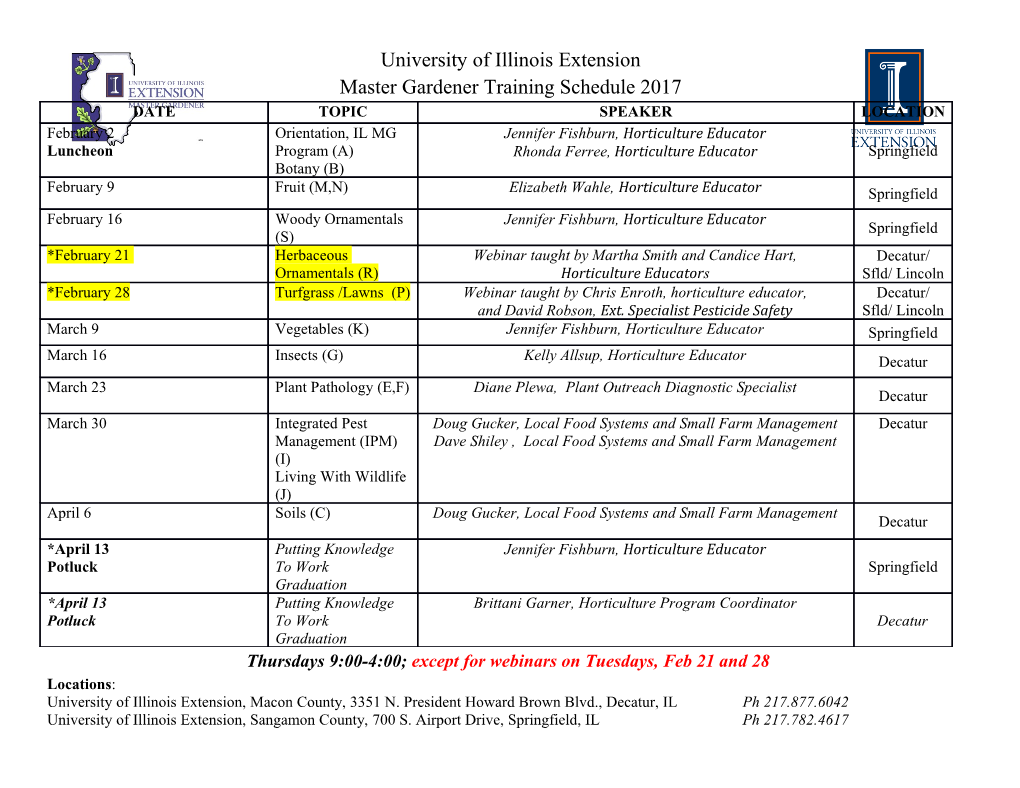
Meta-analysis of Gene Expression in Individuals with Autism Spectrum Disorders by Carolyn Lin Wei Ch’ng BSc., University of Michigan Ann Arbor, 2011 A THESIS SUBMITTED IN PARTIAL FULFILLMENT OF THE REQUIREMENTS FOR THE DEGREE OF Master of Science in THE FACULTY OF GRADUATE AND POSTDOCTORAL STUDIES (Bioinformatics) The University of British Columbia (Vancouver) August 2013 c Carolyn Lin Wei Ch’ng, 2013 Abstract Autism spectrum disorders (ASD) are clinically heterogeneous and biologically complex. State of the art genetics research has unveiled a large number of variants linked to ASD. But in general it remains unclear, what biological factors lead to changes in the brains of autistic individuals. We build on the premise that these heterogeneous genetic or genomic aberra- tions will converge towards a common impact downstream, which might be reflected in the transcriptomes of individuals with ASD. Similarly, a considerable number of transcriptome analyses have been performed in attempts to address this question, but their findings lack a clear consensus. As a result, each of these individual studies has not led to any significant advance in understanding the autistic phenotype as a whole. The goal of this research is to comprehensively re-evaluate these expression profiling studies by conducting a systematic meta-analysis. Here, we report a meta-analysis of over 1000 microarrays across twelve independent studies on expression changes in ASD compared to unaffected individuals, in blood and brain. We identified a number of genes that are consistently differentially expressed across studies of the brain, suggestive of effects on mitochondrial function. In blood, consistent changes were more difficult to identify, despite individual studies tending to exhibit larger effects than the brain studies. Our results are the strongest evidence to date of a common transcriptome signature in the brains of individuals with ASD. ii Preface Under the supervision of Dr. Paul Pavlidis, I conducted and authored the work presented henceforth. Willie Kwok performed preliminary research under the mentorship of Dr. Sanja Rogic, who, together with Dr. Paul Pavlidis, contributed to the development of this project. A version of this work will be submitted to a peer reviewed journal for publication. Carolyn Ch’ng, Willie Kwok, Sanja Rogic, Paul Pavlidis. Meta-analysis of expression profiles in the blood and brains of individuals with autism spectrum disorders (in preparation). Eloi Mercier provided all the aggregated networks for the network analysis in Chapter 2. Portions of Chapter 2 are used with permission from Portales-Casamar et al., of which I am a second author. Elodie Portales-Casamar, Carolyn Ch’ng, Frances Lui, Nicolas St- Georges, Anton Zoubarev, Artemis Y. Lai, Mark Lee, Cathy Kwok, Willie Kwok, Luchia Tseng, and Paul Pavlidis. Neurocarta: aggregating and sharing disease-gene relations for the neurosciences. BMC Genomics, 14(1):129, February 2013. ISSN 1471-2164. doi:10.1186/1471-2164-14-129. URL http://www.biomedcentral.com/1471-2164/14/129/ abstract. PMID: 23442263. iii Table of Contents Abstract . ii Preface . iii Table of Contents . iv List of Tables . vi List of Figures . ix Glossary . xi Acknowledgments . xii Dedication . xiii 1 Introduction . 1 1.1 History and early theories in autism . 2 1.2 Emerging theories in autism genetics . 3 1.3 The search for convergence in the autism spectrum . 5 1.3.1 Transcriptomic analysis in ASD . 7 1.4 Meta-analysis in neuropsychiatry . 8 2 Meta-analysis of gene expression profiles in the blood and brain tissues of individuals with autism spectrum disorders . 10 2.1 Methods . 10 2.1.1 Data retrieval, preprocessing and quality control . 10 2.1.2 Re-analysis of differential expression in existing autism data sets . 17 2.1.3 Meta-analysis of differentially expressed genes . 18 2.1.4 Functional enrichment analysis . 21 iv 2.1.5 Literature derived ASD candidates . 22 2.1.6 Copy number variation enrichment analysis and prediction classifier 22 2.1.7 Network analysis . 23 2.2 Results . 24 2.2.1 Systematic review shows technical differences and heterogeneity in independent Autism Spectrum Disorders (ASD) transcriptome studies 24 2.2.2 Re-analysis for differential expression . 27 2.2.3 Meta-analysis of differential expression . 29 2.2.4 Robust molecular commonalities are more evident in brain samples compared to blood . 32 2.2.5 Functional analyses reveal perturbations in metabolic processes . 39 2.2.6 Shared signatures between autism and other neurodevelopmental syndromes . 40 2.2.7 Meta-signature genes in rare structural variants associated with ASD 41 2.2.8 Network analysis and candidate gene characterization . 45 3 Discussion and conclusion . 50 3.1 Similarities and differences between key findings and previous results . 50 3.2 Biological interpretations of meta-analyzed ASD expression profiles . 54 3.3 Limitations and future directions . 55 3.4 Conclusion . 56 Bibliography . 57 A Appendix . 72 v List of Tables Table 1.1 Data sets from transcriptomic analysis in ASD............... 6 Table 2.1 Summary of platform annotations from Gemma. Number of probes and unique genes for each platform were obtained from the Gemma platform database. 12 Table 2.2 Summary of tissue sources. 13 Table 2.3 Samples excluded in each study. 14 Table 2.4 Summary of diagnosis criteria and ASD phenotypes in the original stud- ies. Refer to Table 1.1 for study citations. 25 Table 2.5 Demographics I - Gender. Gender imbalance is seen in some data sets, such as GSE37772. OR: Odds ratio. 26 Table 2.6 Demographics II - Age, PMI and race of subjects in each study. C: Caucasian or white; AA: African American; A: Asian; M: Mixed or multiracial; U: Unknown . 26 Table 2.7 Differentially expressed genes in each data set after re-analysis. DE: Differentially expressed genes at FDR threshold of 0.05; Up: Up-regulated genes; Down: Down-regulated genes; Number of genes: Number of genes after applying filters. 27 Table 2.8 Overlap between results reported in the literature and individual re- anal- ysis of differential expression. Significant probes: Per data set signifi- cant probes from re-analysis, reported at an false discovery rate (FDR) threshold of 0.05. Probes reported: Differentially expressed probes published in original papers of each study. Gene symbols are used as a proxy for probes in GSE18123.1; GenBank accessions are used in GSE15451 and GSE15402; Spot IDs are used for GSE7329. GSE25507 computed differences in expression variance instead of differential ex- pression; GSE37772 reported outlier genes instead of differentially ex- pressed genes; GSE32136 is not published. 29 vi Table 2.9 Overlap (overlap/total up or down-regulated in data set) between meta- signature (FDR <0.05) and significantly differentially expressed genes per data set (FDR <0.05), as well as enrichment of meta-signatures in the results of individual differential expression analysis. One sided p- values were used to compute FDR here. AU-ROC: area under receiver operating characteristic curve; AP: average precision. 31 Table 2.10 Comparisons of blood and brain signatures. AU-ROC reported for sig- nature of tissue A on ranked gene list from meta-analysis of tissue B (A-B). 32 Table 2.11 Top genes in the “cellular respiration” Gene Ontology (GO) category at a meta-analysis raw p-value threshold of 0.0001. There are a total of 116 genes in this functional group. 39 Table 2.12 Top genes in the Simons Foundation Autism Research Initiative (SFARI) “syndromic” category at a meta-analysis raw p-value threshold of 0.05. There are a total of 19 genes in this gene set. 40 Table 2.13 Dysregulated genes (FDR <0.05, meta-signature) within ASD-associated CNV. Fisher’s exact test was used to compute significance. NS: Not sig- nificant. 42 Table 2.14 Dysregulated genes in the brain that are found in known ASD CNVs. CNVs that span the same gene or set of genes are grouped together. 42 Table 2.15 Dysregulated genes in the blood that are found in known ASD CNVs. 44 Table 2.16 Predictions on GSE37772 samples using preliminary copy number vari- ation (CNV) classifier. CV: cross validated; SV: support vectors; AU- ROC: AU-ROC computed for other 15q samples (originally predicted but not confirmed). 45 Table 2.17 Categorization of our candidate genes based on Neurocarta. Ndev.: neu- rodevelopment. 48 Table 2.18 Meta-signature genes that are also dysregulated in schizophrenia. Meta- analysis FDR <0.1 . 49 Table 3.1 Comparisons between core signature genes in blood and differentially expressed genes reported in original studies. Total hits: Total hits re- ported in original study (Genes); Total genes analyzed: Estimated total number of genes analyzed in each study based on Gemma platform an- notations. 52 Table 3.2 Similar to Table 3.1, for core signature genes in the brain. 53 vii Table A.1 Up-regulated brain meta-signature. FDR Computed before removal of sex-biased genes. A: Known Candidate; B: Gender Biased; C: Known CNV. Y: Yes; N: No. 72 Table A.2 Down-regulated brain meta-signature. FDR Computed before removal of sex-biased genes. A: Known Candidate; B: Gender Biased; C: Known CNV. Y: Yes; N: No. 73 Table A.3 Up-regulated blood meta-signature. FDR Computed before removal of sex-biased genes. A: Known Candidate; B: Gender Biased; C: Known CNV. Y: Yes; N: No. 75 Table A.4 Down-regulated blood meta-signature. FDR Computed before removal of sex-biased genes. A: Known Candidate; B: Gender Biased; C: Known CNV. Y: Yes; N: No. 79 Table A.5 Genes that have been shown to exhibit sexual dimorphism in blood and brain. Asterisks denote known ASD candidates. 83 viii List of Figures Figure 1.1 Two possible models leading to similar behavioral characteristics in ASD.
Details
-
File Typepdf
-
Upload Time-
-
Content LanguagesEnglish
-
Upload UserAnonymous/Not logged-in
-
File Pages96 Page
-
File Size-