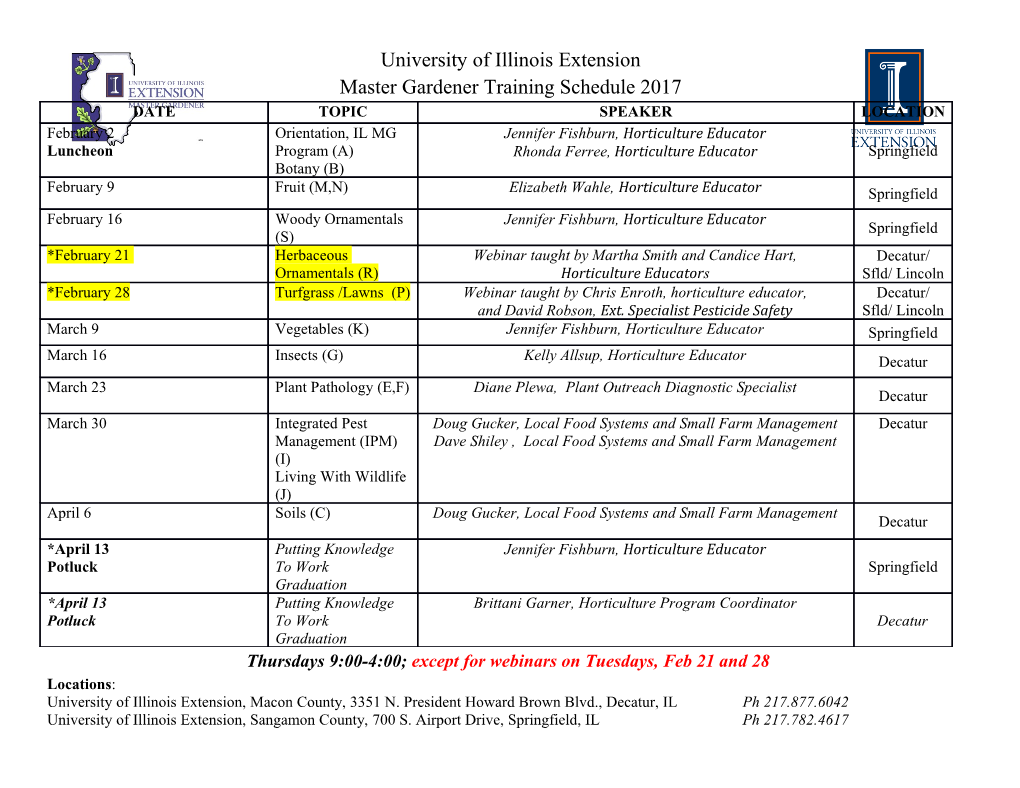
Introduction to Machine Learning (67577) Lecture 10 Shai Shalev-Shwartz School of CS and Engineering, The Hebrew University of Jerusalem Neural Networks Shai Shalev-Shwartz (Hebrew U) IML Lecture 10 Neural Networks 1 / 31 Outline 1 Neural networks 2 Sample Complexity 3 Expressiveness of neural networks 4 How to train neural networks ? Computational hardness SGD Back-Propagation 5 Convolutional Neural Networks (CNN) 6 Feature Learning Shai Shalev-Shwartz (Hebrew U) IML Lecture 10 Neural Networks 2 / 31 A Single Artificial Neuron A single neuron is a function of the form x 7! σ(hv; xi), where σ : R ! R is called the activation function of the neuron x1 v1 x2 v2 v3 x3 σ(hv; xi) v4 x4 v5 x5 E.g., σ is a sigmoidal function Shai Shalev-Shwartz (Hebrew U) IML Lecture 10 Neural Networks 3 / 31 We focus on feedforward networks, formally defined by a directed acyclic graph G = (V; E) Input nodes: nodes with no incoming edges Output nodes: nodes without out going edges weights: w : E ! R Calculation using breadth-first-search (BFS), where each neuron (node) receives as input: X a[v] = w[u ! v]o[u] u!v2E and output o[v] = σ(a[v]) Neural Networks A neural network is obtained by connecting many neurons together Shai Shalev-Shwartz (Hebrew U) IML Lecture 10 Neural Networks 4 / 31 Input nodes: nodes with no incoming edges Output nodes: nodes without out going edges weights: w : E ! R Calculation using breadth-first-search (BFS), where each neuron (node) receives as input: X a[v] = w[u ! v]o[u] u!v2E and output o[v] = σ(a[v]) Neural Networks A neural network is obtained by connecting many neurons together We focus on feedforward networks, formally defined by a directed acyclic graph G = (V; E) Shai Shalev-Shwartz (Hebrew U) IML Lecture 10 Neural Networks 4 / 31 Output nodes: nodes without out going edges weights: w : E ! R Calculation using breadth-first-search (BFS), where each neuron (node) receives as input: X a[v] = w[u ! v]o[u] u!v2E and output o[v] = σ(a[v]) Neural Networks A neural network is obtained by connecting many neurons together We focus on feedforward networks, formally defined by a directed acyclic graph G = (V; E) Input nodes: nodes with no incoming edges Shai Shalev-Shwartz (Hebrew U) IML Lecture 10 Neural Networks 4 / 31 weights: w : E ! R Calculation using breadth-first-search (BFS), where each neuron (node) receives as input: X a[v] = w[u ! v]o[u] u!v2E and output o[v] = σ(a[v]) Neural Networks A neural network is obtained by connecting many neurons together We focus on feedforward networks, formally defined by a directed acyclic graph G = (V; E) Input nodes: nodes with no incoming edges Output nodes: nodes without out going edges Shai Shalev-Shwartz (Hebrew U) IML Lecture 10 Neural Networks 4 / 31 Calculation using breadth-first-search (BFS), where each neuron (node) receives as input: X a[v] = w[u ! v]o[u] u!v2E and output o[v] = σ(a[v]) Neural Networks A neural network is obtained by connecting many neurons together We focus on feedforward networks, formally defined by a directed acyclic graph G = (V; E) Input nodes: nodes with no incoming edges Output nodes: nodes without out going edges weights: w : E ! R Shai Shalev-Shwartz (Hebrew U) IML Lecture 10 Neural Networks 4 / 31 Neural Networks A neural network is obtained by connecting many neurons together We focus on feedforward networks, formally defined by a directed acyclic graph G = (V; E) Input nodes: nodes with no incoming edges Output nodes: nodes without out going edges weights: w : E ! R Calculation using breadth-first-search (BFS), where each neuron (node) receives as input: X a[v] = w[u ! v]o[u] u!v2E and output o[v] = σ(a[v]) Shai Shalev-Shwartz (Hebrew U) IML Lecture 10 Neural Networks 4 / 31 Multilayer Neural Networks T Neurons are organized in layers: V = [· t=0Vt, and edges are only between adjacent layers Example of a multilayer neural network of depth 3 and size 6 Input Hidden Hidden Output layer layer layer layer x1 x2 x3 x4 x5 Shai Shalev-Shwartz (Hebrew U) IML Lecture 10 Neural Networks 5 / 31 We refer to (V; E; σ) as the architecture, and it defines a hypothesis class by HV;E,σ = fhV;E,σ;w : w is a mapping from E to Rg : The architecture is our \Prior knowledge" and the learning task is to find the weight function w We can now study estimation error (sample complexity) approximation error (expressivenss) optimization error (computational complexity) Neural Networks as a Hypothesis Class Given a neural network (V; E; σ; w), we obtain a hypothesis jV |−1 jV j hV;E,σ;w : R 0 ! R T Shai Shalev-Shwartz (Hebrew U) IML Lecture 10 Neural Networks 6 / 31 The architecture is our \Prior knowledge" and the learning task is to find the weight function w We can now study estimation error (sample complexity) approximation error (expressivenss) optimization error (computational complexity) Neural Networks as a Hypothesis Class Given a neural network (V; E; σ; w), we obtain a hypothesis jV |−1 jV j hV;E,σ;w : R 0 ! R T We refer to (V; E; σ) as the architecture, and it defines a hypothesis class by HV;E,σ = fhV;E,σ;w : w is a mapping from E to Rg : Shai Shalev-Shwartz (Hebrew U) IML Lecture 10 Neural Networks 6 / 31 We can now study estimation error (sample complexity) approximation error (expressivenss) optimization error (computational complexity) Neural Networks as a Hypothesis Class Given a neural network (V; E; σ; w), we obtain a hypothesis jV |−1 jV j hV;E,σ;w : R 0 ! R T We refer to (V; E; σ) as the architecture, and it defines a hypothesis class by HV;E,σ = fhV;E,σ;w : w is a mapping from E to Rg : The architecture is our \Prior knowledge" and the learning task is to find the weight function w Shai Shalev-Shwartz (Hebrew U) IML Lecture 10 Neural Networks 6 / 31 Neural Networks as a Hypothesis Class Given a neural network (V; E; σ; w), we obtain a hypothesis jV |−1 jV j hV;E,σ;w : R 0 ! R T We refer to (V; E; σ) as the architecture, and it defines a hypothesis class by HV;E,σ = fhV;E,σ;w : w is a mapping from E to Rg : The architecture is our \Prior knowledge" and the learning task is to find the weight function w We can now study estimation error (sample complexity) approximation error (expressivenss) optimization error (computational complexity) Shai Shalev-Shwartz (Hebrew U) IML Lecture 10 Neural Networks 6 / 31 Outline 1 Neural networks 2 Sample Complexity 3 Expressiveness of neural networks 4 How to train neural networks ? Computational hardness SGD Back-Propagation 5 Convolutional Neural Networks (CNN) 6 Feature Learning Shai Shalev-Shwartz (Hebrew U) IML Lecture 10 Neural Networks 7 / 31 Theorem: The VC dimension of HV;E,σ, for σ being the sigmoidal function, is Ω(jEj2). Representation trick: In practice, we only care about networks where each weight is represented using O(1) bits, and therefore the VC dimension of such networks is O(jEj), no matter what σ is We can further decrease the sample complexity by many kinds of regularization functions (this is left for an advanced course) Sample Complexity Theorem: The VC dimension of HV;E;sign is O(jEj log(jEj)). Shai Shalev-Shwartz (Hebrew U) IML Lecture 10 Neural Networks 8 / 31 Representation trick: In practice, we only care about networks where each weight is represented using O(1) bits, and therefore the VC dimension of such networks is O(jEj), no matter what σ is We can further decrease the sample complexity by many kinds of regularization functions (this is left for an advanced course) Sample Complexity Theorem: The VC dimension of HV;E;sign is O(jEj log(jEj)). Theorem: The VC dimension of HV;E,σ, for σ being the sigmoidal function, is Ω(jEj2). Shai Shalev-Shwartz (Hebrew U) IML Lecture 10 Neural Networks 8 / 31 We can further decrease the sample complexity by many kinds of regularization functions (this is left for an advanced course) Sample Complexity Theorem: The VC dimension of HV;E;sign is O(jEj log(jEj)). Theorem: The VC dimension of HV;E,σ, for σ being the sigmoidal function, is Ω(jEj2). Representation trick: In practice, we only care about networks where each weight is represented using O(1) bits, and therefore the VC dimension of such networks is O(jEj), no matter what σ is Shai Shalev-Shwartz (Hebrew U) IML Lecture 10 Neural Networks 8 / 31 Sample Complexity Theorem: The VC dimension of HV;E;sign is O(jEj log(jEj)). Theorem: The VC dimension of HV;E,σ, for σ being the sigmoidal function, is Ω(jEj2). Representation trick: In practice, we only care about networks where each weight is represented using O(1) bits, and therefore the VC dimension of such networks is O(jEj), no matter what σ is We can further decrease the sample complexity by many kinds of regularization functions (this is left for an advanced course) Shai Shalev-Shwartz (Hebrew U) IML Lecture 10 Neural Networks 8 / 31 Outline 1 Neural networks 2 Sample Complexity 3 Expressiveness of neural networks 4 How to train neural networks ? Computational hardness SGD Back-Propagation 5 Convolutional Neural Networks (CNN) 6 Feature Learning Shai Shalev-Shwartz (Hebrew U) IML Lecture 10 Neural Networks 9 / 31 What type of functions from {±1gn to {±1g can be implemented by HV;E;sign ? Theorem: For every n, there exists a graph (V; E) of depth 2, such n that HV;E;sign contains all functions from {±1g to {±1g Theorem: For every n, let s(n) be the minimal integer such that there exists a graph (V; E) with jV j = s(n) such that the hypothesis n class HV;E;sign contains all the functions from f0; 1g to f0; 1g.
Details
-
File Typepdf
-
Upload Time-
-
Content LanguagesEnglish
-
Upload UserAnonymous/Not logged-in
-
File Pages72 Page
-
File Size-