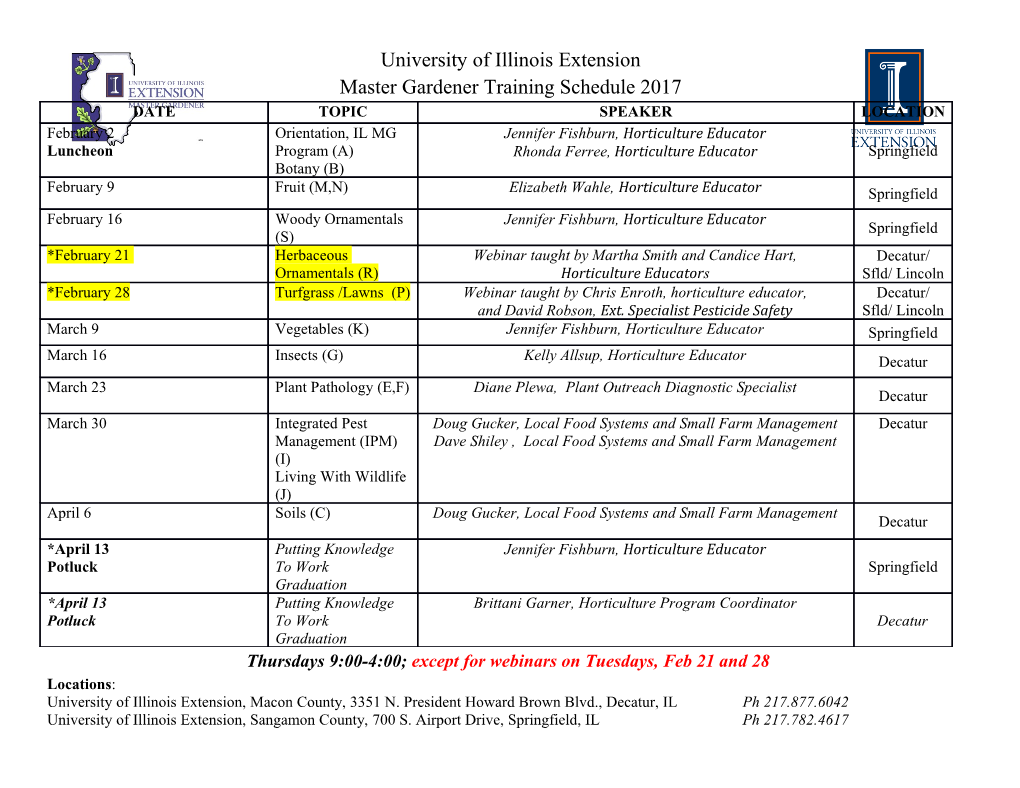
A New Learning Method for Inference Accuracy, Core Occu- pation, and Performance Co-optimization on TrueNorth Chip 1 Wei Wen, 1 Chunpeng Wu, 1 Yandan Wang, 1 Kent Nixon, 2 Qing Wu, 2 Mark Barnell, 1 Hai Li, 1 Yiran Chen 1 University of Pittsburgh, Pittsburgh, PA, USA; 2 Air Force Research Laboratory, Rome, NY, USA 1{wew57, chw127, yaw46, kwn2, hal66, yic52}@pitt.edu, 2{qing.wu.2, Mark.Barnell.1}@us.af.mil ABSTRACT ues. Besides the stochastic scheme, TrueNorth also supports many IBM TrueNorth chip uses digital spikes to perform neuromor- deterministic neural coding schemes (i.e., rate code, population phic computing and achieves ultrahigh execution parallelism and code, time-to-spike code and rank code [9]) in temporal domain to power efficiency. However, in TrueNorth chip, low quantization represent non-binary message using multiple binary spikes. Rate resolution of the synaptic weights and spikes significantly limits code, for example, uses the number of the spikes occurring within the inference (e.g., classification) accuracy of the deployed neural a predefined number of time steps to indicate the amplitude of a network model. Existing workaround, i.e., averaging the results signal [7]. However, this “official” workaround rapidly exhausts over multiple copies instantiated in spatial and temporal domains, hardware resources when the network scale increases and a large rapidly exhausts the hardware resources and slows down the number of spatial samples (copies) are instantiated. It also slows computation. In this work, we propose a novel learning method on down the computation when many spike samples are generated. TrueNorth platform that constrains the random variance of each In this work, we first formulate the standard TrueNorth learning computation copy and reduces the number of needed copies. and deploying method and study its compatibility to learning net- Compared to the existing learning method, our method can works with floating-point precision; we then analyze the essential achieve up to 68.8% reduction of the required neuro-synaptic factors that affect the accuracy of TrueNorth during data quantiza- cores or 6.5× speedup, with even slightly improved inference tion and task deployment; finally, we propose a new learning accuracy. method to mitigate the adverse impacts of these factors on True- North accuracy by constraining the variance of each copy through 1. INTRODUCTION biasing the randomness of synaptic connectivity in neuro-synaptic Von Neumann architecture has achieved remarkable success in cores. Experiments executed on real TrueNorth chip shows that the history of computer industry. However, following the upscal- our proposed scheme can achieve up to 68.8% reduction of the ing of design complexity and clock frequency of modern comput- required neuro-synaptic cores or 6.5× performance speedup, with er systems, power wall [1][2] and memory wall [3], which denote even slightly improved inference (e.g., classification) accuracy. the high power density and low memory bandwidth [4][5] w.r.t. the fast on-chip data processing capability, become two major 2. PRELIMINARY obstacles hindering the deployment of von Neumann architecture A TrueNorth chip is made of a network of neuro-synaptic cores. in data-intensive applications. Neuromorphic computer, therefore, Each neuro-synaptic core includes a 256×256 configurable synap- starts to gain attentions in computer architecture society due to its tic crossbar connecting 256 axons and 256 neurons in close prox- extremely high data processing efficiency for cognitive applica- imity [7]. The inter-core communication is transmitted by spike tions: different from decoupling storage and computation as von events through axons. Determined by the axon type at each neu- Neumann architecture does, neuromorphic system directly maps ron, the weight of the corresponding synapse in the crossbar is the neural networks to distributed computing units each of which selected from 4 possible integers. integrates both computing logic and memory [1][6][7]. As illustrated in Figure 1(a), in an artificial neural network, the In 2014 April, IBM unveiled TrueNorth - a new neuromorphic output of a neuron (z) is determined by the input vector (x) and system composed of 4096 neuro-synaptic cores, 1 million neu- the weights of the connections to the neuron (w), such as rons, and 256 million synapses. Memories (“synapses”) and com- yb=wx⋅+, and (1) puting units (“neurons”) of each neuro-synaptic core are placed in close proximity and the neuro-synaptic cores are connected zhy= (). (2) through “axons” network. TrueNorth chip is extremely efficient in executing neural networks and machine learning algorithms and Here b is a bias. h(·) is a nonlinear activation function. The data in (1) and (2) usually are represented with floating-point precision. able to achieve a peak computation of 58 Giga synaptic operations per second at a power consumption of 145mW [1][8]. When mapping the neural network to a neuro-synaptic core in The high computing efficiency and parallelism of TrueNorth TrueNorth, as shown in Figure 1(b), the input vector x is quan- are achieved by sacrificing the precision of message stored and tized into the spike format x' and the weights w are approximated propagated in networks: inter-core communication is performed using binary spikes and synaptic weights are quantized by low- resolution integers [9]. It is contradictory to common practices of deep learning/neural networks that require some high precisions [10]. Directly deploying the learned model with a low precision on TrueNorth leads to inevitable inference accuracy degradation. To compensate this accuracy loss, the current workaround of TrueNorth is to average the results over multiple copies instantiat- ed in spatial and temporal domains: In spatial domain, TrueNorth utilizes stochastic neural mode to mimic fractional synaptic weights [9]. The similar stochastic scheme can be also applied to temporal domain to generate spikes with fractional expected val- Figure 1. Mapping neural networks in IBM TrueNorth. by random synaptic weight samples w'. More specific, a pseudo ference accuracy of the neural network deployed on TureNorth. random number generator (PRNG) is used to sample each connec- This section will provide the detailed theoretical explanation of tion in the synaptic crossbar: when the synapse is sampled to be our proposed learning method. We first formulate and analyze the “ON”, the value indexed by the axon type will be applied as its learning method of TrueNorth, namely, Tea learning [11], and weight; otherwise the synapse is disconnected. For the neuron analyze its compatibility to the deep learning models. We then implementation, rather than using nonlinear activation functions conduct a theoretical analysis of the expectation and variance of in traditional neural networks, TrueNorth adopts a spiking neuron TrueNorth deployment. Based on this analysis, we propose our model − leaky integrate-and-fire (LIF) model [9] to produce out- probability-biased learning method. put in digital spike format. The model has 22 parameters (14 of which are user configurable) and 8 complex neuron specification 3.1 The Learning and Deploying of TrueNorth equations. Regardless the possible versatility and complexity of Figure 2 gives an overview of the learning and deploying of a LIF designs, a simple version of LIF, e.g., McCulloch-Pitts neu- neural network on TrueNorth, which is named as Tea learning ron model, can be used to realize many high-level cognitive appli- method by IBM [11]. Let’s assume that McCulloch-Pitts neuron cations [11]. The operations of McCulloch-Pitts can be mathemat- model is adopted. The corresponding stochastic operation on ically formulated as: TrueNorth can be represented as follows: n−1 y'''=wx⋅−λ , and (3) ywx' = '' ∑i=0 ii, (5) ⎧1, reset y'= 0; if y'≥ 0 ' . (4) ⎪⎧ Pw()ii== c p i z ' = ⎨ , (6) ⎩ 0, reset y'= 0; if y'<0 ⎨ ' ⎩⎪Pw(0)1ii==− p Here, λ is a constant leak. Note that the spiking time is omitted pwciii= , and (7) in (3) and (4) because McCulloch-Pitts model is history-free. ' ⎪⎧ Px(1)ii== x . (8) The implementation of TrueNorth differs from traditional arti- ⎨ ' ficial neuron model in the following three aspects: First, the inputs ⎩⎪Px(0)1ii==− x (x') and the neuron output (z') are represented in a binary format Here, i is the index of an input. Equation (6) implies that the of spikes; second, the weights (w') are the integers selected from 4 synapses are connected by Bernoulli distributions with probability synaptic weights, and the selections are determined by the axon p = [p ] (i = 0, …, n-1). If the synaptic connection is ON, an inte- type at the corresponding neurons [9]; finally, a digital and recon- i ger c is assigned as its weight; otherwise, the synaptic weight (of figurable LIF model is used to represent the neuron function. i an OFF connection) is set to 0. Equation (7) assures that the ex- Such a digitized quantization operation inevitably results in sys- pectation of the synaptic weight in TrueNorth E{wi'} is equivalent tem accuracy degradation. TrueNorth attempts to compensate this to the weight of neural network wi represented by a real number. loss by introducing stochastic computation processes in temporal Similarly, equation (8) assures the expectation of input spikes is xi and spatial domains [9][11]: first, several neural codes are sup- (0 ≤ xi ≤ 1), which corresponds to the normalized intensity (e.g. ported and used to provide a series of input spikes that follow the pixel value) of the ith input. Note that we omit the bias b because it probabilities of the normalized input value (e.g., pixel) intensities; can be included in wx⋅ by adding an additional connection with second, the PRNG in LIF controls the synapse connectivity and weight b and a constant input 1. The same simplification can also makes the average value of the synaptic weight close to the one in be applied to the leak λ in Equation (3).
Details
-
File Typepdf
-
Upload Time-
-
Content LanguagesEnglish
-
Upload UserAnonymous/Not logged-in
-
File Pages6 Page
-
File Size-