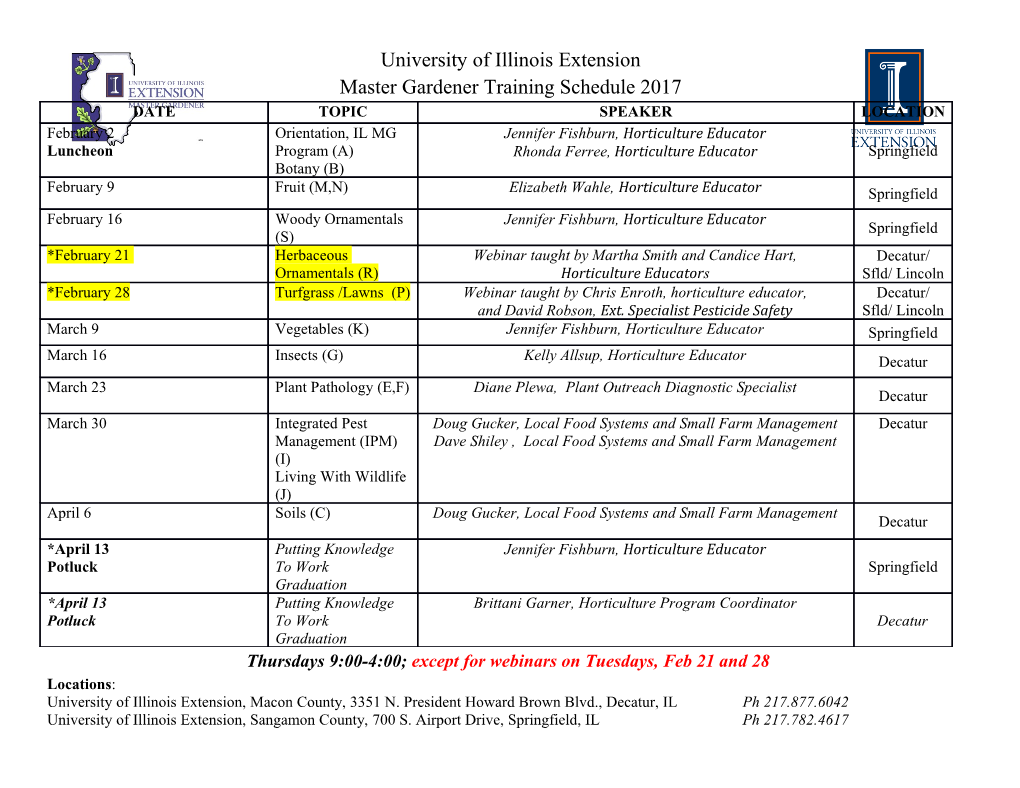
R. A. Fisher's Fiducial Argument and Bayes' Theorem Teddy Seidenfeld Statistical Science, Vol. 7, No. 3. (Aug., 1992), pp. 358-368. Stable URL: http://links.jstor.org/sici?sici=0883-4237%28199208%297%3A3%3C358%3ARAFFAA%3E2.0.CO%3B2-G Statistical Science is currently published by Institute of Mathematical Statistics. Your use of the JSTOR archive indicates your acceptance of JSTOR's Terms and Conditions of Use, available at http://www.jstor.org/about/terms.html. JSTOR's Terms and Conditions of Use provides, in part, that unless you have obtained prior permission, you may not download an entire issue of a journal or multiple copies of articles, and you may use content in the JSTOR archive only for your personal, non-commercial use. Please contact the publisher regarding any further use of this work. Publisher contact information may be obtained at http://www.jstor.org/journals/ims.html. Each copy of any part of a JSTOR transmission must contain the same copyright notice that appears on the screen or printed page of such transmission. The JSTOR Archive is a trusted digital repository providing for long-term preservation and access to leading academic journals and scholarly literature from around the world. The Archive is supported by libraries, scholarly societies, publishers, and foundations. It is an initiative of JSTOR, a not-for-profit organization with a mission to help the scholarly community take advantage of advances in technology. For more information regarding JSTOR, please contact [email protected]. http://www.jstor.org Tue Mar 4 10:35:54 2008 Statistical Science 1992, Vol. 7, No. 3, 358-368 R. A. Fisher's Fiducial Argument and Bayes' Theorem Teddy Seidenfeld 1. INTRODUCTION determine whether the goals Fisher set for fiducial probability are even mutually consistent. In celebration of the 100th anniversary of Fisher's A convenient starting place for our investigation is birth, I want to raise the subject of fiducial inference the distinction between direct and inverse inference (or for our reflection. Shortly after Fisher's death in 1962, direct and inverse probability). These terms date back my teacher and friend, Henry Kyburg, addressed a at least as far as Venn and appear often in those conference on fiducial probability. I find it appropriate passages where Fisher attempts to explicate fiducial to begin with some of Kyburg's (1963) remarks: probability, for the reason I will give below. As a I am a logician and a philosopher; I have not first approximation, the difference between direct and studied statistics for very long, and so I still very inverse probability is the difference between condi- quickly get out of my depth in a discussion of the tional probability for a specific (observable) event D technicalities of statistical inference. But I think given a statistical hypothesis S, p(DIS),and conditional it is important, none the less, for people whose probability of a statistical hypothesis given the evi- interests lie in the area of inference as such to do dence of sample data, p(SlD).For example, an instance the best they can in reacting to -and in having an of a direct probability statement is, "The probability action upon -current work in that particular kind is 0.5 of 'heads' on the next flip of this coin, given that of inference called "statistical." That this interac- it is a fair coin." Here the conditioning proposition may tion is difficult for both parties is the more reason be understood as supplying the statistical hypothesis for attempting it. (p. 938) Sthat there is a binomial model (a hypotheticalpopula- tion) for flips with this coin, 0 = 0.5, and the event D is My purpose in this essay is to try to assist that interac- that the next flip lands "heads." An inverse probability tion by focusing on the rather vague inference pattern statement is, "The probability is 0.4 that the coin is known as the "fiducial argument." I hope to show that, fair, given that 4 of 7 flips land 'heads'." Direct and though it really is untenable as a logical argument, inverse inference, then, denote those principles of in- nonetheless, it illuminates several key foundational ductive logic which determine or, at least, constrain the issues for understanding Fisher's disputes over the probability values of the direct and inverse probability status of Bayes' theorem and thereby some of the statements. They would explain the probability values continuing debates on the differences between so-called 0.5 and 0.4, assuming those values are inductively orthodox and Bayesian statistics. valid. Begin with the frank question: What is fiducial prob- A maxim for direct inference which is commonplace, ability? The difficulty in answering simply is that there in so far as any inductive rule can be so described, are too many responses to choose from. As is well is what Hacking (1965, p. 165) labels the Frequency known, Fisher's style was to offer heuristic examples Principle. It states loosely that, regarding the direct of fiducial arguments and, too quickly, to propose probability p(DIS), provided all one knows (of rele- (different) formal rules to generalize the examples. By vance) about the event D is that it is an instance of the contrast, among those who have attempted to recon- statistical "law" S, the direct conditional probability for struct fiducial inference according to a well-expressed event D is the value specified in the statistical law S. theory, one must single out the following five, in chro- If all we know about the next flip of this coin is that nological order: Jeffreys (1961), Fraser (1961), Demp- it is a flip of a fair coin, then the direct probability is ster (1963), Kyburg (1963, 1974) and Hacking (1965). 0.5 that it lands "heads" on the next flip. So, instead of beginning with a formal definition, let To this extent, direct probability is less problematic us try to find out what fiducial inference is supposed than inverse probability. There is no counterpart to to accomplish and then see whether, in fact, any answer the Frequency Principle for inverse probability. At to our question can be accepted. That is, first we shall best, p(S1D) may be determined by appeal- - to Bayes' theorem, p(SID) a p(DIS)p(S): an inverse inference Teddy Seidenfeld is Professor in the Departments of which involves both a direct probability p(D1S) and a Philosophy and Statistics, Carnegie Mellon University, prior probability p(S). It is here that Fisher sought Pittsburgh, Pennsylvania 15213. relief from inverse inference through fiducial probabil- FISHER'S FIDUCIAL ARGUMENT 359 ity for, as we will see in the next section, his idea ple is not accepted as evidence, this analysis does not was to reduce inverse to direct probability by fiducial invalidate the unconditional confidence level state- inference. Thus, we have to explore the limits of direct ment. The Neyman-Pearson direct inference about the inference in order to appreciate fiducial probability. confidence level is pegged to the reference set of re- Of course, rarely do we find our background informa- peated trials through a (pretrial) constraint on which tion so conveniently arranged as posited by the Fre- features of the statistical sample count as evidence. quency Principle. I may know where the coin was Whereas the Neyman-Pearson resolution to direct minted, or who flipped it last, in addition to knowing inference is grounded on a pragmatic choice of eviden- it is fair. Are these relevant considerations about the tial considerations, Fisher offers an epistemological next flip, given the statistical hypothesis (S)that the criterion. His criterion (reported below) is a balance coin is fair? I may know that the next flip of this coin of what we know and what we don't know. Fisher is an instance of a different statistical law, S', as well incorporates his views about direct inference into what an instance of S. It may be a flip of a coin drawn from he calls a semantics for probability. The following is a urn U (0 = 0.6), where half the coins in U are fair and succinct expression both of Fisher's dissatisfaction the other half biased (0 = 0.7) for "heads." By the with the limiting frequency interpretation of probabil- Frequency Principle, if all I know about the next flip ity and a sketch of his positive theory. is that it is an instance of St, the direct probability that it lands "heads" on the next flip is 0.6, p(DIS') = Remark 0.6. What if I know that the next flip is an instance of In the quotation below, Fisher alludes to an aggre- both laws S and St? (H. Reichenbach, an advocate of a gate or hypotheticalpopulationof, for example, throws limiting frequency interpretation of probability, called of a fair die to suggest what the binomial magnitude this the problem of the reference class for single 0 = 116 is about; to wit, one-sixth of the hypothetical events.) population of throws are "aces." I find Fisher's talk of Invariably, these difficulties are resolved by some hypothetical populations of value only as a metaphor. version of a Total Evidence principle. However, com- It facilitates the additional language of subpopulations peting versions do not lead to the same results. For and relevant reference sets, which are helpful in fram- instance, the usual account of Neyman-Pearson statis- ing the real problems with direct inference. In a differ- tical tests is in terms of direct probabilities of type 1 ent setting- statistical estimation -Fisher (1973) uses and type 2 errors. Likewise, the usual interpretation the concept of a hypothetical population to justify his of confidence levels is as direct probabilities that the criterion of Fisher Consistency (see Seidenfeld, 1992).
Details
-
File Typepdf
-
Upload Time-
-
Content LanguagesEnglish
-
Upload UserAnonymous/Not logged-in
-
File Pages14 Page
-
File Size-