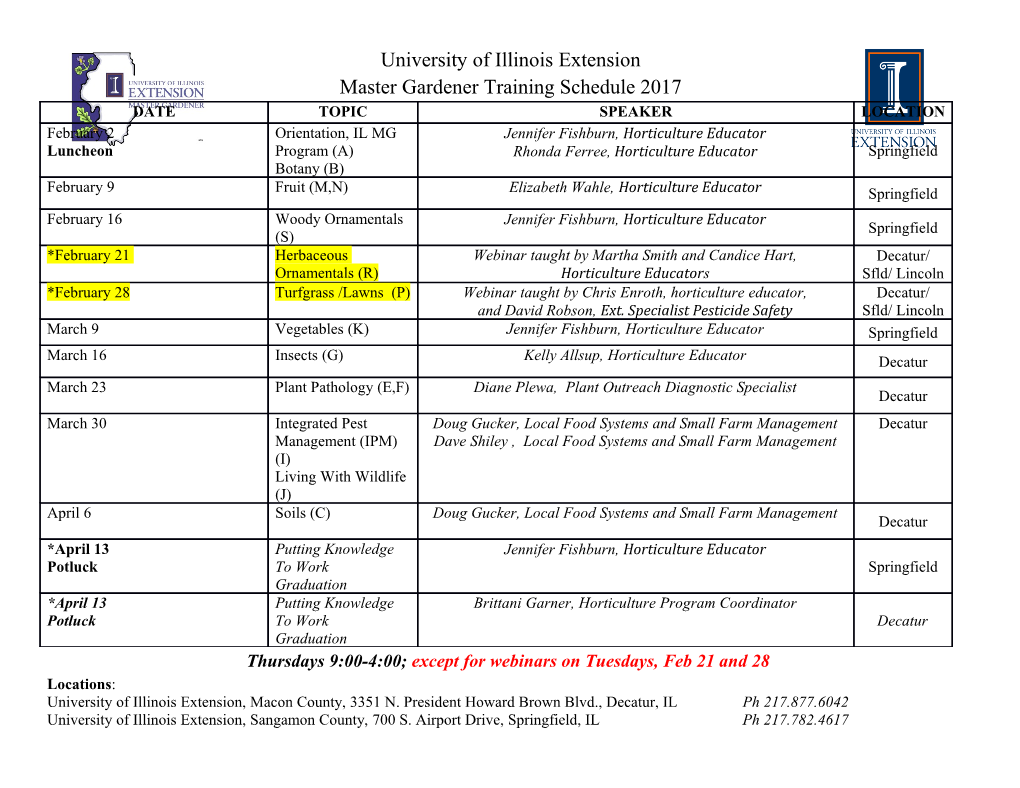
University of Nebraska at Omaha DigitalCommons@UNO Mathematics Faculty Publications Department of Mathematics 8-2019 Two dimensional search algorithms for linear programming Fabio Torres Vitor Follow this and additional works at: https://digitalcommons.unomaha.edu/mathfacpub Part of the Mathematics Commons Two dimensional search algorithms for linear programming by Fabio Torres Vitor B.S., Maua Institute of Technology, Brazil, 2013 M.S., Kansas State University, 2015 AN ABSTRACT OF A DISSERTATION submitted in partial fulfillment of the requirements for the degree DOCTOR OF PHILOSOPHY Department of Industrial and Manufacturing Systems Engineering Carl R. Ice College of Engineering KANSAS STATE UNIVERSITY Manhattan, Kansas 2019 Abstract Linear programming is one of the most important classes of optimization problems. These mathematical models have been used by academics and practitioners to solve numerous real world applications. Quickly solving linear programs impacts decision makers from both the public and private sectors. Substantial research has been performed to solve this class of problems faster, and the vast majority of the solution techniques can be categorized as one dimensional search algorithms. That is, these methods successively move from one solution to another solution by solving a one dimensional subspace linear program at each iteration. This dissertation proposes novel algorithms that move between solutions by repeatedly solving a two dimensional subspace linear program. Computational experiments demonstrate the potential of these newly developed algorithms and show an average improvement of nearly 25% in solution time when compared to the corresponding one dimensional search version. This dissertation's research creates the core concept of these two dimensional search algorithms, which is a fast technique to determine an optimal basis and an optimal solution to linear programs with only two variables. This method, called the slope algorithm, compares the slope formed by the objective function with the slope formed by each constraint to determine a pair of constraints that intersect at an optimal basis and an optimal solution. The slope algorithm is implemented within a simplex framework to perform two dimen- sional searches. This results in the double pivot simplex method. Differently than the well-known simplex method, the double pivot simplex method simultaneously pivots up to two basic variables with two nonbasic variables at each iteration. The theoretical compu- tational complexity of the double pivot simplex method is identical to the simplex method. Computational results show that this new algorithm reduces the number of pivots to solve benchmark instances by approximately 40% when compared to the classical implementation of the simplex method, and 20% when compared to the primal simplex implementation of CPLEX, a high performance mathematical programming solver. Solution times of some random linear programs are also improved by nearly 25% on average. This dissertation also presents a novel technique, called the ratio algorithm, to find an optimal basis and an optimal solution to linear programs with only two constraints. When the ratio algorithm is implemented within a simplex framework to perform two dimensional searches, it results in the double pivot dual simplex method. In this case, the double pivot dual simplex method behaves similarly to the dual simplex method, but two variables are exchanged at every step. Two dimensional searches are also implemented within an interior point framework. This dissertation creates a set of four two dimensional search interior point algorithms derived from primal and dual affine scaling and logarithmic barrier search directions. Each iteration of these techniques quickly solves a two dimensional subspace linear program formed by the intersection of two search directions and the feasible region of the linear program. Search directions are derived by orthogonally partitioning the objective function vector, which allows these novel methods to improve the objective function value at each step by at least as much as the corresponding one dimensional search version. Computational experiments performed on benchmark linear programs demonstrate that these two dimensional search interior point algorithms improve the average solution time by approximately 12% and the average number of iterations by 15%. In conclusion, this dissertation provides a change of paradigm in linear programming optimization algorithms. Implementing two dimensional searches within both a simplex and interior point framework typically reduces the computational time and number of iterations to solve linear programs. Furthermore, this dissertation sets the stage for future research topics in multidimensional search algorithms to solve not only linear programs but also other critical classes of optimization methods. Consequently, this dissertation's research can become one of the first steps to change how commercial and open source mathematical programming software will solve optimization problems. Two dimensional search algorithms for linear programming by Fabio Torres Vitor B.S., Maua Institute of Technology, Brazil, 2013 M.S., Kansas State University, 2015 A DISSERTATION submitted in partial fulfillment of the requirements for the degree DOCTOR OF PHILOSOPHY Department of Industrial and Manufacturing Systems Engineering Carl R. Ice College of Engineering KANSAS STATE UNIVERSITY Manhattan, Kansas 2019 Approved by: Major Professor Todd Easton Copyright © Fabio Torres Vitor 2019. Abstract Linear programming is one of the most important classes of optimization problems. These mathematical models have been used by academics and practitioners to solve numerous real world applications. Quickly solving linear programs impacts decision makers from both the public and private sectors. Substantial research has been performed to solve this class of problems faster, and the vast majority of the solution techniques can be categorized as one dimensional search algorithms. That is, these methods successively move from one solution to another solution by solving a one dimensional subspace linear program at each iteration. This dissertation proposes novel algorithms that move between solutions by repeatedly solving a two dimensional subspace linear program. Computational experiments demonstrate the potential of these newly developed algorithms and show an average improvement of nearly 25% in solution time when compared to the corresponding one dimensional search version. This dissertation's research creates the core concept of these two dimensional search algorithms, which is a fast technique to determine an optimal basis and an optimal solution to linear programs with only two variables. This method, called the slope algorithm, compares the slope formed by the objective function with the slope formed by each constraint to determine a pair of constraints that intersect at an optimal basis and an optimal solution. The slope algorithm is implemented within a simplex framework to perform two dimen- sional searches. This results in the double pivot simplex method. Differently than the well-known simplex method, the double pivot simplex method simultaneously pivots up to two basic variables with two nonbasic variables at each iteration. The theoretical compu- tational complexity of the double pivot simplex method is identical to the simplex method. Computational results show that this new algorithm reduces the number of pivots to solve benchmark instances by approximately 40% when compared to the classical implementation of the simplex method, and 20% when compared to the primal simplex implementation of CPLEX, a high performance mathematical programming solver. Solution times of some random linear programs are also improved by nearly 25% on average. This dissertation also presents a novel technique, called the ratio algorithm, to find an optimal basis and an optimal solution to linear programs with only two constraints. When the ratio algorithm is implemented within a simplex framework to perform two dimensional searches, it results in the double pivot dual simplex method. In this case, the double pivot dual simplex method behaves similarly to the dual simplex method, but two variables are exchanged at every step. Two dimensional searches are also implemented within an interior point framework. This dissertation creates a set of four two dimensional search interior point algorithms derived from primal and dual affine scaling and logarithmic barrier search directions. Each iteration of these techniques quickly solves a two dimensional subspace linear program formed by the intersection of two search directions and the feasible region of the linear program. Search directions are derived by orthogonally partitioning the objective function vector, which allows these novel methods to improve the objective function value at each step by at least as much as the corresponding one dimensional search version. Computational experiments performed on benchmark linear programs demonstrate that these two dimensional search interior point algorithms improve the average solution time by approximately 12% and the average number of iterations by 15%. In conclusion, this dissertation provides a change of paradigm in linear programming optimization algorithms. Implementing two dimensional searches within both a simplex and interior point framework typically reduces the computational time and number of iterations to solve linear programs. Furthermore,
Details
-
File Typepdf
-
Upload Time-
-
Content LanguagesEnglish
-
Upload UserAnonymous/Not logged-in
-
File Pages170 Page
-
File Size-