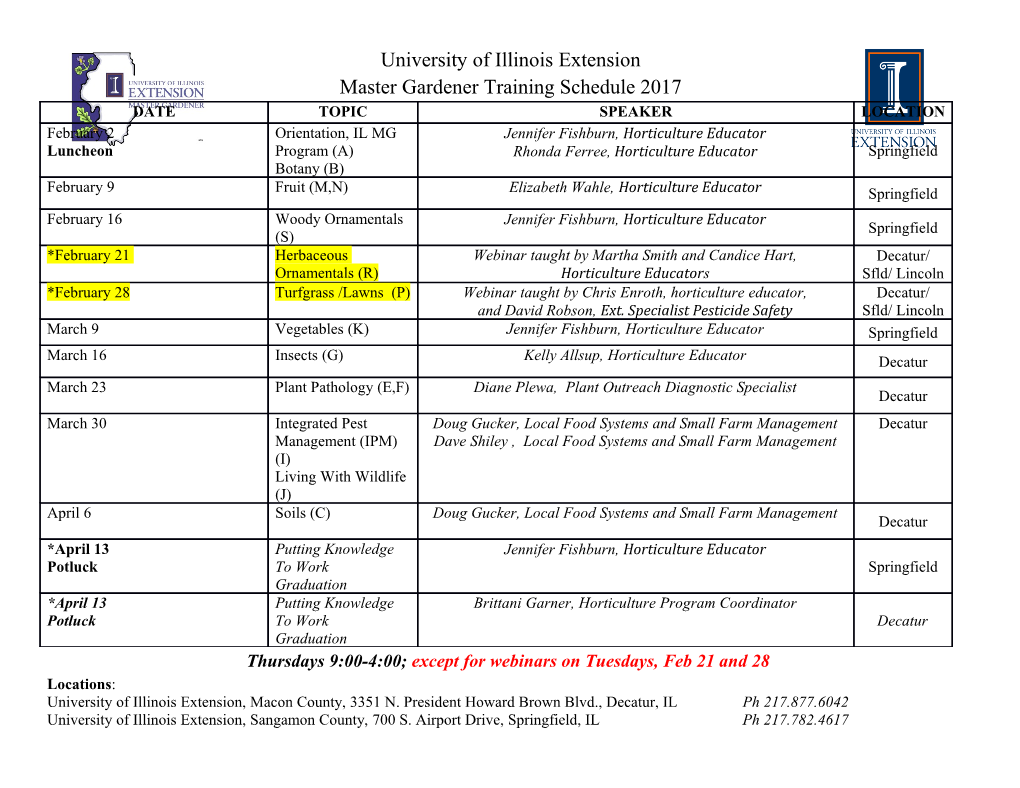
Genomic Science Contractors–Grantees Meeting XIV and USDA‐DOE Plant Feedstock Genomics for Bioenergy Meeting, March 6-9, 2016 -- http://genomicscience.energy.gov/pubs/2016abstracts/ Development of a genome-scale Escherichia coli kinetic metabolic model satisfying flux data for multiple mutant strains Ali Khodayari ([email protected]) and Costas D. Maranas Department of Chemical Engineering, Pennsylvania State University, University Park, Pennsylvania, USA. http://www.maranasgroup.com Project Goals: The goal of this effort is to construct a genome-scale kinetic model of Escherichia coli metabolism by making use of Ensemble Modeling (EM) concepts. Model parameterization is carried out using multiple flux datasets for different substrates and growth (aerobic vs. anaerobic) conditions. Kinetic modeling paradigm provides a promising platform to broaden our knowledge of cellular capacity and cell physiology beyond stoichiometric descriptions. However, developing kinetic models of metabolism at a genome-scale that faithfully recapitulate the effect of multiple genetic interventions is still an ongoing challenge. To this end, we introduce k-ecoli457, a genome-scale kinetic model of Escherichia coli metabolism that satisfies fluxomic data for a wild-type and 25 mutant strains for different substrates and growth (aerobic vs. anaerobic) conditions. The k-ecoli457 model contains 457 reactions and 337 metabolites accounting for all relevant reactions from the genome-scale iAF1260 model that carry flux under the experimental conditions of the flux measurements. These include reactions in glycolysis/gluconeogenesis, the Pentose Phosphate (PP) pathway, the TriCarboxylic Acid (TCA) cycle, anaplerotic reactions, amino acid synthesis/degradation, fatty acid oxidation/synthesis and a number of reactions in other parts of the metabolism, such as folate metabolism, cofactor and prosthetic group synthesis, alternative carbon, membrane lipid, cell envelope, nucleotide salvage and oxidative phosphorylation pathways. In addition, 295 regulatory interactions were extracted from BRENDA and EcoCyc and included in k- ecoli457. The model was also supplemented with a simplified version of the biomass equation including all the constituent precursors. Model predictions were tested against multiple experimentally measured datasets that were not used during model parameterization. These included (i) 898 steady-state metabolite concentrations for twenty of the mutant strains [1-4], (ii) 319 Michaelis-Menten constants (211 Km and 108 kcat values) from BRENDA and EcoCyc, and (iii) 320 experimentally reported product yields for designed strains spanning 24 different bioproducts. Comparisons revealed that 63% of the predicted metabolite concentrations as well as 60% and 64% of the estimated Km and kcat values, respectively, are within the experimentally reported ranges. These levels of agreement, in overall, exceed the previous effort [5], despite the significantly increased scope of the model and coverage of less studied pathways. The average relative error of k- ecoli457 predictions for the yield of 16 bioproducts in 140 designed strains is 0.1, while stoichiometric model based techniques such as flux balance analysis (FBA) or minimization of metabolic adjustment (MOMA) yield corresponding relative errors of 1.05 and 1.19, respectively. This modeling effort describes a stepwise procedure for construction of genome-scale kinetic models with robust parameterization consistent with multiple sets of omics information for E. coli and provides guidelines for developing genome-scale kinetic models for other well-studied organisms. The work was supported by the genomic science grant from Department of Energy, USA (grant# DE-SC0012377). 1. Ishii N, Nakahigashi K, Baba T, Robert M, Soga T, Kanai A, Hirasawa T, Naba M, Hirai K, Hoque A et al: Multiple high- throughput analyses monitor the response of E. coli to perturbations. Science 2007, 316(5824):593-597. Genomic Science Contractors–Grantees Meeting XIV and USDA‐DOE Plant Feedstock Genomics for Bioenergy Meeting, March 6-9, 2016 -- http://genomicscience.energy.gov/pubs/2016abstracts/ 2. Kabir MM, Ho PY, Shimizu K: Effect of ldhA gene deletion on the metabolism of Escherichia coli based on gene expression, enzyme activities, intracellular metabolite concentrations, and metabolic flux distribution. Biochem Eng J 2005, 26(1):1-11. 3. Zhao J, Baba T, Mori H, Shimizu K: Global metabolic response of Escherichia coli to gnd or zwf gene-knockout, based on 13C-labeling experiments and the measurement of enzyme activities. Applied microbiology and biotechnology 2004, 64(1):91-98. 4. Zhao J, Shimizu K: Metabolic flux analysis of Escherichia coli K12 grown on 13C-labeled acetate and glucose using GC- MS and powerful flux calculation method. Journal of biotechnology 2003, 101(2):101-117. 5. Khodayari A, Zomorrodi AR, Liao JC, Maranas CD: A kinetic model of Escherichia coli core metabolism satisfying multiple sets of mutant flux data. Metabolic engineering 2014, 25:50-62. a) b) Glycerophospholipid Metabolism EX_glc(e) Folate Metabolism Unassigned glc-D g3p Nucleotide Salvage Pathway HEX1 pep Cofactor and Prosthetic Group Biosynthesis ATP Cysteine Metabolism pts pyr EDA 2ddg6p ADP Methionine Metabolism EDD Oxidative Phosphorylation ATP g6p g1p NADP NADPH NADP NADPH ADPGLCt GLGC PGMT ru5p RU5Pt Methylglyoxal Metabolism Glutamate metabolism adpglc pep pyr PGI G6PDH2r 6PGL 6PGC GND FRUpts2 PGL Transport, Outer Membrane Porin RPE FORt f6p F6Pt RPI Anaplerotic Reactions fru r5p R5PP ATPPRT Pyruvate Metabolism x5p Histidine ATP ATP Glycine and Serine Metabolism FBP PFKB rib-D Purine ADP TKT1 ADP PFKA TKT2 Alanine and Aspartate Metabolism s7p S7Pt Histidine Metabolism fdp g3p Alternate Carbon Metabolism TALA FBA Citric Acid Cycle g3p E4Pt Sub-system Transport reaction DHAPt G3Pt e4p f6p dhap TPI Pentose Phosphate Pathway NAD GAPD Tryptophan Purine and Pyrimidine Biosynthesis NADH DDPA DHQS Phenylalanine Valine, Leucine, and Isoleucine Metabolism 13dpg 2dda7p PGK ADP Tyrosin Threonine and Lysine Metabolism Serine ATP Glycolysis/Gluconeogenesis PGCD 3pg 3PGt Glycine Tyrosine, Tryptophan, and Phenylalanine Metabolism Cysteine PGM Transport, Outer Membrane Porin and inner membrane h[c] h[e] Arginine and Proline Metabolism 2pg Lac-D Transport, Inner Membrane ENO D_LACt2 Membrane Lipid Metabolism PEPt pep LDH_D PPS NAD ACALDt Cell Envelope Biosynthesis ADP ADP ATP NADH NAD PYKf ADP NADH ACALD ETOHt2r 0 10 20 30 40 50 60 70 80 PYKA coa ATP ATP NAD Alanine NADH ALCD2x etoh accoa acald h[c] h[e] c) # of reactions Valine PDH Nucleotide Salvage Pathway ACLS pyr coa PTAr Leucine NAD NADH ADP ATP Transport, Outer Membrane Porin coa coa AC ACt2r Isoleucine ACCOAC/ Methionine Metabolism PFL KAS15 FORti FOR ACKr actp h[c] h[e] Alternate Carbon Metabolism PPCK Asparagine pep h[e] Valine, Leucine, and Isoleucine Metabolism FORt2 Fatty acid ACS Methionine ADP h[c] Membrane Lipid Metabolism ATP Threonine PPC oaa CS Cell Envelope Biosynthesis ASPK cit Lysine Aspartate Purine and Pyrimidine Biosynthesis ASPTA OAAt coa QH2 NADH ACONTa Histidine Metabolism MDH2 h[c]h[e] MDH ME2 NAD Q Pyruvate Metabolism pyr NADPH NADP glx acon-C Cysteine Metabolism PYRT2R NADH NAD Proline ME1 mal-L MALS ACONTb Pentose Phosphate Pathway PYRt coa accoa Arginine Alanine and Aspartate Metabolism MALt2_2 FUM ICL h[c] icit ACGS/ Sub-system Citric Acid Cycle h[e] h[c] GLU5K GLUN h[e] h[e] FRD2 QH2 Tyrosine, Tryptophan, and Phenylalanine Metabolism ICDHyr NADP GLUt2r Glycine and Serine Metabolism FUMt2_2 fum Q h[c] h[c]h[e] NADPH ATP ADP gln-L SUCDi glu-L Anaplerotic Reactions 2h[e] 2h[c] QH2 Q AKGt2r GLUDy GLNS Threonine and Lysine Metabolism akg NADPH NADP NADP SACOAS succoa Glycolysis/Gluconeogenesis NADPH SUCCt3 succ coa GLUSy NAD h[e] coa Glutamate metabolism NADH SUCCt2_2 ATP ADP AKGDH Arginine and Proline Metabolism h[c] SUCCOAt 0 5 10 15 20 25 30 35 40 # of substrate-level regulations Figure 1 (a) A pictorial representation of the constructed kinetic model of E. coli metabolism. Red, brown and green marks denote the knockout mutants grown aerobically on glucose, anaerobically on glucose and aerobically on pyruvate, respectively, with flux data for the reactions shown in blue. (b) Sub-system classification of reactions in the constructed kinetic model. (c) Sub-system classification of the integrated regulatory interactions. Blue bars denote the content of the core model [5] while red denotes the additional reactions/regulations included in k-ecoli457. .
Details
-
File Typepdf
-
Upload Time-
-
Content LanguagesEnglish
-
Upload UserAnonymous/Not logged-in
-
File Pages2 Page
-
File Size-