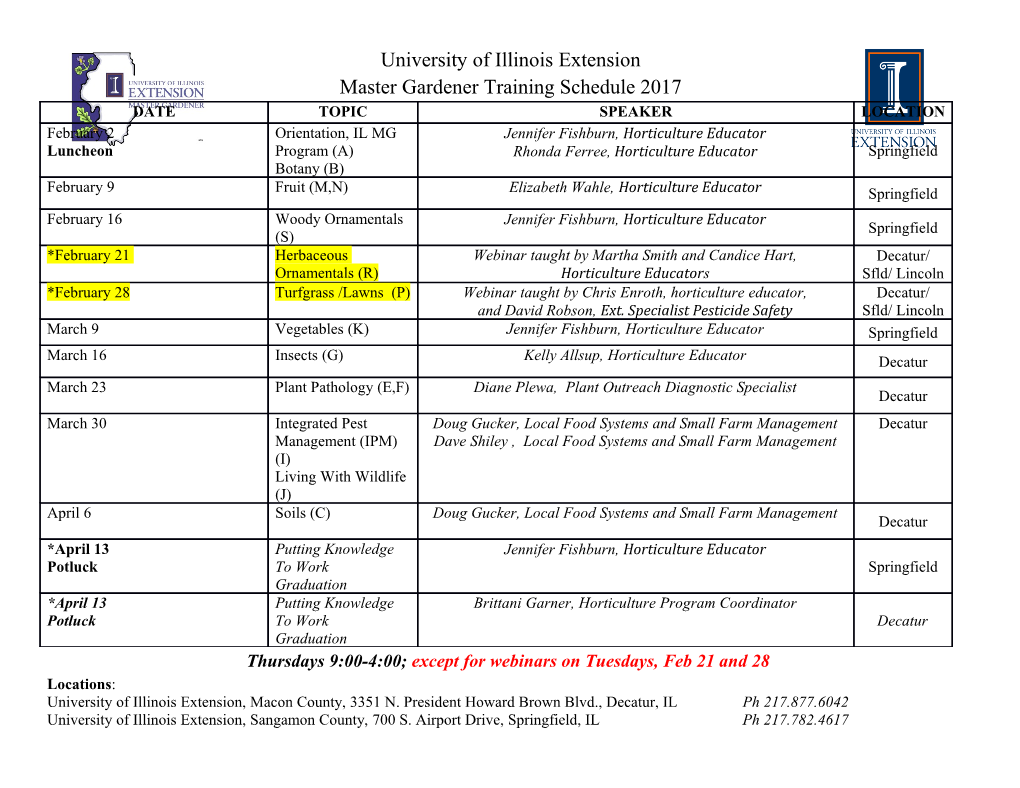
An introduction to Quantum Monte Carlo Jose Guilherme Vilhena Laboratoire de Physique de la Matière Condensée et Nanostructures, Université Claude Bernard Lyon 1 Outline 1) Quantum many body problem; 2) Metropolis Algorithm; 3) Statistical foundations of Monte Carlo methods; 4) Quantum Monte Carlo methods ; 4.1) Overview; 4.2) Variational Quantum Monte Carlo; 4.3) Diffusion Quantum Monte Carlo; 4.4) Typical QMC calculation; Outline 1) Quantum many body problem; 2) Metropolis Algorithm; 3) Statistical foundations of Monte Carlo methods; 4) Quantum Monte Carlo methods ; 4.1) Overview; 4.2) Variational Quantum Monte Carlo; 4.3) Diffusion Quantum Monte Carlo; 4.4) Typical QMC calculation; 1 - Quantum many body problem Consider of N electrons. Since m <<M , to a good e N approximation, the electronic dynamics is governed by : Z Z 1 2 1 H =− ∑ ∇ i −∑∑ ∑∑ 2 i i ∣r i−d∣ 2 i j ≠i ∣ri−r j∣ which is the Born Oppenheimer Hamiltonian. We want to find the eigenvalues and eigenvectos of this hamiltonian, i.e. : H r1, r 2,. ..,r N =E r 1, r2,. ..,r N QMC allow us to solve numerically this, thus providing E0 and Ψ . 0 1 - Quantum many body problem Consider of N electrons. Since m <<M , to a good e N approximation, the elotonic dynamics is governed by : Z Z 1 2 1 H =− ∑ ∇ i −∑∑ ∑∑ 2 i i ∣r i−d∣ 2 i j ≠i ∣ri−r j∣ which is the Born Oppenheimer Hamiltonian. We want to find the eigenvalues and eigenvectos of this hamiltonian, i.e. : H r1, r 2,. ..,r N =E r 1, r2,. ..,r N QMC allow us to solve numerically this, thus providing E0 and Ψ . 0 1 - Quantum many body problem Varionational principle For any normalized wfn Ψ: 〈T R∣H∣T R〉E0 Zero Varience Property The variance of the “local energy” must vanish for Ψ =Ψ , T 0 2 2 ∣T∣ 2 H T R i.e. E =∫ EL−〈 EL〉 =0 ;where EL= L 2 R ∫ d R∣T∣ T Example : Harmonic Oscilator H T x E L x= σ (x) T x EL then... ∂ x E L x =0 ;if T =0 ≠ ≠ 0 ;if T 0 1 - Quantum many body problem Varionational principle For any normalized wfn Ψ: 〈T R∣H∣T R〉E0 Zero Varience Property The variance of the “local energy” must vanish for Ψ =Ψ , T 0 2 2 ∣T∣ 2 H T R i.e. E =∫ EL−〈 EL〉 =0 ;where EL= L 2 R ∫ d R∣T∣ T Example : Harmonic Oscilator H T x E L x= σ (x) T x EL then... ∂ x E L x =0 ;if T =0 ≠ ≠ 0 ;if T 0 1 - Quantum many body problem Varionational principle For any normalized wfn Ψ: 〈T R∣H∣T R〉E0 Zero Varience Property The variance of the “local energy” must vanish for Ψ =Ψ , T 0 2 2 ∣T∣ 2 H T R i.e. E =∫ EL−〈 EL〉 =0 ;where EL= L 2 R ∫ d R∣T∣ T Example : Harmonic Oscilator H T x E L x= σ (x) T x EL then... ∂ x E L x =0 ;if T =0 ≠ ≠ 0 ;if T 0 Outline 1) Quantum many body problem; 2) Metropolis Algorithm; 3) Statistical foundations of Monte Carlo methods; 4) Quantum Monte Carlo methods ; 4.1) Overview; 4.2) Variational Quantum Monte Carlo; 4.3) Diffusion Quantum Monte Carlo; 4.4) Typical QMC calculation 2 - Metropolis Algorithm Markov Chain stocastic process (no memory). Metropolis-Hastings algorith (Markov chain) randomly samples a “problematic” probability distribuition function; Some definitions ... > P(R): normalized probability distribuition function > R=(r ,..., r ) : configuration or walker (can be vector with 1 N the positions of all electrons) > T(R'←R): Proposal density (can be 1, a gaussian, ...) > q=rand(0,1): random number in [0,1] 2 - Metropolis Algorithm We seek a set {R , R , ..., R } of sample points of P(R). 1 2 M How can we get them ?!? Metropolis Algorithm: 1) Start in a point Rt ; 2) Propose a point R', with a probability of T(R'←R) ; T R' R PR A R' R= 3) Calculate the “acceptance rate” : T R R' PR ' 4) if A > 1 then : Rt+1=R' else if A > q then : Rt+1=R' else : Rt+1=Rt 5) Repeat all from setp 2; 6) stop at k>M , and take away the first k-M points. The sampled points match P(R). The mixing is controled by T( R'←R). 2 - Metropolis Algorithm We seek a set {R , R , ..., R } of sample points of P(R). 1 2 M How can we get them ?!? Metropolis Algorithm: 1) Start in a point Rt ; 2) Propose a point R', with a probability of T(R'←R) ; T R' R PR A R' R= 3) Calculate the “acceptance rate” : T R R' PR ' 4) if A > 1 then : Rt+1=R' else if A > q then : Rt+1=R' else : Rt+1=Rt 5) Repeat all from setp 2; 6) stop at k>M , and take away the first k-M points. The sampled points match P(R). The mixing is controled by T( R'←R). 2 - Metropolis Algorithm We seek a set {R , R , ..., R } of sample points of P(R). 1 2 M How can we get them ?!? Metropolis Algorithm: 1) Start in a point Rt ; 2) Propose a point R', with a probability of T(R'←R) ; T R' R PR A R' R= 3) Calculate the “acceptance rate” : T R R' PR ' 4) if A > 1 then : Rt+1=R' else if A > q then : Rt+1=R' else : Rt+1=Rt 5) Repeat all from setp 2; 6) stop at k>M , and take away the first k-M points. The sampled points match P(R). The mixing is controled by T( R'←R). 2 - Metropolis Algorithm We seek a set {R , R , ..., R } of sample points of P(R). 1 2 M How can we get them ?!? Metropolis Algorithm: 1) Start in a point Rt ; 2) Propose a point R', with a probability of T(R'←R) ; T R' R PR A R' R= 3) Calculate the “acceptance rate” : T R R' PR ' 4) if A > 1 then : Rt+1=R' else if A > q then : Rt+1=R' else : Rt+1=Rt 5) Repeat all from setp 2; 6) stop at k>M , and take away the first k-M points. The sampled points match P(R). The mixing is controled by T( R'←R). 2 - Metropolis Algorithm We seek a set {R , R , ..., R } of sample points of P(R). 1 2 M How can we get them ?!? Metropolis Algorithm: 1) Start in a point Rt ; 2) Propose a point R', with a probability of T(R'←R) ; T R' R PR A R' R= 3) Calculate the “acceptance rate” : T R R' PR ' 4) if A > 1 then : Rt+1=R' else if A > q then : Rt+1=R' else : Rt+1=Rt 5) Repeat all from setp 2; 6) stop at k>M , and take away the first k-M points. The sampled points match P(R). The mixing is controled by T( R'←R). 2 - Metropolis Algorithm We seek a set {R , R , ..., R } of sample points of P(R). 1 2 M How can we get them ?!? Metropolis Algorithm: 1) Start in a point Rt ; 2) Propose a point R', with a probability of T(R'←R) ; T R' R PR A R' R= 3) Calculate the “acceptance rate” : T R R' PR ' 4) if A > 1 then : Rt+1=R' else if A > q then : Rt+1=R' else : Rt+1=Rt 5) Repeat all from setp 2; 6) stop at k>M , and take away the first k-M points. The sampled points match P(R). The mixing is controled by T( R'←R). 2 - Metropolis Algorithm We seek a set {R , R , ..., R } of sample points of P(R). 1 2 M How can we get them ?!? Metropolis Algorithm: 1) Start in a point Rt ; 2) Propose a point R', with a probability of T(R'←R) ; T R' R PR A R' R= 3) Calculate the “acceptance rate” : T R R' PR ' 4) if A > 1 then : Rt+1=R' else if A > q then : Rt+1=R' else : Rt+1=Rt 5) Repeat all from setp 2; 6) stop at k>M , and take away the first k-M points. The sampled points match P(R). The mixing is controled by T( R'←R). Outline 1) Quantum many body problem; 2) Metropolis Algorithm; 3) Statistical foundations of Monte Carlo methods; 4) Quantum Monte Carlo methods ; 4.1) Overview; 4.2) Variational Quantum Monte Carlo; 4.3) Diffusion Quantum Monte Carlo; 4.4) Typical QMC calculation 3 – Statistical foundations of MC methods Monte Carlo methods: solve problems using random numbers. Evaluate integrals: - expectation value of a random variable is just the integral over its probability distribution - generate a bunch of random numbers and average to get the integral; Simulate random processes with random walkers. Number of dimensions doesn’t matter. - Simpsons rule (error ~ O (M-4/d)) - Monte Carlo method (error ~ O (M-1/2)) 3 – Statistical foundations of MC methods Monte Carlo methods: solve problems using random numbers. Evaluate integrals: - expectation value of a random variable is just the integral over its probability distribution - generate a bunch of random numbers and average to get the integral; Simulate random processes with random walkers. Number of dimensions doesn’t matter. - Simpsons rule (error ~ O (M-4/d)) - Monte Carlo method (error ~ O (M-1/2)) 3 – Statistical foundations of MC methods Monte Carlo methods: solve problems using random numbers.
Details
-
File Typepdf
-
Upload Time-
-
Content LanguagesEnglish
-
Upload UserAnonymous/Not logged-in
-
File Pages59 Page
-
File Size-