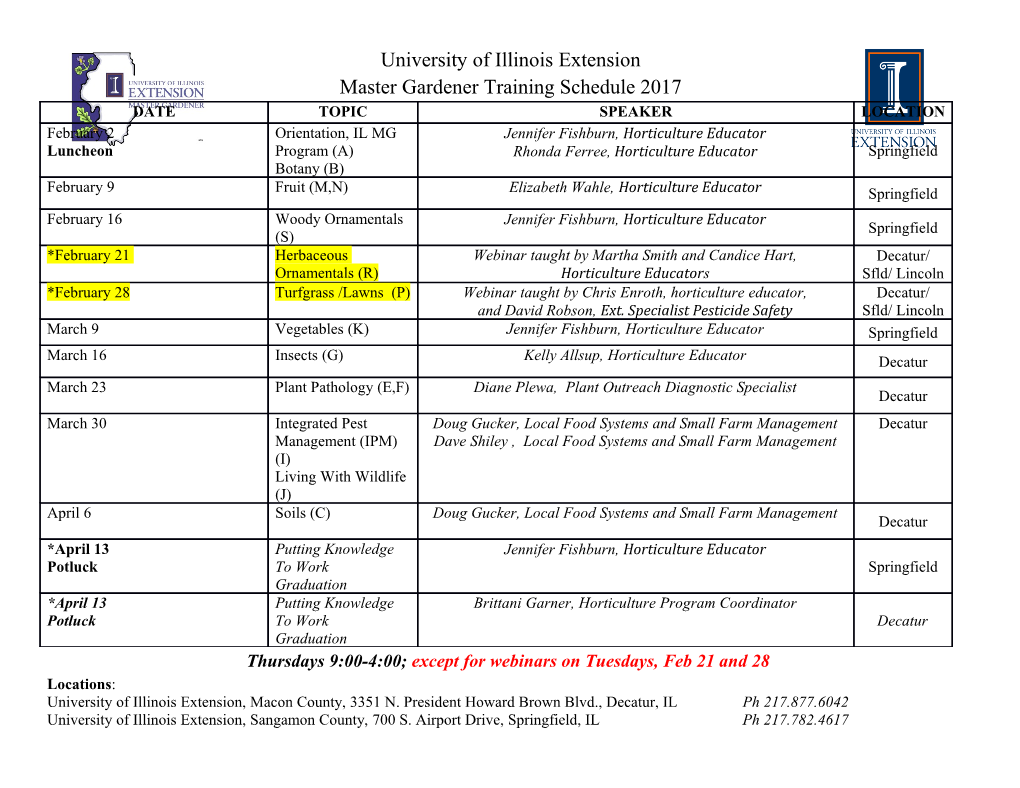
MapReduce: Simplified Data Processing on Large Clusters Jeffry Dean and Sanjay Ghemawat ODSI 2004 Presented by Anders Miltner Problem • When applied on to big data, simple algorithms beCome more complicated • Parallelized Computations • Distributed data • Failure handling • How Can we abstraCt away these Common diffiCulties, allowing the programmer to be able to write simple Code for their simple algorithms? Core Ideas • Require the programmer to state their problem in terms of two funCtions, map and reduCe • Handle the sCalability ConCerns inside the system, instead of requiring the programmer to handle them • Require the programmer to only write Code about the easy to understand functionality Map, Reduce, and MapReduce • map: (k1,v1) -> list (k2, v2) • reduCe: (k2, list v2) -> list (v2) • mapreduCe: list (k1,v1) -> list (k2, list v2) for a rewrite of our production indexing system. Sec- 2.2 Types tion 7 discusses related and future work. Even though the previous pseudo-code is written in terms of string inputs and outputs, conceptually the map and for a rewrite of our production indexing system.2 PrSec-ogramming2.2 TModelypes tion 7 discusses related and future work. reduce functions supplied by the user have associated types: The computationEvtakenesthougha set oftheinputpreviouskey/valuepseudo-codepairs, andis written in terms map (k1,v1) list(k2,v2) produces a setofofstringoutputinputskey/valueand pairs.outputs,Theconceptuallyuser of the map and → 2 Programming Model reduce (k2,list(v2)) list(v2) the MapReducereducelibraryfunctionsexpresses thesuppliedcomputationby theas twusero have associated → functions: Maptypes:and Reduce. I.e., the input keys and values are drawn from a different The computation takes a set of input key/value pairs, and domain than the output keys and values. Furthermore, Map, written by mapthe user, tak(k1,v1)es an input pair and pro- list(k2,v2) produces a set of output key/value pairs. The user of → the intermediate keys and values are from the same do- duces a set of intermediatereduce ke(k2,list(v2))y/value pairs. The MapRe- list(v2) the MapReduce library expresses the computationduceaslibrarytwo groups together all intermediate values asso-→ main as the output keys and values. functions: Map and Reduce. ciated with theI.e.,sametheintermediateinput keyskandey I vandaluespassesare drathemwn fromOura difC++ferentimplementation passes strings to and from Map, written by the user, takes an input pairtoandthepro-Reduce function.domain than the output keys and values. theFurthermore,user-defined functions and leaves it to the user code duces a set of intermediate key/value pairs. The MapRe-The Reduce thefunction,intermediatealso writtenkeysbyandthe vuseralues, acceptsare fromtotheconsamevert betweendo- strings and appropriate types. duce library groups together all intermediate valuesan intermediateasso- mainkey Iasandtheaoutputset of vkalueseys andfor vthatalues.key. It ciated with the same intermediate key I and passesmergesthemtogether theseOur C++valuesimplementationto form a possiblypassessmallerstrings to and from to the Reduce function. set of values. Ttheypicallyuser-definedjust zerofunctionsor one outputand leavaluevesisit to 2.3the userMorcodee Examples The Reduce function, also written by the userproduced, acceptsper Reduce invocation. The intermediate val- to convert between strings and appropriate Heretypes.are a few simple examples of interesting programs an intermediate key I and a set of values for thatueskareey. suppliedIt to the user’s reduce function via an iter- ator. This allows us to handle lists of values that are too that can be easily expressed as MapReduce computa- merges together these values to form a possibly smaller large to fit in memory. tions. set of values. Typically just zero or one output value is 2.3 More Examples produced per Reduce invocation. The intermediate val- ues are supplied to the user’s reduce function via2.1an iterExample- Here are a few simple examples of interestingDistribprogramsuted Grep: The map function emits a line if it that can be easily expressed as MapReducematchescomputa-a supplied pattern. The reduce function is an ator. This allows us to handle lists of values thatConsiderare toothe problem of counting the number of oc- identity function that just copies the supplied intermedi- large to fit in memory. currences of eachtions.word in a large collection of docu- ate data to the output. ments. The user would write code similar to the follow- ing pseudo-code: 2.1 Example Distributed Grep: The map function emits a line if it Example Count of URL Access Frequency: The map func- map(Stringmatcheskey, Stringa suppliedvalue):pattern. The reduce function is an Consider the problem of counting the number of oc- tion processes logs of web page requests and outputs // key:identitydocumentfunctionnamethat just copies the supplied intermedi- URL, 1 . The reduce function adds together all values currences of each word in a large collection of docu-// value: document contents ⟨ ⟩ • Word Count in a lot of doCuments ate data to the output. for the same URL and emits a URL, total count ments. The user would write code similar to the folloforw- each word w in value: ⟨ ⟩ ing pseudo-code: EmitIntermediate(w, "1"); pair. Count of URL Access Frequency: The map func- map(String key, String value): reduce(String key, Iterator values): tion processes logs of web page requests and outputs // key: document name // key: a word Reverse Web-Link Graph: The map function outputs URL, 1 . The reduce function adds togethertargetall values, source pairs for each link to a target // value: document contents // values:⟨ a list⟩ of counts ⟨ ⟩ int resultfor the= 0;same URL and emits a URL, totalURL countfound in a page named source. The reduce for each word w in value: ⟨ ⟩ EmitIntermediate(w, "1"); for eachpairv. in values: function concatenates the list of all source URLs as- result += ParseInt(v); sociated with a given target URL and emits the pair: Emit(AsString(result)); target, list(source) reduce(String key, Iterator values): ⟨ ⟩ Reverse Web-Link Graph: The map function outputs // key: a word The map function emits each word plus an associated // values: a list of counts target, source pairs for each link to a target count of occurrences⟨ (just ‘1’ in this⟩ simple example). Term-Vector per Host: A term vector summarizes the int result = 0; URL found in a page named source. The reduce The reduce function sums together all counts emitted most important words that occur in a document or a set for each v in values: function concatenates the list of all source URLs as- for a particular word. of documents as a list of word, frequency pairs. The result += ParseInt(v); ⟨ ⟩ In addition, thesociateduser writeswithcodea gitovenfilltaringeta maprURLeduceand emitsmap thefunctionpair: emits a hostname, term vector Emit(AsString(result)); target, list(source) ⟨ ⟩ specification object⟨ with the names of the input⟩ and out- pair for each input document (where the hostname is The map function emits each word plus an associatedput files, and optional tuning parameters. The user then extracted from the URL of the document). The re- invokes the MapReduce function, passing it the specifi- duce function is passed all per-document term vectors count of occurrences (just ‘1’ in this simple example). cation object. TheTerm-Vuser’sectorcodeperis linkHost:ed togetherA termwithvectorthe summarizesfor a giventhehost. It adds these term vectors together, The reduce function sums together all countsMapReduceemitted librarymost(implementedimportant wordsin C++).that Appendixoccur in aAdocumentthrowingor aawsetay infrequent terms, and then emits a final for a particular word. of documents as a list of word, frequency pairs. The contains the full program text for this example.⟨ hostname⟩ , term vector pair. In addition, the user writes code to fill in a mapreduce map function emits a hostname, term⟨ vector ⟩ ⟨ ⟩ specification object with the names of the input and out- pair for each input document (where the hostname is 2 put files, and optional tuning parameters. The userTo appearthen in eOSDIxtracted2004from the URL of the document). The re- invokes the MapReduce function, passing it the specifi- duce function is passed all per-document term vectors cation object. The user’s code is linked together with the for a given host. It adds these term vectors together, MapReduce library (implemented in C++). Appendix A throwing away infrequent terms, and then emits a final contains the full program text for this example. hostname, term vector pair. ⟨ ⟩ To appear in OSDI 2004 2 User Program (1) fork (1) fork (1) fork Master Architecture (2) (2) assign assign reduce map worker split 0 (6) write output split 1 worker (5) remote read file 0 • Large Clusters of Commodity PCs split 2 (3) read (4) local write worker output split 3 worker file 1 • Input files partitioned into M splits split 4 worker Input Map Intermediate files Reduce Output • Intermediate keys partitioned into R files phase (on local disks) phase files distributions Figure 1: Execution overview Inverted Index: The map function parses each docu- large clusters of commodity PCs connected together with ment, and emits a sequence of word, document ID switched Ethernet [4]. In our environment: • Written to disk, remotely read by ⟨ ⟩ pairs. The reduce function accepts all pairs for a given (1) Machines are typically dual-processor x86 processors word, sorts the corresponding document IDs and emits a running Linux, with 2-4 GB of memory per machine. word, list(document
Details
-
File Typepdf
-
Upload Time-
-
Content LanguagesEnglish
-
Upload UserAnonymous/Not logged-in
-
File Pages16 Page
-
File Size-