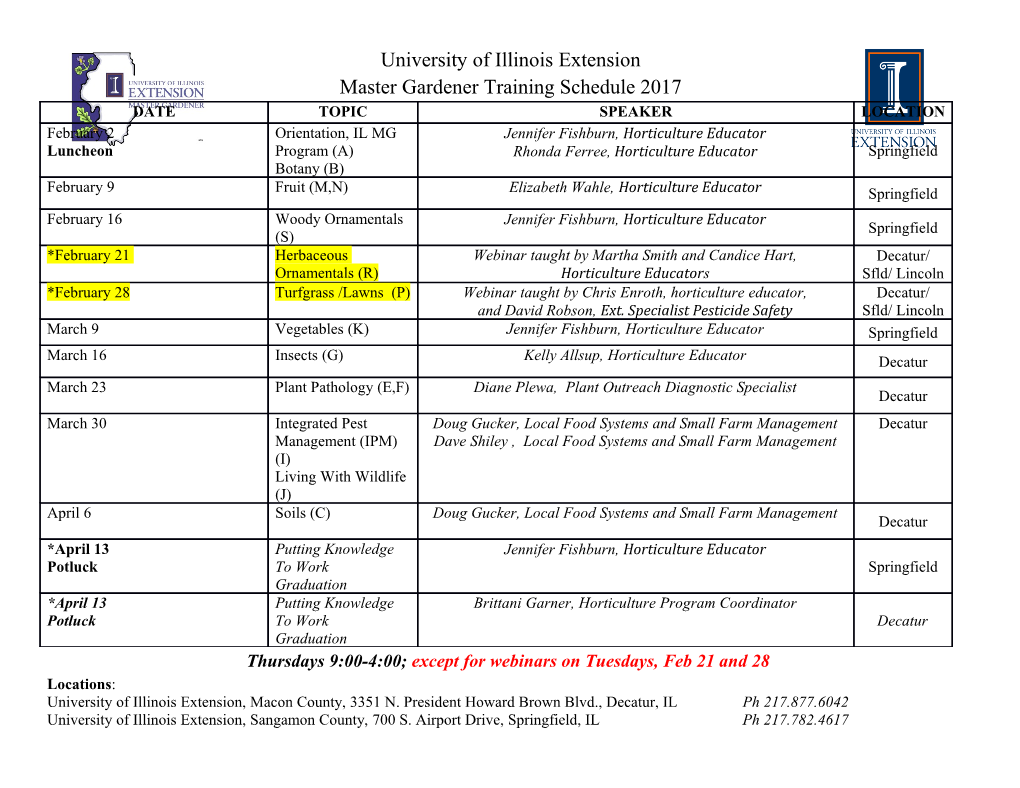
Doctoral thesis Governing Machine Learning that Matters Michael Veale 2019 A dissertation submitted in partial fulfilment of the requirements for the degree of Doctor of Philosophy in Science, Technology, Engineering and Public Policy Department of Science, Technology, Engineering and Public Policy (STEaPP) University College London 84,570 words 2019 Declaration of Authorship I, Michael Veale, confirm that the work presented in this thesis is my own. Wherein- formation has been derived from other sources, I confirm that this has been indicated in the thesis. Signed: Declaration of Integrated Publications This section acknowledges the integration of work of the Author into the different sec- tions of this thesis. All work integrated into the thesis was undertaken during the period in which the Author was registered with the University as working towards the doctorate. The work is acknowledged here as, since publication, other researchers have responded to and engaged with these works in this fast-moving field, and this thesis represents both a statement of the original arguments and findings in those works, as well as a partial response to the research field as it stands at the time of sub- mission. Chapter 1, Hello, World!, includes some content from the following articles: 1. Vasilios Mavroudis and Michael Veale, ‘Eavesdropping Whilst You’re Shopping: Balancing Personalisation and Privacy in Connected Retail Spaces’ in Proceedings of the 2018 PETRAS/IoTUK/IET Living in the IoT Conference (IET 2018) DOI: 10/gffng2; 2. Lilian Edwards and Michael Veale, ‘Slave to the Algorithm? Why a ‘Right to an Explanation’ is Probably Not The Remedy You Are Looking For’ (2017) 16 Duke L. & Tech. Rev. 18 DOI: 10/gdxthj; 3. Reuben Binns, Max Van Kleek, Michael Veale, Ulrik Lyngs, Jun Zhao and Nigel Shadbolt, ‘‘It’s Reducing a Human Being to a Percentage’; Perceptions of Justice in Algorithmic Decisions’ in Proceedings of the SIGCHI Conference on Human Factors in Computing Systems (CHI’18) (ACM 2018) DOI: 10/cvcp Chapter 2, The Law of Machine Learning?, draws upon and extends the following articles: 1. Michael Veale, Reuben Binns and Lilian Edwards, ‘Algorithms That Remember: Model Inversion Attacks and Data Protection Law’ (2018) 376 Phil. Trans. R. Soc. A 20180083 DOI: 10/gfc63m; 1 2. Michael Veale, Reuben Binns and Jef Ausloos, ‘When data protection by design and data subject rights clash’ (2018) 8(2) International Data Privacy Law 105 DOI: 10/gdxthh; 3. Michael Veale and Lilian Edwards, ‘Clarity, Surprises, and Further Questions in the Article 29 Working Party Draft Guidance on Automated Decision-Making and Profiling’ (2017) 34(2) Comput. Law & Secur. Rev. 398 DOI: 10/gdhrtm; 4. Lilian Edwards and Michael Veale, ‘Slave to the Algorithm? Why a ‘Right to an Explanation’ is Probably Not The Remedy You Are Looking For’ (2017) 16 Duke L. & Tech. Rev. 18 DOI: 10/gdxthj; 5. Lilian Edwards and Michael Veale, ‘Enslaving the Algorithm: From a “Right to an Explanation” to a “Right to Better Decisions”?’ (2018) 16(3) IEEE Security & Privacy 46 DOI: 10/gdz29v. Chapter 3, Data Protection’s Lines, Blurred by Machine Learning, draws upon and extends the following articles: 1. Michael Veale, Reuben Binns and Jef Ausloos, ‘When data protection by design and data subject rights clash’ (2018) 8(2) International Data Privacy Law 105 DOI: 10/gdxthh 2. Michael Veale, Reuben Binns and Lilian Edwards, ‘Algorithms That Remember: Model Inversion Attacks and Data Protection Law’ (2018) 376 Phil. Trans. R. Soc. A 20180083 DOI: 10/gfc63m 3. Michael Veale and Lilian Edwards, ‘Better seen but not (over)heard? Automatic lipreading systems and privacy in public spaces’ [2018] Presented at PLSC EU 2018 Chapter 4, Coping with Value(s) in Public Sector Machine Learning , draws upon and extends the following articles: 1. Michael Veale, ‘Logics and Practices of Transparency and Opacity in Real-World Applications of Public Sector Machine Learning’ in Presented at the 4th Workshop on Fairness, Accountability and Transparency in Machine Learning (FAT/ML 2017), Hal- ifax, Nova Scotia, Canada, 2017 (2017) hhttps://arxiv.org/abs/1706.09249i 2. Michael Veale, Max Van Kleek and Reuben Binns, ‘Fairness and Accountability Design Needs for Algorithmic Support in High-Stakes Public Sector Decision- Making’ in Proceedings of the SIGCHI Conference on Human Factors in Computing Sys- tems (CHI’18) (ACM 2018) DOI: 10/ct4s 2 3. Michael Veale and Irina Brass, ‘Administration by Algorithm? Public Manage- ment meets Public Sector Machine Learning’ in Karen Yeung and Martin Lodge (eds), Algorithmic Regulation (Oxford University Press 2019) Chapter 5, Unpacking a tension: ‘Debiasing’, privately , draws upon and extends the following articles: 1. Michael Veale and Reuben Binns, ‘Fairer machine learning in the real world: Mit- igating discrimination without collecting sensitive data’ (2017) 4(2) Big Data & Society DOI: 10/gdcfnz 2. Michael Veale and Irina Brass, ‘Administration by Algorithm? Public Manage- ment meets Public Sector Machine Learning’ in Karen Yeung and Martin Lodge (eds), Algorithmic Regulation (Oxford University Press 2019) Other publications produced during the course of this thesis and related to its sub- ject matter but not integrated into the document include: 1. Max Van Kleek, William Seymour, Michael Veale, Reuben Binns and Nigel Shad- bolt, ‘The Need for Sensemaking in Networked Privacy and Algorithmic Respons- ibility’ in Sensemaking in a Senseless World: Workshop at ACM CHI’18, 22 April 2018, Montréal, Canada (2018) hhttp://discovery.ucl.ac.uk/id/eprint/10046886i 2. Michael Veale, Reuben Binns and Max Van Kleek, ‘Some HCI Priorities for GDPR- Compliant Machine Learning’ in The General Data Protection Regulation: An Oppor- tunity for the CHI Community? (CHI-GDPR 2018). Workshop at ACM CHI’18, 22 April 2018, Montréal, Canada (2018) hhttps://arxiv.org/abs/1803.06174i 3. Michael Veale, Data management and use: case studies of technologies and governance (The Royal Society and the British Academy 2017) 4. Niki Kilbertus, Adria Gascon, Matt Kusner, Michael Veale, Krishna P Gummadi and Adrian Weller, ‘Blind Justice: Fairness with Encrypted Sensitive Attributes’ in Proceedings of the 35th International Conference on Machine Learning (ICML 2018) (2018) hhttp://proceedings.mlr.press/v80/kilbertus18a.htmli 5. Reuben Binns, Michael Veale, Max Van Kleek and Nigel Shadbolt, ‘Like trainer, like bot? Inheritance of bias in algorithmic content moderation’ in Giovanni Luca Ciampaglia, Afra Mashhadi and Taha Yasseri (eds), Social Informatics: 9th Interna- tional Conference, SocInfo 2017, Proceedings, Part II (Springer 2017) DOI: 10/cvc2 3 6. Michael Veale, Lilian Edwards, David Eyers, Tristan Henderson, Christopher Mil- lard and Barbara Staudt Lerner, ‘Automating Data Rights’ in David Eyers, Chris- topher Millard, Margo Seltzer and Jatinder Singh (eds), Towards Accountable Sys- tems (Dagstuhl Seminar 18181) (Dagstuhl Reports 8(4), Schloss Dagstuhl–Leibniz- Zentrum fuer Informatik 2018) DOI: 10/gffngz Abstract Personal data is increasingly used to augment decision-making and to build digital services, often through machine learning technologies, model-building tools which recognise and operationalise patterns in datasets. Researchers, regulators and civil society have expressed concern around how machine learning might create or re- inforce social challenges, such as discrimination, or create new opacities difficult to scrutinise or challenge. This thesis examines how of machine learning systems that matter–those involved in high-stakes decision-making–are and should be governed, in their technical, legal and social contexts. First, it unpacks the provisions and framework of European data protection law in relation to these social concerns and machine learning’s technical characteristics. In chapter 2, how data protection and machine learning relate is presented and ex- amined, revealing practical weaknesses and inconsistencies. In chapter 3, charac- teristics of machine learning that might further stress data protection law are high- lighted. The framework’s implicit assumptions and resultant tensions are examined through three lenses. These stresses bring policy opportunities amidst challenges, such as the chance to make clearer trade-offs and expand the collective dimension of data protection rights. The thesis then pivots to the social dimensions of machine learning on-the-ground. Chapter 4 reports upon interviews with 27 machine learning practitioners in the pub- lic sector about how they cope with value-laden choices today, unearthing a range of tensions between practical challenges and those imagined by the ‘fairness, accountab- ility and transparency’ literature in computer science. One tension between fairness and privacy is unpacked and examined in further detail in chapter 5 to demonstrate the kind of change in method and approach that might be needed to grapple with the findings of the thesis. The thesis concludes by synthesising the findings of the previous chapters, and outlines policy recommendations going forward of relevance to a range of interested parties. 4 Impact Statement Research in this thesis has been strongly motivated by a desire to create actionable knowledge. The research questions and approaches used attempt to be responsive to real-world challenges, and the research presented is directly usable by a wide range of actors. Legislators, regulators and activists can draw upon chapters 2 and 3 when look- ing to enforce, amend or reform data law to cope with emerging
Details
-
File Typepdf
-
Upload Time-
-
Content LanguagesEnglish
-
Upload UserAnonymous/Not logged-in
-
File Pages354 Page
-
File Size-