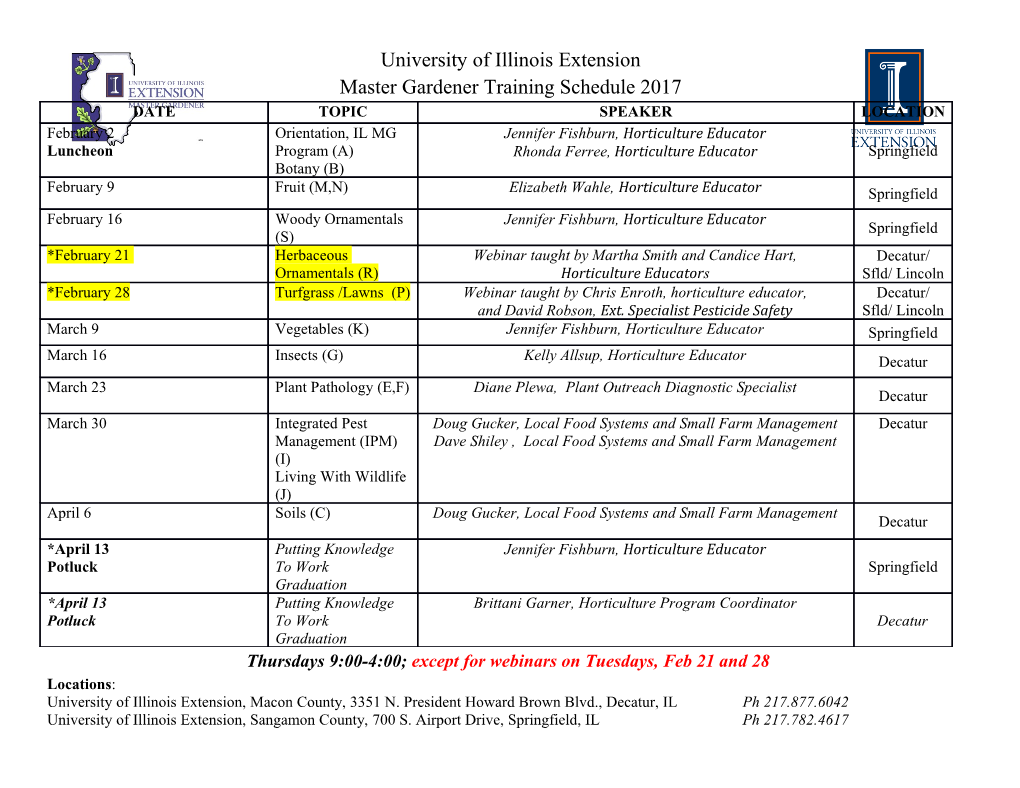
Sparse Estimation and Dictionary Learning (for Biostatistics?) Julien Mairal Biostatistics Seminar, UC Berkeley Julien Mairal Sparse Estimation and Dictionary Learning Methods 1/69 What this talk is about? Why sparsity, what for and how? Feature learning / clustering / sparse PCA; Machine learning: selecting relevant features; Signal and image processing: restoration, reconstruction; Biostatistics: you tell me. Julien Mairal Sparse Estimation and Dictionary Learning Methods 2/69 Part I: Sparse Estimation Julien Mairal Sparse Estimation and Dictionary Learning Methods 3/69 Sparse Linear Model: Machine Learning Point of View i i n i Rp Let (y , x )i=1 be a training set, where the vectors x are in and are called features. The scalars y i are in 1, +1 for binary classification problems. {− } R for regression problems. We assume there is a relation y w⊤x, and solve ≈ n 1 min ℓ(y i , w⊤xi ) + λψ(w) . w∈Rp n Xi=1 regularization empirical risk | {z } | {z } Julien Mairal Sparse Estimation and Dictionary Learning Methods 4/69 Sparse Linear Models: Machine Learning Point of View A few examples: n 1 1 i ⊤ i 2 2 Ridge regression: min (y w x ) + λ w 2. w∈Rp n 2 − k k Xi=1 n 1 i ⊤ i 2 Linear SVM: min max(0, 1 y w x ) + λ w 2. w∈Rp n − k k Xi=1 n 1 i i −y w⊤x 2 Logistic regression: min log 1+ e + λ w 2. w∈Rp n k k Xi=1 Julien Mairal Sparse Estimation and Dictionary Learning Methods 5/69 Sparse Linear Models: Machine Learning Point of View A few examples: n 1 1 i ⊤ i 2 2 Ridge regression: min (y w x ) + λ w 2. w∈Rp n 2 − k k Xi=1 n 1 i ⊤ i 2 Linear SVM: min max(0, 1 y w x )+ λ w 2. w∈Rp n − k k Xi=1 n 1 i i −y w⊤x 2 Logistic regression: min log 1+ e + λ w 2. w∈Rp n k k Xi=1 The squared ℓ2-norm induces “smoothness” in w. When one knows in advance that w should be sparse, one should use a sparsity-inducing regularization such as the ℓ1-norm. [Chen et al., 1999, Tibshirani, 1996] Julien Mairal Sparse Estimation and Dictionary Learning Methods 6/69 Sparse Linear Models: Signal Processing Point of View Let y in Rn be a signal. Let X = [x1,..., xp] Rn×p be a set of ∈ normalized “basis vectors”. We call it dictionary. X is “adapted” to y if it can represent it with a few basis vectors—that is, there exists a sparse vector w in Rp such that y Xw. We call w ≈ the sparse code. w1 w2 y x1 x2 xp . ≈ ··· . wp y∈Rn X∈Rn×p | {z } | {z } w∈Rp ,sparse | {z } Julien Mairal Sparse Estimation and Dictionary Learning Methods 7/69 The Sparse Decomposition Problem 1 2 min y Xw 2 + λψ(w) w∈Rp 2k − k sparsity-inducing data fitting term regularization | {z } | {z } ψ induces sparsity in w. It can be △ the ℓ “pseudo-norm”. w =# i s.t. wi = 0 (NP-hard) 0 k k0 { 6 } △ p the ℓ norm. w = wi (convex), 1 k k1 i=1 | | ... P This is a selection problem. When ψ is the ℓ1-norm, the problem is called Lasso [Tibshirani, 1996] or basis pursuit [Chen et al., 1999] Julien Mairal Sparse Estimation and Dictionary Learning Methods 8/69 Why does the ℓ1-norm induce sparsity? Exemple: quadratic problem in 1D 1 min (u w)2 + λ w w∈R 2 − | | Piecewise quadratic function with a kink at zero. Derivative at 0 : g = u + λ and 0 : g = u λ. + + − − − − − Optimality conditions. w is optimal iff: w > 0 and (u w)+ λ sign(w) = 0 | | − w = 0 and g 0 and g 0 + ≥ − ≤ The solution is the soft-thresholding operator: w ⋆ = sign(u)( u λ)+. | |− Julien Mairal Sparse Estimation and Dictionary Learning Methods 9/69 Why does the ℓ1-norm induce sparsity? w ⋆ w ⋆ λ √2λ − − λ u √2λ u (a) soft-thresholding operator, (b) hard-thresholding operator w ⋆ u u λ + w ⋆ u = sign( )(| | − ) , = 1 u √2λ 1 u w 2 λ w 1 | |≥ 2 minw 2 ( − ) + | | minw (u − w) + λ1 w >0 2 | | Julien Mairal Sparse Estimation and Dictionary Learning Methods 10/69 Why does the ℓ1-norm induce sparsity? Comparison with ℓ2-regularization in 1D ψ(w)= w ψ(w)= w 2 | | ψ′(w) = 2w ψ′ (w)= 1, ψ′ (w) = 1 − − + The gradient of the ℓ2-penalty vanishes when w get close to 0. On its differentiable part, the norm of the gradient of the ℓ1-norm is constant. Julien Mairal Sparse Estimation and Dictionary Learning Methods 11/69 Why does the ℓ1-norm induce sparsity? Physical illustration E1 = 0 E1 = 0 y0 Julien Mairal Sparse Estimation and Dictionary Learning Methods 12/69 Why does the ℓ1-norm induce sparsity? Physical illustration E = k1 (y y)2 1 2 0 − E = k1 (y y)2 1 2 0 − E2 = mgy k2 2 y E2 = 2 y y Julien Mairal Sparse Estimation and Dictionary Learning Methods 13/69 Why does the ℓ1-norm induce sparsity? Physical illustration E = k1 (y y)2 1 2 0 − E = k1 (y y)2 1 2 0 − k2 2 y E2 = 2 y y = 0 !! E2 = mgy Julien Mairal Sparse Estimation and Dictionary Learning Methods 14/69 Regularizing with the ℓ1-norm w1 w2 ℓ1-ball w T k k1 ≤ The projection onto a convex set is “biased” towards singularities. Julien Mairal Sparse Estimation and Dictionary Learning Methods 15/69 Regularizing with the ℓ2-norm w1 w2 ℓ2-ball w T k k2 ≤ The ℓ2-norm is isotropic. Julien Mairal Sparse Estimation and Dictionary Learning Methods 16/69 Regularizing with the ℓ -norm ∞ w1 w2 ℓ∞-ball w T k k∞ ≤ The ℓ -norm encourages w = w . ∞ | 1| | 2| Julien Mairal Sparse Estimation and Dictionary Learning Methods 17/69 In 3D. Copyright G. Obozinski Julien Mairal Sparse Estimation and Dictionary Learning Methods 18/69 What about more complicated norms? Copyright G. Obozinski Julien Mairal Sparse Estimation and Dictionary Learning Methods 19/69 What about more complicated norms? Copyright G. Obozinski Julien Mairal Sparse Estimation and Dictionary Learning Methods 20/69 Examples of sparsity-inducing penalties Exploiting concave functions with a kink at zero p ψ(w)= φ( wi ). i=1 | | P △ p q ℓq-“pseudo-norm”, with 0 < q < 1: ψ(w) = wi , i=1 | | △ p P log penalty, ψ(w) = log( wi + ε), i=1 | | P φ is any function that looks like this: φ( w ) | | w Julien Mairal Sparse Estimation and Dictionary Learning Methods 21/69 Examples of sparsity-inducing penalties (c) ℓ0.5-ball, 2-D (d) ℓ1-ball, 2-D (e) ℓ2-ball, 2-D Figure: Open balls in 2-D corresponding to several ℓq-norms and pseudo-norms. Julien Mairal Sparse Estimation and Dictionary Learning Methods 22/69 Examples of sparsity-inducing penalties The ℓ1-ℓ2 norm (group Lasso), 2 1/2 wg = w , with a partition of 1,..., p . k k2 j G { } gX∈G gX∈G Xj∈g selects groups of variables [Yuan and Lin, 2006]. the fused Lasso [Tibshirani et al., 2005] or total variation [Rudin et al., 1992]: p−1 ψ(w)= wj wj . | +1 − | Xj=1 Extensions (out of the scope of this talk): hierarchical norms [Zhao et al., 2009]. structured sparsity [Jenatton et al., 2009, Jacob et al., 2009, Huang et al., 2009, Baraniuk et al., 2010] Julien Mairal Sparse Estimation and Dictionary Learning Methods 23/69 Part II: Dictionary Learning and Matrix Factorization Julien Mairal Sparse Estimation and Dictionary Learning Methods 24/69 Matrix Factorization and Clustering Let us cluster some training vectors y1,..., ym into p clusters using K-means: m i li 2 min y x 2. (xj )p ,(l )m k − k j=1 i i=1 Xi=1 It can be equivalently formulated as m p i i 2 i i min y Xw F s.t. w 0 and wj = 1, X∈Rn p,W∈{0,1}p m k − k ≥ × × Xi=1 Xj=1 which is a matrix factorization problem: p 2 i min Y XW F s.t. W 0 and wj = 1, X∈Rn p,W∈{0,1}p m k − k ≥ × × Xj=1 Julien Mairal Sparse Estimation and Dictionary Learning Methods 25/69 Matrix Factorization and Clustering Hard clustering p 2 i min Y XW F s.t. W 0 and wj = 1, X∈Rn p,W∈{0,1}p m k − k ≥ × × Xj=1 X =[x1,..., xp] are the centroids of the p clusters. Soft clustering p 2 i min Y XW F s.t. W 0 and wj = 1, X∈Rn p,W∈Rp m k − k ≥ × × Xj=1 X =[x1,..., xp] are the centroids of the p clusters. Julien Mairal Sparse Estimation and Dictionary Learning Methods 26/69 Other Matrix Factorization Problems PCA 1 min Y XW 2 s.t. X⊤X = I and WW⊤ is diagonal. p n F W∈R × 2k − k m p X∈R × X =[x1,..., xp] are the principal components. Julien Mairal Sparse Estimation and Dictionary Learning Methods 27/69 Other Matrix Factorization Problems Non-negative matrix factorization [Lee and Seung, 2001] 1 min Y XW 2 s.t. W 0 and X 0. p n F W∈R × 2k − k ≥ ≥ m p X∈R × Julien Mairal Sparse Estimation and Dictionary Learning Methods 28/69 Dictionary Learning and Matrix Factorization [Olshausen and Field, 1997] n 1 i i 2 i min y Xw F + λ w 1, X∈X ,W∈Rp m 2k − k k k × Xi=1 which is again a matrix factorization problem 1 2 min Y XW + λ W 1.
Details
-
File Typepdf
-
Upload Time-
-
Content LanguagesEnglish
-
Upload UserAnonymous/Not logged-in
-
File Pages101 Page
-
File Size-