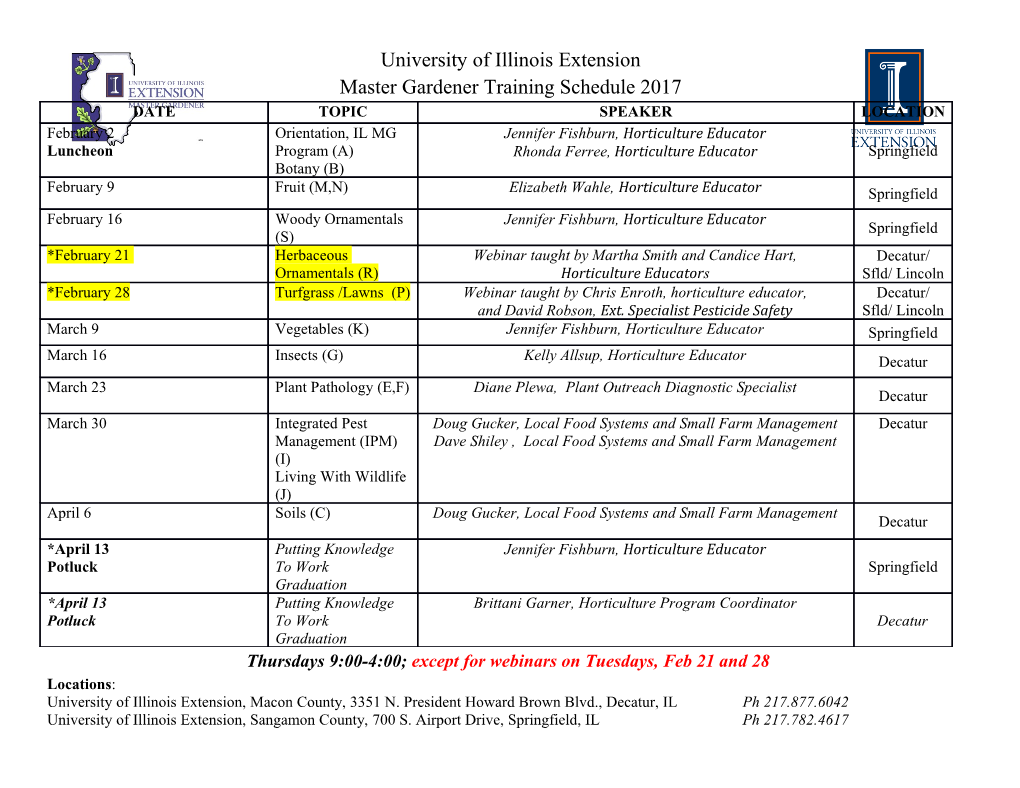
Comparison of Dimensionality Reduction Techniques on Audio Signals Tamás Pál, Dániel T. Várkonyi Eötvös Loránd University, Faculty of Informatics, Department of Data Science and Engineering, Telekom Innovation Laboratories, Budapest, Hungary {evwolcheim, varkonyid}@inf.elte.hu WWW home page: http://t-labs.elte.hu Abstract: Analysis of audio signals is widely used and this work: car horn, dog bark, engine idling, gun shot, and very effective technique in several domains like health- street music [5]. care, transportation, and agriculture. In a general process Related work is presented in Section 2, basic mathe- the output of the feature extraction method results in huge matical notation used is described in Section 3, while the number of relevant features which may be difficult to pro- different methods of the pipeline are briefly presented in cess. The number of features heavily correlates with the Section 4. Section 5 contains data about the evaluation complexity of the following machine learning method. Di- methodology, Section 6 presents the results and conclu- mensionality reduction methods have been used success- sions are formulated in Section 7. fully in recent times in machine learning to reduce com- The goal of this paper is to find a combination of feature plexity and memory usage and improve speed of following extraction and dimensionality reduction methods which ML algorithms. This paper attempts to compare the state can be most efficiently applied to audio data visualization of the art dimensionality reduction techniques as a build- in 2D and preserve inter-class relations the most. ing block of the general process and analyze the usability of these methods in visualizing large audio datasets. 2 Related Work 1 Introduction A large number of research addresses the problem of map- ping a collection of sounds into a 2-dimensional map and With recent advances in machine learning, audio, speech, examining the results both visually and analytically. and music processing has evolved greatly, with many ap- Roma et al. [2] used MFCC and autoencoders as feature plications like searching, clustering, classification, and extraction methods and compared PCA, tSNE, Isomap, tagging becoming more efficient and robust through ma- MDS, SOM and the Fruchterman-Reingold dimensional- chine learning techniques. Well performing dimension- ity reduction to map the output of the feature extraction ality reduction methods have been successfully applied process into 2D. in many areas of computer science and interdisciplinary Fedden [7] used a very similar approach, with only fields. different methods tried. As far as feature extraction is A very important phenomenon that justifies the use of concerned, MFCCs and an autoencoder-like architecture such methods is the curse of dimensionality. The ba- called NSynth were used. PCA, t-SNE, UMAP methods sic idea is that the increase of dimensionality increases were used for dimensionality reduction. the volume of space, causing the data to become sparse. Hantrakul et al. [8] implements the usual pipeline of Therefore, the amount of data required for a model to applying feature extraction, reshaping, then dimensional- achieve high accuracy and efficiency increases greatly. ity reduction into 2D. STFT, MFCC, high-level features Considering audio signals, meaningful features need to and the Wavenet encoder were used for feature extrac- be extracted before applying any dimensionality reduction tion. PCA, UMAP and t-SNE were the candidates for the method. Using methods for feature extraction like Short next step. The dataset consisted of different drum sample Time Fourier Transformation (STFT), Mel-frequency cep- sounds. The results were analyzed through external clus- stral coefficient (MFCC), or high-level descriptors like tering metrics like homogeneity, completeness score and zero-crossing-rate, representative data can be extracted V-measure, using k-means as clustering method. from the audio data. After extraction these features can Dupont et al. [11] used MFCCs combined with spec- be projected into two-dimensional space for cluster analy- tral flatness to represent the extracted information from the sis and examination of class separation. audio. PCA, Isomap and t-SNE were chosen for dimen- The dataset used is the UrbanSound 8k dataset, contain- sionality reduction. In addition, supervised dimensional- ing 8732 labeled sound excerpts (≤ 4 seconds) of urban ity reduction methods were included as well, like LDA sounds from 10 classes, from which 5 have been used in (Linear Discriminant Analysis) and HDA (Heteroscedas- tic Discriminant Analysis). Charles Lo’s work [1] is focused on dimensionality re- Copyright c 2020 for this paper by its authors. Use permitted un- der Creative Commons License Attribution 4.0 International (CC BY duction for music feature extraction. The author used fea- 4.0). ture vectors composed of: 13 MFCCs and high-level fea- tures. Locally Linear Embedding (LLE), Autoencoder, t- 1. Low: 1 Hz, High: 10000 Hz SNE and PCA were used to map into lower dimensions. The dataset contained 1000 song clips equally distributed 2. Low: 25 Hz, High: 9000 Hz from 10 musical genres. In order to test the results, Gaus- 3. Low: 50 Hz, High: 8500 Hz sian mixture models were implemented to test cluster pu- rity and supervised classification was also performed with 4. Low: 100 Hz, High: 8000 Hz kNN classifier. tSNE achieved the best classification per- 5. Low: 150 Hz, High: 7000 Hz formance. 4.2 Feature Extraction 3 Basic Notation In order to extract meaningful data, that can be interpreted Given a dataset of discrete audio signals, we de- by machine learning models, the step of feature extraction note the i-th recording as x(ii) and the dataset as is required. This step also acts as a dimensionality reduc- X = fx(1);x(2);:::;x(N)g. The corresponding ground- tion process, because it transforms a sound segment repre- truth labels are stored in Y = fy(1);y(2);:::;y(N)g. A single sented by hundreds of thousands of samples to a data struc- audio element of the dataset is comprised of a number of ture containing only a few thousand values or less. This is (ii) (i) (i) (i) a crucial step as it enables to apply dimensionality reduc- samples: x = (x1 ;x2 ;:::xM ). tion methods later that will ultimately project the sample into the lowest dimensionality of just 2 components. 4 Methods STFT The Short-time Fourier transform (STFT) cap- The building blocks of the process used in this work are tures both temporal and spectral information from a sig- presented in the following sections and are also shown on nal. As a result, the STFT transforms the signal from figure 1. As seen on the figure, a set of discrete audio sig- time-amplitude representation to time-frequency represen- nals are transformed trough consecutive methods to result tation. Formally, the STFT is calculated by applying the in a 2D map of datapoints. discrete Fourier transform with a sliding window to over- lapping segments of the signal. It can be formulated in the following way: L−1 (i) 2p STFTfx(ii)g := S(i)[k;n] = ∑ x(i)[n + m]w[m]e− jk L m m=0 (1) where w[m] is the window function, L is the window length, n denotes the frame number and k denotes the fre- quency in question. The STFT returns a complex-valued matrix, where jS[n;k]j is the magnitude of the frequency bin k at time- frame n. Mel-scaled STFT As human perception of pitch (fre- Figure 1: Steps of the process quency) is logarithmic, the Mel-scale is often applied to the frequency axis of the STFT output. Specifically, the Mel-scale is linearly spaced below 1000 Hz and turns log- 4.1 Band-pass filtering arithmic afterward. The Mel-scaled frequency value can be computed from Applying filtering of some kind before feature extraction a frequency( f ) in Hertz using the following equation: could be justifiable to remove frequency intervals that usu- ally do not have discriminative power. The simplest such f Mel( f ) = 2595 · log (1 + ) (2) solution is applying a band-pass filter. A band-pass filter 10 700 passes frequencies between a lower limit fL and a higher limit fH , while rejecting frequencies outside this interval. MFCC Mel Frequency Cepstral coefficients are widely Band-pass filters are usually implemented using Butter- used in audio and speech recognition, and were introduced worth filters, as these have maximally flat frequency re- by Paul Mermelstein in 1976 [10]. Overview of the pro- sponse in the passband. cess: The following low-cutoff and high-cutoff frequency pairs have been used as band-pass filter specifications: 1. Take the Short-time Fourier transform of the signal. 2. Apply the Mel filterbank to the power spectra of each • Spectral bandwidth: frame, summing the energies in each frequency band. s K−1 2 2 The Mel filterbank is a set of overlapping triangular ∑ (k − vSC(n)) · jS[k;n]j v (n) = k=0 (8) filters. SS K−1 2 ∑k=0 jS[k;n]j 3. Take the logarithm of the calculated energies. The spectral bandwidth is a measure of the average 4. Take the Discrete Cosine Transform (DCT) of the log spread of the spectrum in relation to its centroid. filterbank energies, resulting in a so-called cepstrum. Intuitively, the cepstrum captures information of the • Spectral flatness: rate of change in frequency bands. The DCT is re- q lated to the DFT (Discrete Fourier Transform). While K K−1 ∏k=0 jS[k;n]j DFT uses both sine and cosine functions to express a vSF (n) = (9) 1 K−1 jS[k;n]j function as a sum of these sinusoids, the DCT uses K ∑k=0 only cosine functions. Formally (using the DCT-II): The spectral flatness is the ratio of geometric mean N−1 p 1 and arithmetic mean of the magnitude spectrum.
Details
-
File Typepdf
-
Upload Time-
-
Content LanguagesEnglish
-
Upload UserAnonymous/Not logged-in
-
File Pages8 Page
-
File Size-