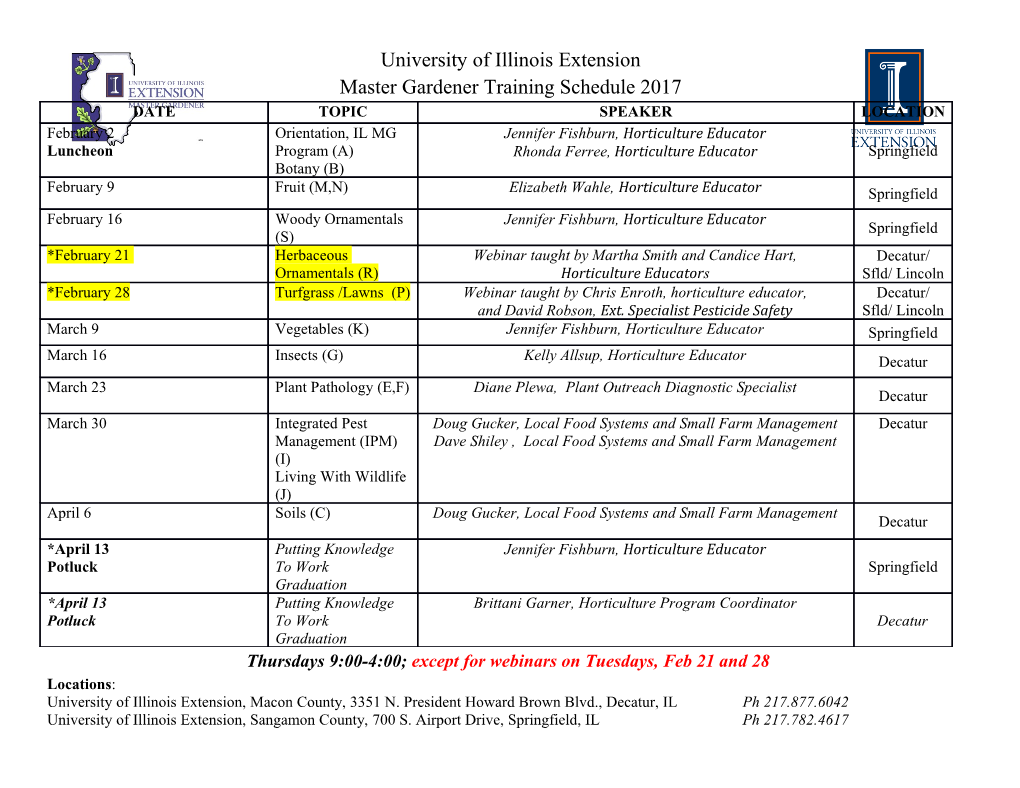
Treatment and Spillover Effects under Network Interference Michael P. Leung˚ July 3, 2016 Abstract. We study identification and estimation of treatment and spillover effects when interference is mediated by a network, focusing on the case of ran- domized assignment. We show that identification of spillover effects is possible with only a single, possibly sampled, network under common shape restrictions on treatment response. Estimation in the single-network setting is nonstan- dard due to correlation between outcomes induced by network spillovers and correlated effects. Nonetheless, under restrictions on moments of the network, nonparametric estimators for treatment and spillover effects are consistent and asymptotically normal, and we can construct consistent variance estimators. We also derive analogous results for GMM estimators, including robust stan- dard errors. JEL Codes: C13, C21 Keywords: social networks, treatment effects, spillovers, SUTVA, Stein’s method ˚USC, Department of Economics and MIT, Institute for Data, Systems, and Society. E-mail: [email protected]. 1 Michael P. Leung 1 Introduction A vast literature in econometrics and statistics studies identification and estimation of treatment effects. In this literature, the stable-unit treatment value assumption (SUTVA) is a fundamental assumption, which states that the ego’s treatment response is invariant to the treatment assignment of any alter. In other words, there is no “interference” or are no “spillovers” in treatment assignment. However, there are many important contexts in which this assumption fails to hold, and the measurement of spillover effects is often of inherent interest. This paper studies identification and estimation of treatment and spillover effects in the absence of SUTVA. In our model, treatment response may depend on the treat- ment assignment of others in a network. We focus on randomized assignment, which is relevant for a wide variety of experimental contexts, including those in development economics (Bhattacharya et al., 2013; Duflo et al., 2011; Miguel and Kremer, 2004), social economics (Kling et al., 2007; Sobel, 2006), medical science (Christakis, 2004; Kim et al., 2015; Valente et al., 2007), and the study of online social networks (Bond et al., 2012; Kramer et al., 2014).1 Unlike most papers in the literature, we assume the econometrician observes a small sample of networks, possibly only a single network. Our results also hold if the network is sampled under a common sampling scheme. Because standard inference procedures treat the network, rather than the node (individual), as the unit of observation, a common strategy is to partition the observed network into subunits, which are then assumed to be independent, often without apparent justification. Our analysis obviates the need to arbitrarily cluster in this fashion. Perhaps as a consequence, the collection of extensive data on many social networks through large-scale surveys is becoming more common (e.g. Banerjee et al., 2013; Cai et al., 2015). Our results substantially reduce the burden of data collection, since, at least under our assumptions, it is unnecessary to sample a large number of networks for the purposes of internal validity. Let the treatment response Yi of any node i be given by Yi “ rpi; D; G; "iq; where D is the vector of treatment assignments for all nodes in the network G, and "i is unobserved heterogeneity. We are interested in functionals of the response function rp¨q, for instance the average structural function (ASF) Errpi; d; g; "iqs, where d and g are fixed realizations of the treatment assignment vector and the network. Average treatment and spillover effects can then be recovered from differences Errpi; d; g; "iqs´ 1 1 Errpi; d ; g ;"iqs. The quantile structural function (QSF) and quantile treatment and spillover effects can be similarly defined. 1Other works in applied economics that estimate treatment spillovers in experimental settings include Bandiera et al.(2009), Bursztyn et al.(2014), Cai et al.(2015), Duflo and Saez(2003), Dupas(2014), and Oster and Thornton(2012). 2 Treatment and Spillover Effects If the econometrician observes a large number of independent networks, then iden- tification and estimation largely follow from the standard theory by viewing networks as “individuals” and a vector of treatment assignments as the treatment assigned to a network, assuming SUTVA now holds across networks. Instead, we consider an asymptotic sequence that sends the size of a single network, rather than the number of networks, to infinity, to approximate the finite-sample reality in which we observe a small sample of networks. Note that multiple networks is a special case, since one can define the union of all observed networks as a single network. It is evident that without further restrictions on rp¨q, identification of treat- ment/spillover effects is impossible in a single-network context, since the dimensions of D and G grow with the number of nodes. We therefore consider two natural shape restrictions on rp¨q that are often used in practice, namely local spillovers (individ- ual responses may depend only on own treatment and the treatment assignments of network neighbors) and anonymity (individual identities do not enter the response function). We find that rp¨q satisfies local spillovers and anonymity if and only if pi; D; Gq enter rp¨q only through the following vector of sufficient statistics: own treatment assignment, number of treated neighbors, and number of untreated neigh- bors. This is useful because the dimension of this vector is fixed with respect to the network size. Then under nontrivial treatment assignment and support conditions on the degree distribution,2 the average and quantile structural functions are identified, provided consistent estimators exist. Estimation and inference requires a central limit theorem, but the dependence structure of the set of outcomes is nonstandard and network-dependent. In particular, conditional on G, the responses of two nodes are dependent if the nodes are connected or they share a common network neighbor, due to treatment spillovers. There may also be dependence due to correlated effects. Notice that unlike time series or spatial settings, there is no underlying metric space with which to define α-mixing or other more standard forms of dependence. Fortunately, a central limit theorem may still be proved using Stein’s method (Penrose, 2003; Ross, 2011). This implies a weak law of large numbers, and therefore the ASF and QSF can be nonparametrically estimated using sample conditional means. These convergence results hold conditional on G, provided certain restrictions on “moments” of G are satisfied. We provide primitive conditions on a large class of network formation models that guarantee these restrictions hold. An additional complication is that, conditional on the network, responses Yi are non-identically distributed, since they depend on the network through spillovers. It is well-known that even in the case of independent and non-identically distributed random variables, it is impossible in general to consistently estimate the asymptotic variance of means, and only conservative upper bounds are estimable. Fortunately, we find that local spillovers and anonymity generate sufficient homogeneity in our 2The degree of a node is its number of connections. A network G’s degree distribution is the distribution of node degrees. 3 Michael P. Leung setting that a consistent estimator for the asymptotic variance exists for nonpara- metric estimators of treatment and spillover effects. Therefore, asymptotically exact inference is possible. Lastly, we derive analogous results for linear regression, including robust standard errors that account for dependence due to spillovers. We also discuss generalizations to method of moments. While we focus on network-driven sources of dependence, our approach accommodates more general forms of heterogeneous cross-sectional depen- dence including spatial correlation and clustering with overlapping groups. A growing literature in statistics studies estimation of treatment effects when SUTVA is violated (e.g. Basse and Airoldi, 2015; Hudgens and Halloran, 2012; Toulis and Kao, 2013). The majority of papers implicitly assume that the sample contains a large number of independent networks or groups, which, as previously noted, simply corresponds to assuming SUTVA across groups. While we study structural quantities such as the ASF, this literature focuses on estimands 1 n 1 1 yipdq ´ 1 yipdq ; (1) n »|Di| |Di| 1 fi i“1 dPDi dPD ¸ ¸ ¸i – fl 1 where Di; Di are sets of treatment assignment vectors D, and yip¨q is the treatment 3 1 response function. For instance, Toulis and Kao define “k-level effects” by setting Di to be a singleton set containing the zero vector (no treated nodes) and Di to be the set of treatment assignments in which exactly k neighbors of i are treated. While such estimands appear to be natural analogs of the average treatment effect (ATE) under SUTVA, there are many possible choices of Di, and it is unclear why averaging responses over treatment assignments within such classes delivers policy- relevant estimands without further restrictions on the response function. Aronow and Samii(2015) impose homogeneity in the response function by assuming that 1 yipdq “ yipd q for all d P Di, but this still leaves open the question of the proper choice of Di. In this respect, one of our contributions is to show that natural and widely used shape restrictions on the response function exist that justify simple choices of Di. In particular, we can let Di “ Dipd; t; uq, the set of pD; Gq under which i is assigned to treatment d, i has t treated network neighbors, and i has u untreated network neighbors. Furthermore, for such choices of Di, the probability limit of each term in the difference (1) has a structural interpretation as an ASF, and the QSF may be similarly defined. Aronow and Samii(2015) and van der Laan(2014) are some of the few papers that discuss inference with a single large network. There are several differences between the work of Aronow and Samii and ours.
Details
-
File Typepdf
-
Upload Time-
-
Content LanguagesEnglish
-
Upload UserAnonymous/Not logged-in
-
File Pages38 Page
-
File Size-