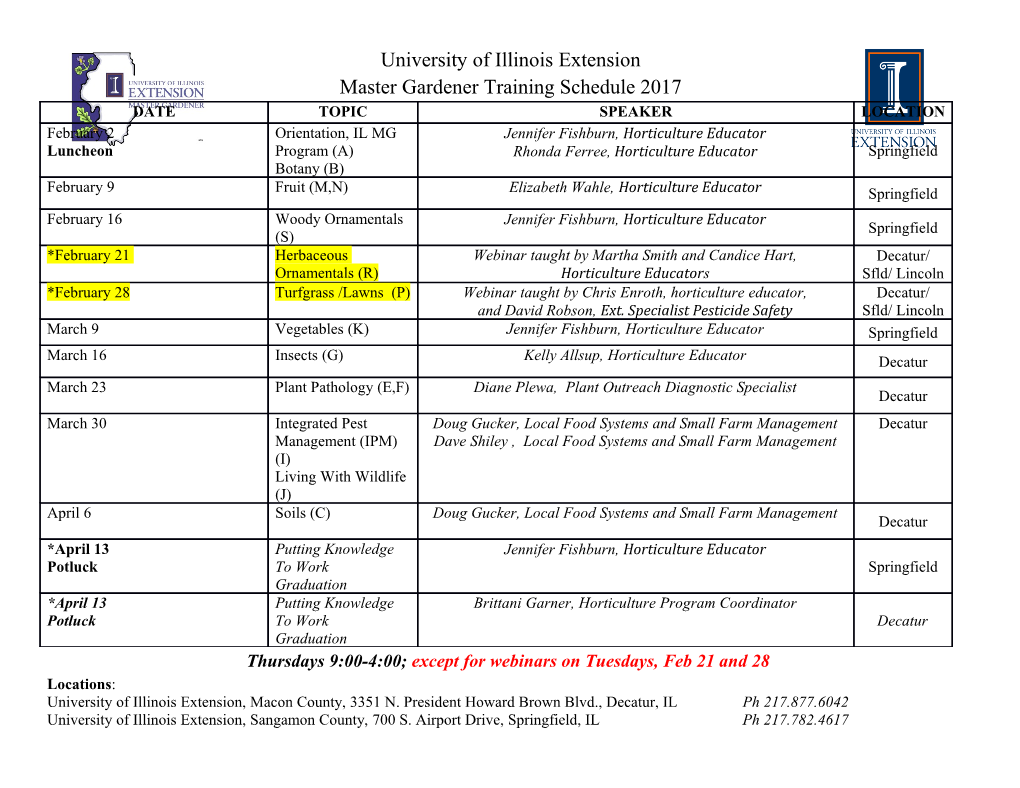
Leveraging Frame Semantics and Distributional Semantics for Unsupervised Semantic Slot Induction in Spoken Dialogue Systems (Extended Abstract) Yun-Nung Chen, William Yang Wang, and Alexander I. Rudnicky School of Computer Science, Carnegie Mellon University 5000 Forbes Ave., Pittsburgh, PA 15213-3891, USA fyvchen, yww, [email protected] Abstract for designing spoken dialogue systems (SDS) in an unsupervised fashion. Comparing to the tradi- Although the spoken dialogue system tional approach where domain experts and devel- community in speech and the semantic opers manually define the semantic ontology for parsing community in natural language SDS, the unsupervised approach has the advan- processing share many similar tasks and tages to reduce the costs and avoid human induced approaches, they have progressed inde- bias. pendently over years with few interac- On the other hand, the distributional view of se- tions. This paper connects two worlds to mantics hypothesizes that words occurring in the automatically induce the semantic slots for same contexts may have similar meanings (Har- spoken dialogue systems using frame and ris, 1954). With the recent advance of deep learn- distributional semantic theories. Given ing techniques, the continuous representation of a collection of unlabeled audio, we ex- word embeddings has further boosted the state- ploit continuous-valued word embeddings of-the-art results in many applications, such as to augment a probabilistic frame-semantic frame identification (Hermann et al., 2014), sen- parser that identifies key semantic slots timent analysis (Socher et al., 2013), language in an unsupervised fashion. Our exper- modeling (Mikolov, 2012), and sentence comple- iments on a real-world spoken dialogue tion (Mikolov et al., 2013a). dataset show that distributional word rep- In this paper, given a collection of unlabeled resentation significantly improves adapta- raw audio files, we investigate an unsupervised ap- tion from FrameNet-style parses of rec- proach for semantic slot induction. To do this, we ognized utterances to the target semantic use a state-of-the-art probabilistic frame-semantic space, that comparing to a state-of-the-art parsing approach (Das et al., 2010; Das et al., baseline, a 12% relative mean average pre- 2014), and perform an adaptation process, map- cision improvement is achieved, and that ping the generic FrameNet (Baker et al., 1998) the proposed technology can be used to re- style semantic parses to the target semantic space duce the costs for designing task-oriented that is suitable for the domain-specific conversa- spoken dialogue systems. tion settings. We utilize continuous word em- beddings trained on very large external corpora 1 Introduction (e.g. Google News and Freebase) for the adap- Frame semantics is a linguistic theory that de- tation process. To evaluate the performance of our fines meaning as a coherent structure of re- approach, we compare the automatically induced lated concepts (Fillmore, 1982). Although there semantic slots with the reference slots created by has been some successful applications in natural domain experts. Empirical experiments show that language processing (Hedegaard and Simonsen, the slot creation results generated by our approach 2011; Coyne et al., 2011; Hasan and Ng, 2013), align well with those of domain experts. this linguistically principled theory has not been 2 The Proposed Approach explored in the speech community until recently: Chen et al. (2013b) showed that it is possible to We build our approach on top of the recent suc- use probabilistic frame-semantic parsing to auto- cess of an unsupervised frame-semantic parsing matically induce and adapt the semantic ontology approach. Chen et al. (2013b) formulated the se- can i have a cheap restaurant 2.2 Continuous Space Word Representations To better adapt the FrameNet-style parses to the Frame: expensiveness target task-oriented SDS domain, we make use of FT LU: cheap continuous word vectors derived from a recurrent Frame: capability Frame: locale by use neural network architecture (Mikolov et al., 2010). FT LU: can FE Filler: i FT/FE LU: restaurant The recurrent neural network language models use Figure 1: An example of probabilistic frame- the context history to include long-distance in- semantic parsing on ASR output. FT: frame target. formation. Interestingly, the vector-space word FE: frame element. LU: lexical unit. representations learned from the language mod- els were shown to capture syntactic and semantic regularities (Mikolov et al., 2013c; Mikolov et al., mantic mapping and adaptation problem as a rank- 2013b). The word relationships are characterized ing problem, and proposed the use of unsupervised by vector offsets, where in the embedded space, clustering methods to differentiate the generic se- all pairs of words sharing a particular relation are mantic concepts from target semantic space for related by the same constant offset. Considering task-oriented dialogue systems. However, their that this distributional semantic theory may bene- clustering approach only performs on the small fit our SLU task, we leverage word representations in-domain training data, which may not be robust trained from large external data to differentiate se- enough. Therefore, this paper proposes a radical mantic concepts. extension of the previous approach: we aim at im- proving the semantic adaptation process by lever- 2.3 Slot Ranking Model aging distributed word representations. Our model ranks the slot candidates by integrat- ing two scores (Chen et al., 2013b): (1) the rela- 2.1 Probabilistic Semantic Parsing tive frequency of each candidate slot in the corpus, FrameNet is a linguistically-principled semantic since slots with higher frequency may be more resource (Baker et al., 1998), developed based on important. (2) the coherence of slot-fillers cor- the frame semantics theory (Fillmore, 1976). In responding to the slot. Assuming that domain- our approach, we parse all ASR-decoded utter- specific concepts focus on fewer topics and are ances in our corpus using SEMAFOR, a state-of- similar to each other, the coherence of the corre- the-art semantic parser for frame-semantic pars- sponding values can help measure the prominence ing (Das et al., 2010; Das et al., 2014), and ex- of the slots. tract all frames from semantic parsing results as w(s ) = (1 − α) · log f(s ) + α · log h(s ); (1) slot candidates, where the LUs that correspond to i i i the frames are extracted for slot-filling. For ex- where w(si) is the ranking weight for the slot can- ample, Figure 1, shows an example of SEMAFOR didate si, f(si) is the frequency of si from seman- parsing of an ASR-decoded text output. tic parsing, h(si) is the coherence measure of si, Since SEMAFOR was trained on FrameNet and α is the weighting parameter within the inter- annotation, which has a more generic frame- val [0; 1]. semantic context, not all the frames from the pars- For each slot si, we have the set of correspond- ing results can be used as the actual slots in the ing slot-fillers, V (si), constructed from the utter- domain-specific dialogue systems. For instance, ance including the slot si in the parsing results. in Figure 1, we see that the “expensiveness” The coherence measure h(si) is computed as av- and “locale by use” frames are essentially the erage pair-wise similarity of slot-fillers to evaluate key slots for the purpose of understanding in the if slot si corresponds to centralized or scattered restaurant query domain, whereas the “capability” topics. frame does not convey particular valuable infor- P mation for SLU. In order to fix this issue, we com- Sim(xa; xb) h(s ) = xa;xb2V (si);xa6=xb ; pute the prominence of these slot candidates, use i 2 (2) jV (si)j a slot ranking model to rerank the most frequent slots, and then generate a list of induced slots for where V (si) is the set of slot-fillers correspond- use in domain-specific dialogue systems. ing slot si, jV (si)j is the size of the set, and Sim(xa; xb) is the similarity between the pair of can be computed as the cosine similarity between fillers xa and xb. The slot si with higher h(si) rxa and rxb , called NeiSim(xa; xb). usually focuses on fewer topics, which is more The idea of using NeiSim(xa; xb) is very sim- specific and more likely for slots occurring in dia- ilar as using RepSim(xa; xb), where we assume logue systems. that words with similar concepts should have sim- We involve distributional semantics of slot- ilar representations and share similar neighbors. fillers xa and xb for deriving Sim(xa; xb). Hence, NeiSim(xa; xb) is larger when xa and xb Here, we propose two similarity measures: have more overlapped neighbors in continuous the representation-derived similarity and the space. neighbor-derived similarity as Sim(xa; xb) in (2). 3 Experiments 2.3.1 Representation-Derived Similarity We examine the slot induction accuracy by com- Given that distributional semantics can be cap- paring the reranked list of frame-semantic pars- tured by continuous space word representa- ing induced slots with the reference slots created tions (Mikolov et al., 2013c), we transform each by system developers. Furthermore, using the token x into its embedding vector x by pre-trained reranked list of induced slots and their associated distributed word representations, and then the sim- slot fillers (value), we compare against the human ilarity between a pair of slot-fillers x and x a b annotation. For the slot-filling task, we evaluate can be computed as their cosine similarity, called both on ASR transcripts of the raw audio, and on RepSim(x ; x ). a b the manual transcripts. We assume that words occurring in similar domains have similar word representations thus 3.1 Experimental Setup RepSim(x ; x ) will be larger when x and x are a b a b In this experiment, we used the Cambridge Uni- semantically related.
Details
-
File Typepdf
-
Upload Time-
-
Content LanguagesEnglish
-
Upload UserAnonymous/Not logged-in
-
File Pages6 Page
-
File Size-