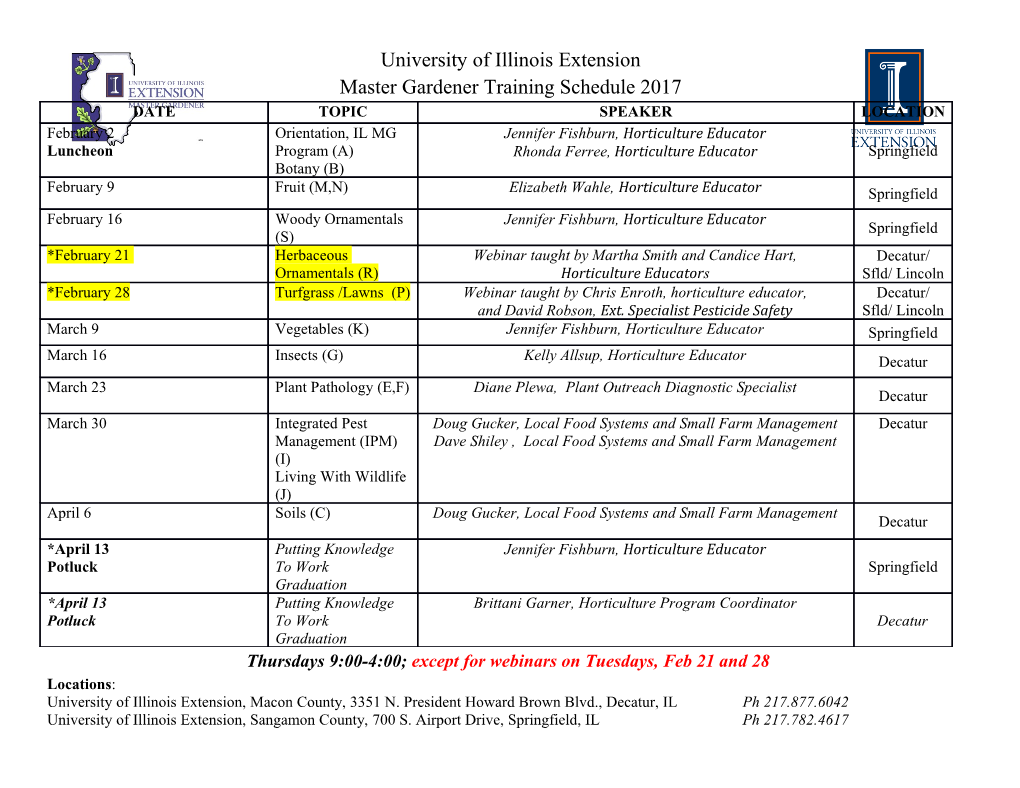
University of Massachusetts Medical School eScholarship@UMMS PEER Liberia Project UMass Medical School Collaborations in Liberia 2019-2 Introduction to Biostatistics - Lecture 3: Statistical Inference for Proportions Jonggyu Baek University of Massachusetts Medical School Let us know how access to this document benefits ou.y Follow this and additional works at: https://escholarship.umassmed.edu/liberia_peer Part of the Biostatistics Commons, Family Medicine Commons, Infectious Disease Commons, Medical Education Commons, and the Public Health Commons Repository Citation Baek J. (2019). Introduction to Biostatistics - Lecture 3: Statistical Inference for Proportions. PEER Liberia Project. https://doi.org/10.13028/9g0p-am33. Retrieved from https://escholarship.umassmed.edu/ liberia_peer/11 This material is brought to you by eScholarship@UMMS. It has been accepted for inclusion in PEER Liberia Project by an authorized administrator of eScholarship@UMMS. For more information, please contact [email protected]. Introduction to Biostatistics 2/29/2019 Jonggyu Baek, PhD Lecture 3: Statistical Inference for proportions 2 CTS605A - Lecture Notes, Jonggyu Baek, PhD Statistical Inference Two broad areas of statistical inference: • Estimation: Use sample statistics to estimate the unknown population parameter. – Point Estimate: the best single value to describe the unknown parameter. – Standard Error (SE): standard deviation of the sample statistic. Indicates how precise is the point estimate. – Confidence Interval (CI): the range with the most probable values for the unknown parameter with a (1-α)% level of confidence. • Hypothesis Testing: Test a specific statement (assumption) about the unknown parameter. 3 CTS605A - Lecture Notes, Jonggyu Baek, PhD Statistical Inference for proportions Suppose X: discrete (binary) variable with: 1, event A with probability p X=ቊ 0, otherwise with probability 1 − p We are interested in estimating the probability p of the event A in a population of size N: # of favorable outcomes = # of successes (1) P(event A) = sample space Total # of units in the population N Suppose Y = # of successes = σi=1 Xi. Then Y ~ Binomial(N, p) σN X P(event A) = i=1 i N 4 CTS605A - Lecture Notes, Jonggyu Baek, PhD Statistical Inference for proportions Suppose X: discrete (binary) variable with: 1, event A with probability p X=ቊ 0, otherwise with probability 1 − p We are interested in estimating the probability p of the event A in a population of size N: # of favorable outcomes = # of successes (1) P(event A) = sample space Total # of units in the population N Suppose Y = # of successes = σi=1 Xi. Then Y ~ Binomial(N, p) σN X P(event A) = i=1 i N 5 CTS605A - Lecture Notes, Jonggyu Baek, PhD Statistical Inference for proportions Suppose X: discrete (binary) variable with: 1, event A with probability p X=ቊ 0, otherwise with probability 1 − p We are interested in estimating the probability p of the event A in a population of size N: # of favorable outcomes = # of successes (1) P(event A) = sample space Total # of units in the population N Suppose Y = # of successes = σi=1 Xi. Then Y ~ Binomial(N, p) σN X P(event A) = i=1 i N 6 CTS605A - Lecture Notes, Jonggyu Baek, PhD Statistical Inference for proportions Suppose X: discrete (binary) variable with: 1, event A with probability p X=ቊ 0, otherwise with probability 1 − p We are interested in estimating the probability p of the event A in a population of size N: # of favorable outcomes = # of successes (1) P(event A) = sample space Total # of units in the population N Suppose Y = # of successes = σi=1 Xi. Then Y ~ Binomial(N, p) σN X P(event A) = i=1 i N 7 CTS605A - Lecture Notes, Jonggyu Baek, PhD Statistical Inference for proportions Suppose X: discrete (binary) variable with: 1, event A with probability p X=ቊ 0, otherwise with probability 1 − p We are interested in estimating the probability p of the event A in a population of size N: # of favorable outcomes = # of successes (1) P(event A) = sample space Total # of units in the population N Suppose Y = # of successes = σi=1 Xi. Then Y ~ Binomial(N, p) What is this? σN X P(event A) = i=1 i N 8 CTS605A - Lecture Notes, Jonggyu Baek, PhD Statistical Inference for proportions Suppose X: discrete (binary) variable with: 1, event A with probability p X=ቊ 0, otherwise with probability 1 − p We are interested in estimating the probability p of the event A in a population of size N: # of favorable outcomes = # of successes (1) P(event A) = sample space Total # of units in the population N Suppose Y = # of successes = σi=1 Xi. Then Y ~ Binomial(N, p) What is this? σN X P(event A) = i=1 i = p • A proportion N • A population mean Hence, all the statistical inference procedures we learned about the means also apply for proportions. 9 CTS605A - Lecture Notes, Jonggyu Baek, PhD Statistical Inference for proportions • Case 1: single population (one-sample) • Case 2: two-independent populations (two-samples) • Case 3: two-dependent populations (paired or matched samples) 10 CTS605A - Lecture Notes, Jonggyu Baek, PhD One Sample Case 1: single population (one-sample) Suppose X=‘stroke’ from a population with mean μ=p and standard deviation σ= 퐩(ퟏ − 퐩). Estimation • Point Estimates: – of p: xത = pො – of σ: s = pො(1 − pො) pෝ(1−pෝ) – precision of xത : standard error (s.e.) of pො→ n • (1-α)% CI: pෝ(1−pෝ) pෝ(1−pෝ) [ pො- Z ( ) , pො+ Z ( )] 1-α/2 n 1-α/2 n 11 CTS605A - Lecture Notes, Jonggyu Baek, PhD One Sample Case 1: single population (one-sample) Suppose X=‘stroke’ from a population with mean μ=p and standard deviation σ= 퐩(ퟏ − 퐩). • Point Estimates: • If the binary variable is code as [“1”=yes, “0”=no] we can also calculate the mean: 12 One Sample Case 1: single population (one-sample) Suppose X=‘stroke’ from a population with mean μ=p and standard deviation σ= 퐩(ퟏ − 퐩). • Point Estimates: • If the binary variable is code as [“1”=yes, “0”=no] we can also calculate the mean: 퐩ෝ 13 One Sample Case 1: single population (one-sample) Suppose X=‘stroke’ from a population with mean μ=p and standard deviation σ= 퐩(ퟏ − 퐩). • What about σ= 퐩(ퟏ − 퐩) ?: 14 One Sample Case 1: single population (one-sample) Suppose X=‘stroke’ from a population with mean μ=p and standard deviation σ= 퐩(ퟏ − 퐩). Hypothesis Testing • Null hypothesis (H0): p=p0 • Alternative hypothesis (H1): – p p0 (two-sided test), or Why? – p < p0 (one-sided test), or – p > p0 (one-sided test) H0 pෝ−p0 • Test statistic: Z0 = ~ N(0, 1) pෝ(1−pෝ) n 15 CTS605A - Lecture Notes, Jonggyu Baek, PhD One Sample Case 1: single population (one-sample) Suppose X=‘stroke’ from a population with mean μ=p and standard deviation σ= 퐩(ퟏ − 퐩). Hypothesis Testing • Null hypothesis (H0): p=p0 • Alternative hypothesis (H1): – p p0 (two-sided test), or Why? – p < p0 (one-sided test), or From the CLT: σ – p > p (one-sided test) xത N(μ, ) 0 n H0 i.e., pෝ−p0 • Test statistic: Z = ~ N(0, 1) p(1−p) 0 pො N(p, ) pෝ(1−pෝ) n n 16 CTS605A - Lecture Notes, Jonggyu Baek, PhD One Sample Case 1: single population (one-sample) Suppose X=‘stroke’ from a population with mean μ=p and standard deviation σ= 퐩(ퟏ − 퐩). Hypothesis Testing H pෝ−p 0 • Test statistic: Z = 0 ~ N(0, 1) 0 pෝ(1−pෝ) n • Decision Rules by H1: Testing H0: p=p0 vs : H1 Reject H0 if: p p0 Z0 < Zα/2 or Z0 > Z1-α/2 p < p0 Z0 < Zα p > p0 Z0 > Z1-α 17 CTS605A - Lecture Notes, Jonggyu Baek, PhD One Sample • Case 1: single population (one-sample) Example (FHS): – Calculate 95% CI for the proportion of strokes in the population – Test whether this proportion is not different from 0.12=12% 18 CTS605A - Lecture Notes, Jonggyu Baek, PhD One Sample Case 1: single population (one-sample) Suppose X=‘stroke’ from a population with mean μ=p and standard deviation σ= 퐩(ퟏ − 퐩). Hypothesis Testing • Testing H0: p=p0=12%=0.12 19 CTS605A - Lecture Notes, Jonggyu Baek, PhD One Sample • Case 1: single population (one-sample) X: discrete (binary) variable (e.g., ‘stroke’) Statistical Inference about: p (Proportion of strokes in the population) ESTIMATION HYPOTHESIS TESTING (H0: ρ=ρ0) pෝ −p Point Estimate pො Test Statistic 0 Z0 = pෝ(1−pෝ) ) n Standard Error Decision rules pො(1 − pො) Reject H0 n against H1: (1-α)% CI pෝ(1−pෝ) μ μ0 Z0 < Zα/2 or Z0 > Z1-α/2 pො Z1-α/2 ( ) n μ < μ0 Z0<Zα μ > μ0 Z0>Z1-α 20 CTS605A - Lecture Notes, Jonggyu Baek, PhD Two Independent Samples • Case 2: two-independent populations (two-samples) Suppose Y=‘stroke’ and X=‘prevchd’ (both binary variables). There are two-independent populations, one with coronary heart disease (chd) and the other without chd. We want to compare proportions of strokes between those two populations. • p1 is the proportion of strokes in the population with CHD. • p2 is the proportion of strokes in the population without CHD. 21 CTS605A - Lecture Notes, Jonggyu Baek, PhD Two Independent Samples • Case 2: two-independent populations (two-samples) Y=‘stroke’ and X=‘prevchd’ (both binary variables) Statistical Inference about: p1-p2 (compare proportions of strokes between the two populations) ESTIMATION HYPOTHESIS TESTING (H0: p1-p2=0) Point Estimate pො -pො Test Statistic pෝ1−pෝ2 1 2 Z = s.e. Standard Error Decision rules (for ‘small’ n1, n2 use the pො1(1 − pො1) pො2(1 − pො2) Binomial distribution – + Reject H0 n1 n2 exact test) against H1: (1-α)% CI pො1−pො2 Z1-α/2 s.e.
Details
-
File Typepdf
-
Upload Time-
-
Content LanguagesEnglish
-
Upload UserAnonymous/Not logged-in
-
File Pages43 Page
-
File Size-