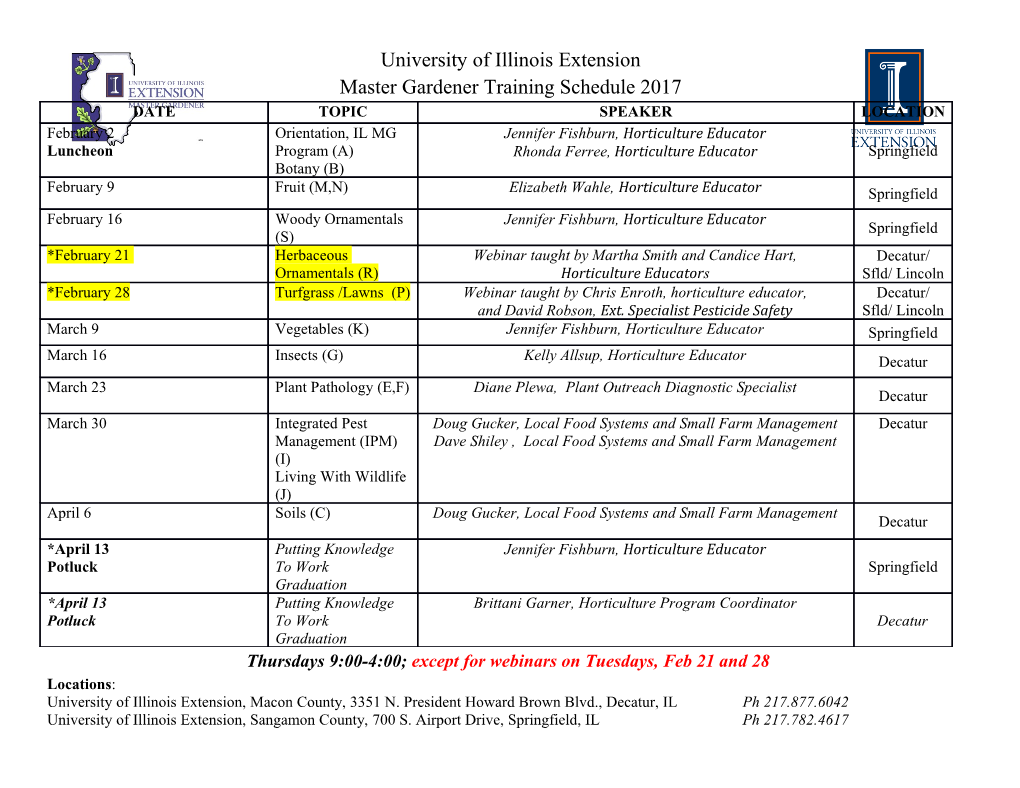
Transformations and Bayesian Estimation of Skewed and Heavy-Tailed Densities Dissertation Presented in Partial Fulfillment of the Requirements for the Degree Doctor of Philosophy in the Graduate School of The Ohio State University By Andrew Bean, B.A.,M.S. Graduate Program in Statistics The Ohio State University 2017 Dissertation Committee: Xinyi Xu, Co-Advisor Steven N. MacEachern, Co-Advisor Yoonkyung Lee Matthew T. Pratola c Copyright by Andrew Bean 2017 Abstract In data analysis applications characterized by large and possibly irregular data sets, nonparametric statistical techniques aim to ensure that, as the sample size grows, all unusual features of the data generating process can be captured. Good large- sample performance can be guaranteed in broad classes of problems. Yet within these broad classes, some problems may be substantially more difficult than others. This fact, long recognized in classical nonparametrics, also holds in the growing field of Bayesian nonparametrics, where flexible prior distributions are developed to allow for an infinite-dimensional set of possible truths. This dissertation studies the Bayesian approach to the classic problem of nonpara- metric density estimation, in the presence of specific irregularities such as heavy tails and skew. The problem of estimating an unknown probability density is recognized as being harder when the density is skewed or heavy tailed than when it is symmetric and light-tailed. It is more challenging problem for classical kernel density estimators, where the expected squared-error loss is higher for heavier tailed densities. It is also a more challenging problem in Bayesian density estimation, where heavy tails preclude the analytical treatment required to establish a large-sample convergence rate for the popular Dirichlet-Process (DP) mixture model. ii Our proposed approach addresses these features by incorporating a low-dimensional parametric transformation of the sample, estimated from the data, with the aim of set- ting up an easier density estimation problem on the transformed scale. This strategy was proposed earlier in combination with kernel density estimators, and we illustrate its usefulness in the Bayesian context. Further, we develop a set of transformations estimated in a way to ensure that the fastest proven convergence rate for the DP mixture is applicable to the transformed problem. The transformation-density estimation technique makes advantageous use of a parametric pre-analysis to address specific irregularities in the data generating pro- cess. Since the parametric stage is low-dimensional, and governed by a faster con- vergence rate, the asymptotic performance of the model is enhanced without slowing down the overall convergence rate. We consider other settings where this recipe for semiparametric analysis | with parametric sub-analyses designed to address specific irregularities, or to simplify the main nonparametric component of the analysis | might be beneficial. iii To my family. iv Acknowledgments This dissertation is the culmination of a six-year odyssey at Ohio State. I was \carried to Ohio in a swarm of bees," as the rock band The National put it. (Figu- ratively, of course, when it comes to the bees.) My studies have taken me far from my home state of Arizona. They have taken me far from friends and family, who, despite the distance, have continued to provide much-needed balance during my time in Ohio. In the end, Columbus too proved to be a wonderful home away from home. This is mostly thanks to the people I have met here, including the faculty and staff in the Department of Statistics, and friends, roommates, and classmates throughout the years. I will miss them, and will look back on these years with fondness. I would not have reached this point without the support of several people I want to thank individually. My advisors Xinyi Xu and Steve MacEachern are tremendous role models, both personally and as statisticians and researchers. They were unfailingly supportive and encouraging, even when my work did not proceed smoothly. It has been a privilege to work with them, and I look forward to continuing collaboration in the future. I thank Yoon Lee and Matt Pratola for serving on the committee for this dissertation. Their perspective and input on this work is invaluable. I have loved teaching statistics at Ohio State (loved everything but the grading, that is), and I became a better teacher during my time here thanks to Michelle Everson, Jonathan Baker, Laura Kubatko, and others. I also thank the faculty and staff of the v Mathematics and Computer Science Department at Colorado College; the wonderful teachers there inspired me to pursue my studies this far. Most of all, I am grateful to my family | my parents Jeff and Sydney, and my brother Owen | for their love and support as I worked towards this degree. I am privileged to have had parents and grandparents who gave me the opportunity to succeed at every level of my education. The only way to properly give thanks for this gift is to pass it on. I can only hope to do so with the same selflessness. Lastly, to Yi: the greatest fortune I've had during my time in Ohio is to have found a partner like you. The daily ups and downs of doctoral studies, the halls of Cockins: neither are romantic, but both seemed that way as we navigated them together. I look forward to writing our next chapters together. vi Vita August 3, 1987 . Born - San Francisco, CA, USA 2009 . .B.A. Mathematics, The Colorado College. 2012 . .M.S. Statistics, The Ohio State University. 2012-present . .Graduate Teaching Associate, The Ohio State University. Publications Research Publications A. Bean, X. Xu, S.N. MacEachern, \Transformations and Bayesian Density Estima- tion". Electronic Journal of Statistics, 10(2):3355-3373, Nov. 2016. Fields of Study Major Field: Statistics vii Table of Contents Page Abstract . ii Dedication . iv Acknowledgments . .v Vita......................................... vii List of Tables . xi List of Figures . xii 1. Introduction and Theoretical Motivation . .1 1.1 Parametric and Nonparametric Asymptotics by Example . .3 1.2 Asymptotic Properties of Bayesian Posteriors . .6 1.2.1 Parametric Bayesian Models . .7 1.2.2 Nonparametric Bayesian Models . .9 1.3 Outline of the Thesis . 12 2. Frequentist Transformation-Density Estimation . 14 2.1 Parzen-Type Kernel Density Estimators . 15 2.1.1 Asymptotic properties of the kernel density estimator . 16 2.2 Density Estimation with Transformations . 19 2.2.1 An L2 Criterion for Selecting Transformations . 20 2.3 Transformation Families . 27 2.3.1 Parametric transformation, nonparametric density estimation 27 2.3.2 Nonparametric transformation, nonparametric density esti- mation . 29 2.3.3 Other Transformation Families . 30 viii 3. Density Estimation using Dirichlet Process Mixtures . 35 3.1 Nonparametric Prior Construction . 35 3.1.1 The Dirichlet Process . 36 3.1.2 Dirichlet process mixtures . 38 3.2 Posterior computation for DP mixtures . 41 3.3 Performance of Dirichlet process mixtures for density estimation . 43 3.3.1 Measurements of accuracy for density estimation . 43 3.3.2 Constructions for the DP mixture prior, and finite-sample performance . 46 3.3.3 Asymptotic properties of DP mixtures . 48 4. Iterative Transformation and Bayesian Density Estimation . 53 4.1 Background . 53 4.2 Transformations . 56 4.2.1 Family of Transformations . 60 4.2.2 A Criterion for Estimating Transformation Parameters . 62 4.2.3 Iterative Transformation Selection . 66 4.3 Simulation Study . 67 4.3.1 Simulation Design . 67 4.3.2 Simulation Results . 69 4.4 An Application to BMI Modeling . 74 4.5 Discussion . 77 5. Heavy-Tailed Density Estimation Using Transformations and DP Mixtures 80 5.1 Motivation . 80 5.2 DP Mixture Asymptotics and Distribution Tails . 82 5.2.1 Convergence Rates for Sub-Gaussian Tails . 84 5.2.2 Characterization of Heavy-Tailed Distributions . 85 5.3 A Family of Transformations for Heavy-Tailed Densities . 86 5.3.1 Transformations to sub-Gaussian tails . 86 5.3.2 Skew-t cdf-inverse-cdf transformations . 89 5.3.3 Estimating Skew-t Transformation Parameters . 90 5.4 Simulations and Data Analysis . 98 5.4.1 Data Analysis . 99 5.4.2 Simulation Study . 107 5.5 Discussion . 110 ix 6. Extensions and Future Work . 120 6.1 Multivariate Density Estimation . 120 6.1.1 Transformations to Multivariate sub-Gaussianity . 123 6.1.2 Bayesian Analysis of Heavy-Tailed Time Series . 126 6.1.3 Median Regression with a Heavy-Tailed Error Distribution . 127 6.2 A Recipe for Efficient Semiparametric Analysis . 130 Appendices 131 A. Asymptotic Expansion of MISE for Kernel Estimates . 131 B. Estimating integrated squared second derivatives . 133 Bibliography . 136 x List of Tables Table Page 4.1 Ohio Family Health Survey (2008) sample sizes, divided into training and holdout samples. 77 5.1 Unemployment data: Log predictive scores (5.36) for transformation / DP mixture (5.2) predictive densities. 102 5.2 Acidity data: Log predictive scores (5.36) for transformation / DP mixture (5.2) predictive densities. 106 5.3 Griffin (2010) predictive scores for the acidity data. 107 xi List of Figures Figure Page 4.1 DPM density estimates (dashed lines) based on samples of size 100 for two examples of the two-piece distributions (the true densities are shown in solid black). The leftmost density is symmetric, but has t2 tails. The rightmost density has Gaussian tails, but is right-skewed. 54 4.2 Illustration of the Transformation-DPM technique. The heavy-tailed sample (left column, A1-A3) and skewed sample (right column, B1- B3) of figure 4.1 are transformed according to the symmetrizing and tail-shortening transformations of section 2. The DPM model is fit to the transformed samples in the bottom panels, then back-transformed to give the TDPM estimate on the original scale.
Details
-
File Typepdf
-
Upload Time-
-
Content LanguagesEnglish
-
Upload UserAnonymous/Not logged-in
-
File Pages161 Page
-
File Size-