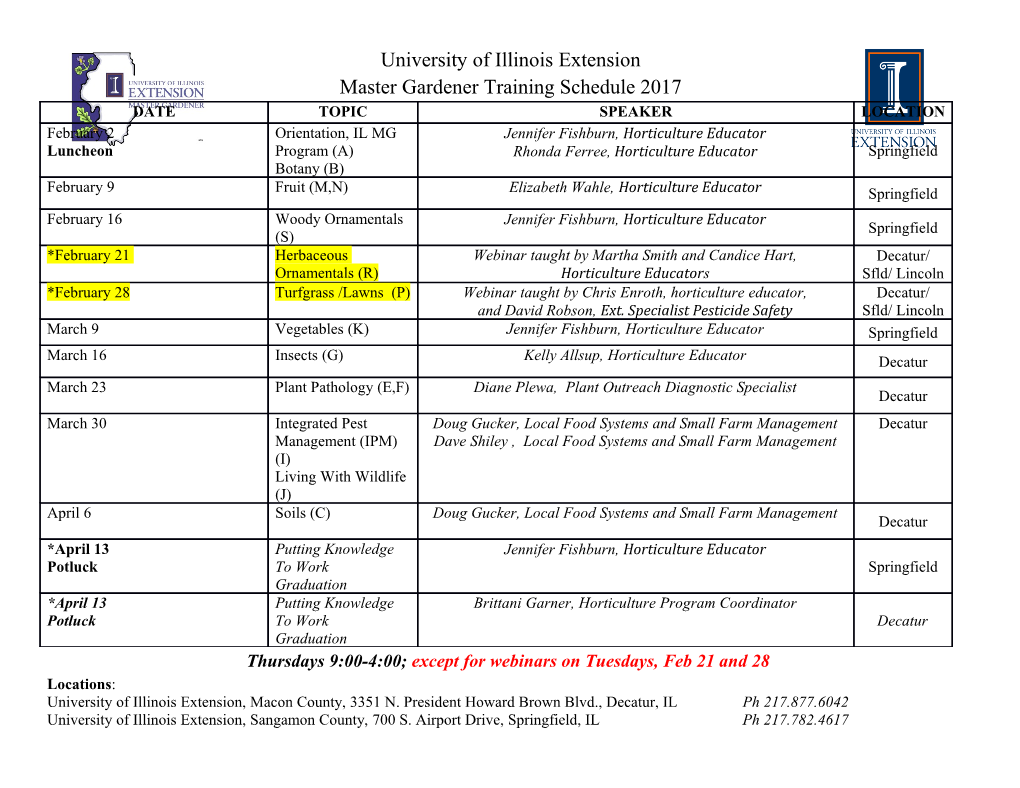
Characterizing the diffuse neutrino flux with the future KM3NeT/ARCA detector Charakterisierung des diffusen Neutrinoflusses mit dem zukunftigen¨ KM3NeT/ARCA-Neutrinoteleskop Der Naturwissenschaftlichen Fakultat¨ der Friedrich-Alexander-Universitat¨ Erlangen-Nurnberg¨ zur Erlangung des Doktorgrades Dr. rer. nat. vorgelegt von Thomas Gerhard Georg Heid aus Roth Als Dissertation genehmigt von der Naturwissenschaftlichen Fakultat¨ der Friedrich-Alexander-Universitat¨ Erlangen-Nurnberg¨ Tag der mundlichen¨ Prufung:¨ 13.12.2018 Vorsitzender des Promotionsorgans: Prof. Dr. Georg Kreimer Gutachter: Prof. Dr. Gisela Anton Dr. Paschal Coyle Contents 1 Introduction 6 2 Neutrino physics8 2.1 Neutrino properties...................................8 2.2 Neutrino production...................................8 2.2.1 Neutrinos from astrophysical sources.....................9 2.2.2 Neutrinos produced in the Earth’s atmosphere................. 11 2.3 Neutrino Oscillation................................... 12 2.4 Neutrino interaction................................... 13 2.4.1 Interactions of neutrinos of all kinds of flavor in the neutral current..... 15 2.4.2 Interaction of νe in the charged current..................... 15 2.4.3 Interaction of νµ in the charged current..................... 15 2.4.4 Interactions of ντ in the charged current.................... 16 3 KM3NeT: A worker for neutrino astronomy 18 3.1 KM3NeT........................................ 18 3.2 Future prospects of neutrino astronomy........................ 20 4 Detector Simulation 21 5 Atmospheric muons and neutrinos in KM3NeT 24 5.1 Cosmic Rays...................................... 24 5.2 Simulation........................................ 29 5.2.1 CORSIKA................................... 29 5.3 Comparison of simulations............................... 36 5.4 Atmospheric neutrino self-veto............................. 44 6 Event Identification 49 6.1 Definitions towards the goodness of classification................... 49 6.2 Calculation of features................................. 50 6.2.1 Spacial distribution............................... 50 6.2.2 Time residual distribution........................... 51 6.2.3 Output of reconstruction............................ 55 6.3 Definition of classes................................... 57 6.4 Identification neutrino events with machine learning algorithms............ 61 6.4.1 Neural network................................. 61 6.5 Training process..................................... 62 6.5.1 Event selection................................. 63 6.5.2 Event preprocessing.............................. 65 6.5.3 Balancing the dataset.............................. 67 4 5 6.5.4 Training of the neural network......................... 69 6.6 Application of trained classifier............................. 70 6.7 Results of event identification.............................. 70 6.7.1 Performance criteria.............................. 70 6.7.2 Event distributions............................... 72 6.7.3 Variance of classifier.............................. 75 6.8 Further Applications.................................. 78 6.9 Outlook......................................... 79 7 Flavor Composition 80 7.1 Analysis......................................... 80 7.1.1 Event selection and identification....................... 81 7.1.2 Pseudo experiments.............................. 83 7.1.3 Fit process................................... 84 7.1.4 Construction of confidence intervals...................... 84 7.1.5 Fit correction.................................. 87 7.2 Results.......................................... 89 7.2.1 Studies on influences to the fit process..................... 90 7.2.2 Not included in this study........................... 92 7.3 Log-likelihood Ratio Tests............................... 93 7.4 Comparison to sensitivities for IceCube Gen2..................... 95 7.5 Conclusion....................................... 96 8 Conclusion 97 Zusammenfassung 100 Appendices 103 A Details on atmospheric particle simulation 104 A.1 Compile options..................................... 104 A.2 Run time options.................................... 105 A.3 Atmospheric models.................................. 105 A.4 MUPAGE........................................ 116 A.5 Comparison of values characterizing atmospheric muon bundles........... 117 A.5.1 SIBYLL.................................... 117 A.5.2 EPOS...................................... 118 A.5.3 QGSJET01................................... 119 A.5.4 QGSJETII................................... 120 A.5.5 SIBYLL.................................... 121 A.5.6 Comparison of hadronic interaction models.................. 122 A.5.7 Comparison of cosmic-ray flux models.................... 123 B Atmospheric self-veto 127 C EventIdentification 128 C.1 Features......................................... 128 Bibliography......................................... 131 Appendix 137 Chapter 1 Introduction During 100 years after Victor Hess discovered the cosmic rays in 1912 [1], the measurements done with numerous experiments increased the knowledge about features of the cosmic ray flux. The observable energy interval has been extended up to highest energies of 1020 eV [2]. Nevertheless, more and more questions have arisen. The origin of cosmic-ray particles is not clear up to now. As the cosmic-ray particles, discovered by Hess, are charged particles, they are deflected by magnetic fields on their journey to the Earth. Therefore, their path and origin are hidden. However, there are two unique messengers which are not deflected by magnetic fields. They point back to the place of their creation. Firstly, there are the γ-ray photons, which can be observed by experiments like H.E.S.S. [3]. Sky maps are full of sources found by these experiments, but the production mechanism is not yet fully understood as γ-rays can be produced by electro-magnetic as well as hadronic processes. Another messenger combines the benefits of the straight path from the source to the observer and is produced in hadronic processes only, therefore it can resolve the ”electromagnetic-versus-hadronic” puzzle. The particle sought is the neutrino, which was first postulated by Pauli in 1930 [4]. Neutrinos do not interact via strong force nor electro-magnetic force, so the weak interaction is the only production and detection mechanism. The neutrino can traverse dense regions in astrophysical objects, which is not possible at the same extent for γ-rays due to a much shorter interaction length. Neutrinos will enable mankind to look into objects which were unreachable up to now. Though neutrinos are perfect probes to study the universe, they are not easy to detect. The interaction cross section is very small. Additionally, the flux in the interesting energy interval of galactic and extra-galactic sources is very low on Earth, of the order of 10 interacting neutrinos above 1 PeV per year in cubic-kilometer sized detectors. Only a few years ago, this new ”light” from the sky pushed the young field of neutrino astronomy when the astrophysical neutrino flux was detected by the IceCube collaboration [5]. In the course thereof cubic-kilometer-sized detectors are planned and built or were built. Another collaboration facing this challenge is KM3NeT (km3-Neutrino Telescope), a European collaboration for neutrino research facilities [6]. Its experiment ARCA (Astroparticle Research with Cosmics in the Abyss) is optimized for galactic neutrino point-like sources. It is located deep in the Mediterranean Sea near Sicily and is still growing. However, as the neutrino flux is so small, up to now no point- like source was detected. Even if single neutrino sources are too weak to be detected, the diffuse flux emitted collectively by all sources is strong enough as seen with the discovery of IceCube [5]. Nevertheless, most characteristic quantities are still hidden behind the sparse data. Most analyses aim at measuring the total neutrino flux or one single-flavor flux. The expectation of the ratio between different neutrino flavors, the so-called neutrino flavor composition, has not been unraveled up to now. Analyses, like the IceCube analysis from 2015 [7], show a wide range of possible values. This work is one step towards a better understanding of neutrino sources via solving the question of neutrino flavor composition. The degree to which ARCA can characterize the neutrino flux depends 6 7 significantly on the possibility to separate neutrinos according to their flavor. Furthermore, the con- fidence in the omnipresent background of atmospheric particles and its rejection directly influences the view on the flavor composition. In this thesis, these two conditions are examined and optimized to work out the first estimate on the sensitivity of ARCA to the neutrino flavor composition. For this purpose, a new method to separate events into several classes of event topologies is developed. The subsamples are further used to evaluate the sensitivity of KM3NeT/ARCA to the neutrino flavor composition. This is done with techniques of spectral fitting. This thesis starts with a brief introduction to neutrino astronomy. Chapter2 summarizes the physics of neutrinos, starting from their production at astrophysical sites, proceeding with the neutrino’s journey being subject to neutrino oscillations and ending with the interaction of neutrinos inside the detector. The detection principle and its implementation are outlined in Chapter3. Standard simulation algo- rithms necessary for the event identification and flavor composition
Details
-
File Typepdf
-
Upload Time-
-
Content LanguagesEnglish
-
Upload UserAnonymous/Not logged-in
-
File Pages138 Page
-
File Size-