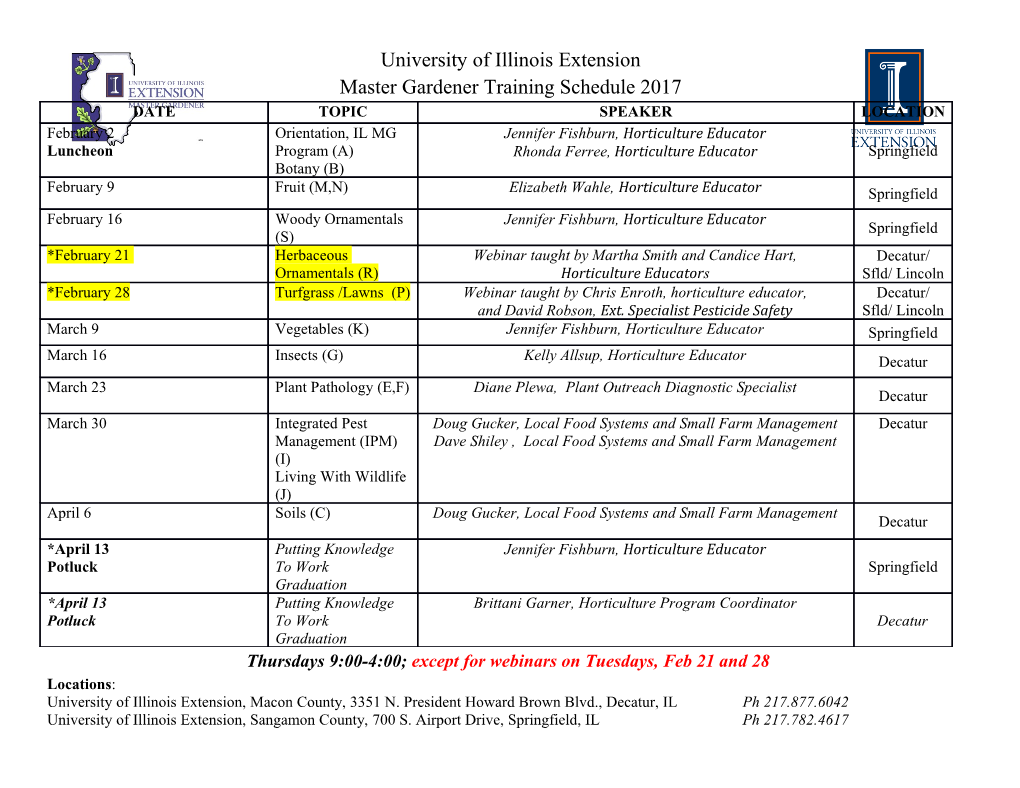
CS 2750 Machine Learning Lecture 7 Linear regression (cont.) Linear methods for classification Milos Hauskrecht [email protected] 5329 Sennott Square CS 2750 Machine Learning Coefficient shrinkage • The least squares estimates often have low bias but high variance • The prediction accuracy can be often improved by setting some coefficients to zero – Increases the bias, reduces the variance of estimates • Solutions: – Subset selection – Ridge regression – Principal component regression • Next: ridge regression CS 2750 Machine Learning 1 Ridge regression • Error function for the standard least squares estimates: = 1 − T 2 J n (w ) ∑ (yi w x i ) n i=1,..n * = 1 − T 2 • We seek: w arg min ∑ (yi w x i ) w n i=1,..n • Ridge regression: = 1 − T 2 + λ 2 J n (w ) ∑ (yi w x i ) w n i=1,..n •Where d 2 = 2 λ ≥ w ∑ wi and 0 i =0 • What does the new error function do? CS 2750 Machine Learning Ridge regression • Standard regression: = 1 − T 2 J n (w ) ∑ (yi w x i ) n i=1,..n • Ridge regression: = 1 − T 2 + λ 2 J n (w ) ∑ (yi w x i ) w n i=1,..n d 2 = 2 •w ∑ wi penalizes non-zero weights with the cost = i 0 proportional to λ (a shrinkage coefficient) • If an input attribute x j has a small effect on improving the error function it is “shut down” by the penalty term • Inclusion of a shrinkage penalty is often referred to as regularization CS 2750 Machine Learning 2 Supervised learning = Data: D { d 1 , d 2 ,.., d n } a set of n examples =< > di xi , yi xi is input vector, and y is desired output (given by a teacher) Objective: learn the mapping f : X → Y ≈ = s.t. yi f (xi ) for all i 1,.., n Two types of problems: • Regression: Y is continuous Example: earnings, product orders company stock price • Classification: Y is discrete Example: temperature, heart rate disease Today: binary classification problems: CS 2750 Machine Learning Binary classification • Two classes Y = {0,1} • Our goal is to learn to classify correctly two types of examples – Class 0 – labeled as 0, – Class 1 – labeled as 1 • We would like to learn f : X → { 0,1} • Zero-one error (loss) function 1 f (x , w ) ≠ y Error (x , y ) = i i 1 i i = 0 f (x i , w ) y i • Error we would like to minimize: E ( x, y ) (Error 1 (x, y)) • First step: we need to devise a model of the function CS 2750 Machine Learning 3 Discriminant functions • One convenient way to represent classifiers is through – Discriminant functions • Works for binary and multi-way classification • Idea: – For every class i = 0,1, …k define a function g i (x) mapping X → ℜ – When the decision on input x should be made choose the class with the highest value of g i (x) • So what happens with the input space? Assume a binary case. CS 2750 Machine Learning Discriminant functions 2 1.5 1 ≥ 0.5 g1 (x) g 0 (x) 0 -0.5 -1 -1.5 -2 -2 -1.5 -1 -0.5 0 0.5 1 1.5 CS 2750 Machine Learning 4 Discriminant functions 2 1.5 1 ≥ 0.5 g1 (x) g 0 (x) 0 -0.5 -1 ≤ g1 (x)≤ g 0 (x) -1.5 g1 (x) g 0 (x) -2 -2 -1.5 -1 -0.5 0 0.5 1 1.5 CS 2750 Machine Learning Discriminant functions 2 1.5 1 ≥ 0.5 g1 (x) g 0 (x) g (x) ≥ g (x) 0 1 0 -0.5 -1 g (x) ≤ g (x) 1 ≤ 0 -1.5 g1 (x) g 0 (x) -2 -2 -1.5 -1 -0.5 0 0.5 1 1.5 CS 2750 Machine Learning 5 Discriminant functions • Define decision boundary. 2 1.5 1 ≥ 0.5 g1 (x) ≥g 0 (x) g1 (x) g 0 (x) 0 -0.5 = g1 (x) g 0 (x) -1 ≤ g1 (x) ≤g 0 (x) -1.5 g1 (x) g 0 (x) -2 -2 -1.5 -1 -0.5 0 0.5 1 1.5 CS 2750 Machine Learning Quadratic decision boundary Decision boundary 3 2.5 2 1.5 1 ≥ g1 (x) g 0 (x) 0.5 0 -0.5 g (x) ≤ g (x) -1 1 0 = g1 (x) g 0 (x) -1.5 -2 -2 -1.5 -1 -0.5 0 0.5 1 1.5 CS 2750 Machine Learning 6 Logistic regression model • Defines a linear decision boundary • Discriminant functions: = T = − T g1 (x) g (w x) g 0 (x) 1 g (w x) − • where g (z) = 1 /(1 + e z ) - is a logistic function = T = T f (x, w ) g1 (w x) g (w x) 1 Logistic function w0 ∑ x1 w1 z f (x, w ) w x 2 Input vector 2 w x d xd CS 2750 Machine Learning Logistic function 1 function g (z) = (1 + e − z ) • also referred to as a sigmoid function • Replaces the threshold function with smooth switching • takes a real number and outputs the number in the interval [0,1] 1 0.9 0.8 0.7 0.6 0.5 0.4 0.3 0.2 0.1 0 -20 -15 -10 -5 0 5 10 15 20 CS 2750 Machine Learning 7 Logistic regression model • Discriminant functions: = T = − T g1 (x) g (w x) g 0 (x) 1 g (w x) − z • Where g (z) = 1 /(1 + e ) - is a logistic function • Values of discriminant functions vary in [0,1] – Probabilistic interpretation = = = = T f (x, w ) p( y 1 | w, x) g1 (x) g (w x) 1 w0 ∑ p(y =1| x,w) x1 w1 z w x 2 Input vector 2 w x d xd CS 2750 Machine Learning Logistic regression • Instead of learning the mapping to discrete values 0,1 f : X →{0,1} •we learn a probabilistic function f : X →[0,1] –where f describes the probability of class 1 given x f (x, w) = p( y = 1| x, w) Note that: p( y = 0 | x, w) = 1− p( y = 1| x, w) • Transformation to discrete class values: If p ( y = 1 | x ) ≥ 1 / 2 then choose 1 Else choose 0 CS 2750 Machine Learning 8 Linear decision boundary • Logistic regression model defines a linear decision boundary • Why? • Answer: Compare two discriminant functions. = • Decision boundary: g1 (x) g 0 (x) • For the boundary it must hold: − T go (x) = 1 g(w x) = log log T 0 g1(x) g(w x) exp− (wTx) g (x) 1+ exp− (wTx) log o = log = logexp− (wTx) = wTx = 0 1 g1(x) 1+ exp− (wTx) CS 2750 Machine Learning Logistic regression model. Decision boundary • LR defines a linear decision boundary Example: 2 classes (blue and red points) Decision boundary 2 1.5 1 0.5 0 -0.5 -1 -1.5 -2 -2 -1.5 -1 -0.5 0 0.5 1 1.5 2 CS 2750 Machine Learning 9 Logistic regression: parameter learning. Likelihood of outputs • Let =< > µ = = = = T D i x i , y i i p(yi 1| xi ,w) g(zi ) g(w x) • Then n n y − = = = µ i − µ 1 yi L(D, w) ∏ P( y yi | x i , w) ∏ i (1 i ) i=1 i=1 • Find weights w that maximize the likelihood of outputs – Apply the log-likelihood trick The optimal weights are the same for both the likelihood and the log-likelihood n n y − y − = µ i − µ 1 yi = µ i − µ 1 yi = l(D, w ) log ∏ i (1 i ) ∑ log i (1 i ) i=1 i=1 n = µ + − − µ ∑ y i log i (1 y i ) log( 1 i ) i =1 CS 2750 Machine Learning Logistic regression: parameter learning • Log likelihood n = µ + − − µ l(D, w ) ∑ yi log i (1 yi ) log(1 i ) i=1 • Derivatives of the loglikelihood ∂ n Nonlinear in weights !! − l(D, w ) = − x ( y − g (z )) ∂ ∑ i, j i i w j i=1 n n ∇ − = − − T = − − w l(D, w) ∑ xi ( yi g(w xi )) ∑ xi ( yi f (w, xi )) i=1 i=1 • Gradient descent: ( k ) ← ( k −1) − α ∇ − w w (k ) [ l(D, w )] | ( k −1) w w n ( k ) ← ( k −1) + α − (k −1) w w (k )∑ [ yi f (w , x i )]x i i=1 CS 2750 Machine Learning 10 Logistic regression. Online gradient descent • On-line component of the loglikelihood − = µ + − − µ J online ( D i , w ) y i log i (1 y i ) log( 1 i ) • On-line learning update for weight w J online (D k , w ) ( k ) ← ( k −1) − α ∇ w w (k ) [J (D , w )] | ( k −1) w online k w =< > • ith update for the logistic regression and Dk xk , yk (i ) ← ( k −1) + α − ( k −1) w w (k )[ yi f (w , x k )]x k CS 2750 Machine Learning Online logistic regression algorithm Online-logistic-regression (D, number of iterations) = initialize weights w (w0 , w1, w2 Kwd ) for i=1:1: number of iterations =< > do select a data point D i x i , y i from D set α =1/i update weights (in parallel) ← + α − w w (i)[ yi f (w, x i )]x i end for return weights w CS 2750 Machine Learning 11 Online algorithm. Example. CS 2750 Machine Learning Online algorithm. Example. CS 2750 Machine Learning 12 Online algorithm. Example. CS 2750 Machine Learning 13.
Details
-
File Typepdf
-
Upload Time-
-
Content LanguagesEnglish
-
Upload UserAnonymous/Not logged-in
-
File Pages13 Page
-
File Size-