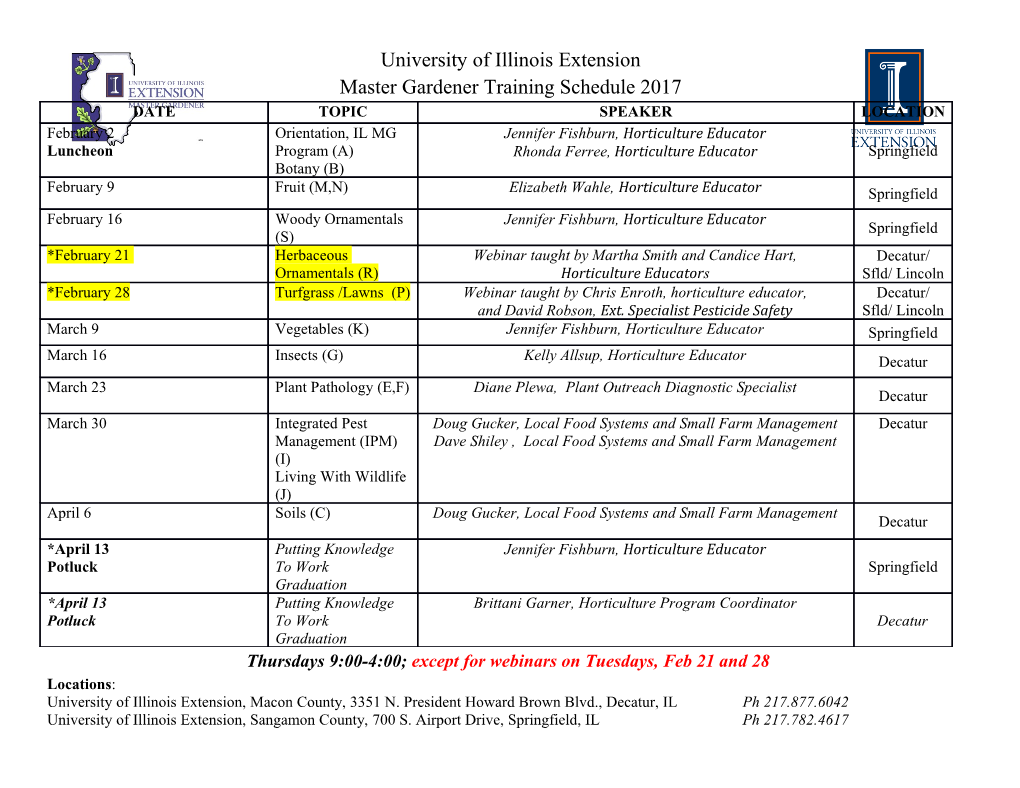
EE290T: Advanced Reconstruction Methods for Magnetic Resonance Imaging Martin Uecker Introduction Topics: I Image Reconstruction as Inverse Problem I Parallel Imaging I Non-Cartestian MRI I Subspace Methods I Model-based Reconstruction I Compressed Sensing Tentative Syllabus I 01: Jan 27 Introduction I 02: Feb 03 Parallel Imaging as Inverse Problem I 03: Feb 10 Iterative Reconstruction Algorithms I {: Feb 17 (holiday) I 04: Feb 24 Non-Cartesian MRI I 05: Mar 03 Nonlinear Inverse Reconstruction I 06: Mar 10 Reconstruction in k-space I 07: Mar 17 Reconstruction in k-space I {: Mar 24 (spring recess) I 08: Mar 31 Subspace methods I 09: Apr 07 Model-based Reconstruction I 10: Apr 14 Compressed Sensing I 11: Apr 21 Compressed Sensing I 12: Apr 28 TBA Today I Review I Non-Cartesian MRI I Non-uniform FFT I Project 2 Direct Image Reconstruction I Assumption: Signal is Fourier transform of the image: Z ~ s(t) = d~x ρ(~x)e−i2π~x·k(t) I Image reconstruction with inverse DFT ky kx ) ) sampling iDFT ~ R t ~ image k(t) = γ 0 dτ G(τ) k-space Requirements I Short readout (signal equation holds for short time spans only) I Sampling on a Cartesian grid ) Line-by-line scanning α echo radio measurement time: slice TR ≥ 2ms read 2D: N ≈ 256 ) seconds phase 3D: N ≈ 256 × 256 ) minutes TR ×N 2D MRI sequence Poisson Summation Formula Periodic summation of a signal f from discrete samples of its Fourier transform f^: 1 1 X X 1 l 2πi l t f (t + nP) = f^( )e P P P n=−∞ l=−∞ No aliasing ) Nyquist criterium l For MRI: P = FOV ) k-space samples are at k = FOV I Periodic summation from discrete Fourier samples on grid I Other positions can be recovered by sinc interpolation (Whittaker-Shannon formula) image k-space I Other positions can be recovered by sinc interpolation (Whittaker-Shannon formula) image k-space I Periodic summation from discrete Fourier samples on grid image k-space I Periodic summation from discrete Fourier samples on grid I Other positions can be recovered by sinc interpolation (Whittaker-Shannon formula) Nyquist-Shannon Sampling Theorem Theorem 1: If a function f (t) contains no frequencies higher than W cps, it is completely determined by giving its ordinates at a series of points spaced 1=2W seconds apart.1 I Band-limited function I Regular sampling I Linear sinc-interpolation 1. CE Shannon. Communication in the presence of noise. Proc Institute of Radio Engineers; 37:10{21 (1949) Fast Fourier Transform (FFT) Algorithms Discrete Fourier Transform (DFT): N−1 N−1 X 2π N ^ i N kn DFTk ffngn=0 := fk = e fn for k = 0; ··· ; N − 1 n=0 Matrix multiplication: O(N2) Fast Fourier Transform Algorithms: O(N log N) Cooley-Tukey Algorithm Decomposition of a DFT: N−1 N N−1 X kn DFTk ffngn=0 = (ξN ) fn n=0 N1−1 N2−1 X k1n1 k2n1 X k2n2 = (ξN1 ) (ξN ) (ξN2 ) fn1+n2N1 n1=0 n2=0 n oN1−1 = DFT N1 (ξ )k2n1 DFT N2 ff gN2−1 k1 N k2 n1+n2N1 n2=0 n1=0 I Recursive decomposition to prime-sized DFTs ) Efficient computation for smooth sizes (efficient algorithms available for all other sizes too) Parallel MRI: Iterative Algorithms Signal equation: Z ~ −i2π~x·~k si (k) = d~x ρ(~x) ci (~x)e V | {z } encoding functions Discretization: A = Pk FC A has size 2562 × (8 × 2562) ) Iterative methods built from matrix-vector products sensitivities Ax, AH y Landweber Iteration Gradient descent: 1 φ(x) = kAx − yk2 2 2 rφ(x) = AH (Ax − y) Iteration rule: H H xn+1 = xn − µA (Axn − y) with µkA Ak ≤ 1 H H = (I − µA A)xn − A y Explicit formula for x0 = 0: n−1 X H j H xn = (I − µA A) µA y j=0 Projection Onto Convex Sets (POCS) Iterative projection onto convex sets: yn+1 = PB PAyn A B Problems: slow, not stable, A \ B = ? POCSENSE Iteration: y^n+1 = PC Py y^n (multi-coil k-spacey ^) Projection onto sensitivities: H −1 PC = FCC F (normalized sensitivities) Projection onto data y: Py y^ = My + (1 − M)^y AA Samsonov, EG Kholmovski, DL Parker, and CR Johnson. POCSENSE: POCS-based reconstruction for sensitivity encoded magnetic resonance imaging. Magn Reson Med, 52:1397{1406, 2004. Krylov Subspace Methods Krylov subspace: n Kn = spani=0···n fT bg ) Repeated application of T . Landweber, Arnoldi, Lanczos, Conjugate gradients, ... Conjugate Gradients T symmetric (or Hermitian) Initialization: r0 = b − Tx0 d0 = r0 Iteration: qi ( Tdi 2 jri j α = H R ri qi xi+1 ( xi + αdi ri+1 ( ri − αqi 2 jri+1j β = 2 jri j di+1 ( ri+1 + βdi Conjugate Gradients on Normal Equations Normal equations: H H 2 A Ax = A y , x = argminx kAx − yk2 Tikhonov: H H 2 2 A A + αI x = A y , x = argminx kAx − yk2 + αkxk2 CGNE: Tx = b with: T = AH A b = AH y Implementation repeat FFT P IFFT FFT P IFFT sum channels point-wise mult. with conjugate sensitivities data flow inverse FFT sampling mask or projection onto data multi-dimensional FFT point-wise multiplication with sensitivities distribute image Conjugate Gradient Algorithm vs Landweber conjugate gradients Landweber 2 1 1: 1:414 1:732 0 2: Project 1: Iterative SENSE Project: Implement and study Cartesian iterative SENSE I Tools: Matlab, reconstruction toolbox, python, ... I Deadline: Feb 24 I Hand in: Working code and plots/results with description. I See website for data and instructions. Project 1: Iterative SENSE Step 1: Implement Model A = PFC AH = C H F −1PH Hints: I Use unitary and centered (fftshift) FFT kFxk2 = kxk2 I Implement undersampling as a mask, store data with zero H I Check hx; Ayi = A x; y for random vectors x; y Project 1: Iterative SENSE Step 2: Implement Reconstruction Landweber (gradient descent)1 H xn+1 = xn + αA (y − Axn) Conjugate gradient algorithm2 Step 3: Experiments I Noise, errors, and convergence speed I Different sampling I Regularization 1. L Landweber. An iteration formula for Fredholm integral equations of the first kind. Amer J Math; 73:615{624 (1951) 2. MR Hestenes and E Stiefel. Methods of conjugate gradients for solving linear systems. J. Ref. N.B.S. 49:409{436 (1952) Today I Review I Non-Cartesian MRI I Non-uniform FFT I Project 2 Parallel Imaging as Inverse Problem Model: Signal from multiple coils (image ρ, sensitivities cj ): Z −i2π~x·~k(t) sj (t) = d~x ρ(~x)cj (~x)e + nj (t) Ω Assumptions: I Image is square-integrable function ρ 2 L2(Ω; C) I Additive multi-variate Gaussian white noise n Problem: Find best approximate/regularized solution in L2(Ω; C). Ω Non-Cartesian Trajectories Examples: Cartesian radial spiral Non-Cartesian Trajectories Practical issues: I Long readout: blurring, artifacts I Imperfect gradient waveforms (e.g. delays, eddy currents) I Efficient implementation of the reconstruction algorithm Spiral Long readout: I Efficient! ? I T2 -Relaxation ) blurring I Off-resonance Spiral Signal equation: Z itγ∆B (~x) −t=T ?(~x) −2πi~k~x y(t) = dx ρ(~x)e 0 e 2 e Ω Off-resonance: itγ∆B0(~x) jt − tTE j ∆γB0 ) e ≈ const Relaxation: ? ? −t=T2 jt − tTE j T2 ) e ≈ const Spiral Signal equation: Z itγ∆B (~x) −t=T ?(~x) −2πi~k~x y(t) = dx ρ(~x)e 0 e 2 e Ω Off-resonance: itγ∆B0(~x) jt − tTE j ∆γB0 ) e ≈ const Relaxation: ? ? −t=T2 jt − tTE j T2 ) e ≈ const Radial π I Not efficient (Nyquist: 2 × spokes vs. Cartesian lines) I Motion robustness I High tolerance to undersampling I Continuous update possible Radial - Undersampling Artifacts Left to right: 96, 48, 24 radial spokes Forward Problem Signal equation: Z ~ −2πikl~x yl = d~x ρ(~x)e Ω Non-Cartesian sample positions: kl Discretization: ρ(~x) pixel values at grid positions ~x ~ X −2πikl~x yl = ρ(~x)e ~x ) non-uniform DFT from grid to non-Cartesian positions. Inverse Non-Uniform Fourier Transform Forward model: Z ~ ikl~x yl = d~x ρ(~x)e Inverse problem: y = Pk Fρ = Aρ Best-approximation (least-squares + minimum-norm): −1 H H x = limα!0A AA + αI y Non-uniform FFT Fourier transform at arbitray (non-Cartesian) positions kl Z −2πikl x yl = dx f (x)e DFT at arbitray (non-Cartesian) positions kl N−1 k n X −2πi l yl = xne N n=0 Fast computation: nuFFT Interpolation to Non-Cartesian Data I Periodic extension: FFT yields Fourier transform on grid I Values at k-space positions recovered by sinc interpolation Whittaker-Shannon formula: 1 X t − nT sin(πx) f (t) = f (nT ) sinc( ) sinc(x) = T πx n=−∞ Approximation of the Interpolation Computation: N=2−1 X t − nT f (t) = f (nT ) kern( ) T n=−N=2 I Sinc interpolation: not practical I Compactly supported kernels: truncated Gaussian, Kaiser-Bessel Kernel with compact support: kern(x) = 0 for jxj >= wT ) Sum has only 2 ∗ w terms ) Efficient computation Approximation of the Interpolation Computation (2D): N=2−1 M=2−1 X X s − nS t − nT f (s; t) = f (nS; mT ) kern( ) kern( ) S T n=−N=2 m=−M=2 Approximation of the Interpolation Computation (2D): N=2−1 M=2−1 X X s − nS t − nT f (nS; mT ) kern( ) kern( ) S T n=−N=2 m=−M=2 Isotropic kernel: 0 1 N=2−1 M=2−1 s 2 2 X X s − nS t − nT f (nS; mT ) kern + @ S T A n=−N=2 m=−M=2 Approximation of the Interpolation I Sinc interpolation: not practical I Finite kernel: Kaiser-Bessel interpolation I Oversampling to reduce approximation errors stop pass stop Approximation of the Interpolation I Sinc interpolation: not practical I Finite kernel: Kaiser-Bessel interpolation I Oversampling to reduce approximation errors stop pass stop Approximation of the Interpolation I Sinc interpolation: not practical I Finite kernel: Kaiser-Bessel interpolation I Oversampling to reduce approximation errors stop pass stop Approximation of the Interpolation I Sinc interpolation: not practical I Finite kernel: Kaiser-Bessel interpolation I Oversampling to reduce approximation errors stop pass stop Approximation of the Interpolation I Sinc interpolation: not practical I Finite kernel: Kaiser-Bessel interpolation I Oversampling to reduce approximation
Details
-
File Typepdf
-
Upload Time-
-
Content LanguagesEnglish
-
Upload UserAnonymous/Not logged-in
-
File Pages68 Page
-
File Size-