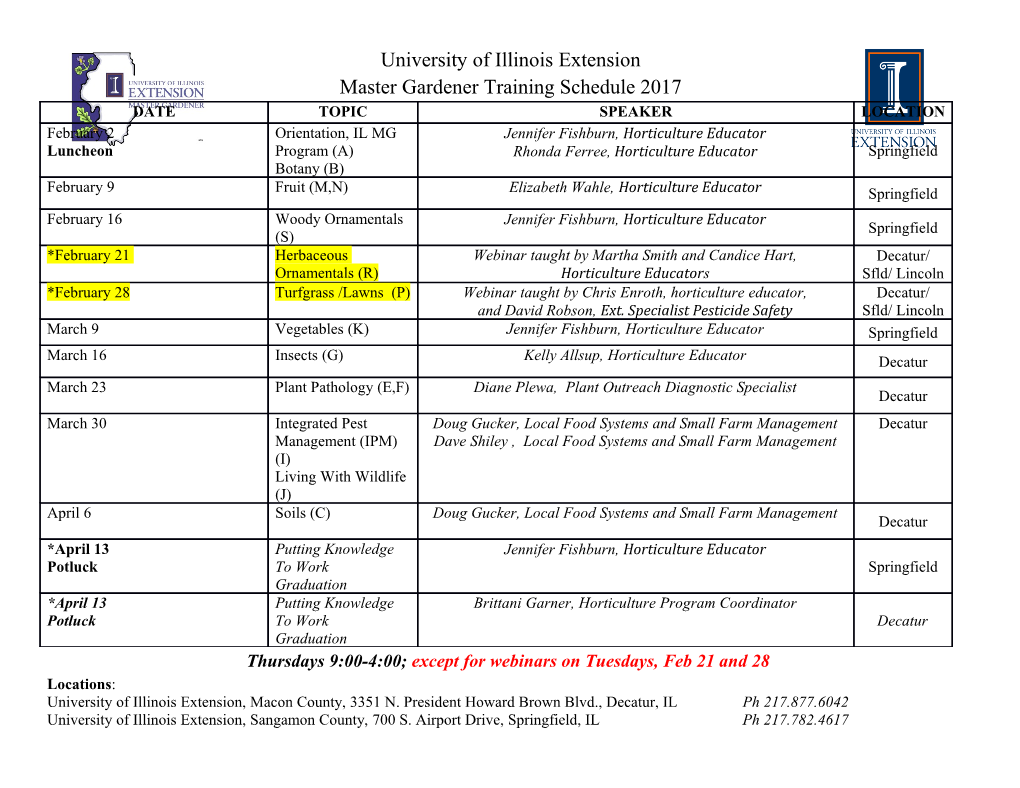
WSEAS TRANSACTIONS on BIOLOGY and BIOMEDICINE Li-Fei Huang, Jen-Jen Lin The estimation of the m parameter of the Nakagami distribution Li-Fei Huang Jen-Jen Lin Ming Chuan University Ming Chuan University Dept. of Applied Stat. and Info. Science Dept. of Applied Stat. and Info. Science 5 Teh-Ming Rd., Gwei-Shan Taoyuan City 5 Teh-Ming Rd., Gwei-Shan Taoyuan City Taiwan Taiwan [email protected] [email protected] Abstract: This paper introduces the Nakagami-m distribution which is usually used to simulate the ultrasound image. The gamma distribution is used to derive the moment estimator and the maximum likelihood estimator because the Nakagami distribution has no moment generation function and too complicate likelihood function. The moment estimator of m is normally distributed with a smaller bias and a larger standard deviation. The second order maximum likelihood estimator of m is also normally distributed with a larger bias and a smaller standard deviation. The confidence interval for the ratio of medians from two independent distributions of Nakagami-m estimators is constructed. The moment estimator provides a quick understanding about the m parameter, while the second order maximum likelihood estimator provides a full understanding about the m parameter. Key–Words: The Nakagami distribution, The moment estimator, The maximum likelihood estimator, The distribu- tion of the Nakagami-m, The ratio of medians. 1 Introduction agami distribution is as follows. 2 m m 2m−1 − m n2 f(n) = ( ) n e Ω The Nakagami distribution is usually used to simu- Γ(m) Ω late the ultrasound image. Smolikova et al. say that Huang and Johnson [3] provide theorems to con- analysis of backscatter in the ultrasound echo enve- struct a confidence interval for ratio of percentiles lope, in conjunction with ultrasound B-scans, can pro- from two independent distributions. vide important information for tissue characterization and pathology diagnosis[6]. Pavlovic et al. present the joint probability density function (PDF) and PDF 2 The estimators of Nakagami-m of maximum of ratios mu(1) = R-1/r(1) and mu(2) = R-2/r(2) for the cases where R-1, R-2, r(1), and r(2) 2.1 The moment estimator are Rayleigh, Rician, Nakagami-m., and Weibull dis- ∼ 2 1=2 tributed random variables[4]. Agrawal and Karmeshu If N Nakagami(m, Ω), let G = N . Then n = g 2 ∼ Ω propose a new composite probability distribution, and G = N gamma(m, m ). i. e. Nakagami-generalized inverse Gaussian dis- dn 1 − 1 tribution (NGIGD) with four parameters[1]. Chen jJj = j j = j g 2 j dg 2 applies order statistics to analyze the performance of ordered selection combining schemes with differ- 2 m m 2m−1 − m n2 f(n) = ( ) n e Ω ent modulation receptions operating in Nakagami-m Γ(m) Ω fading environments and all the results are validated 2 m m 2m−1 − m g 1 − 1 by comparing the special case Rayleigh distribution ) f(g) = ( ) g 2 e Ω · g 2 with the fading figure m = 1 in the Nakagami-m Γ(m) Ω 2 distribution[2]. Peppas presents a closed-form expres- 1 m m m−1 − m g = ( ) g e Ω sion for the moments generating function of the half- Γ(m) Ω harmonic mean of two independent, not necessarily With the shape parameter α and the scale param- identically distributed gamma random variables with eter β, the probability density function of the gamma arbitrary parameters[5]. distribution is as follows. With the shape parameter m and the spread pa- 1 − − g f(g) = gα 1e β rameter Ω, the probability density function of the Nak- Γ(α)βα E-ISSN: 2224-2902 67 Volume 13, 2016 WSEAS TRANSACTIONS on BIOLOGY and BIOMEDICINE Li-Fei Huang, Jen-Jen Lin 2.2 The maximum likelihood estimator Table 1: The moment estimator of m Let n be the sample size. The likelihood function is m Ω m^ Yn 1 1 1.003686 L(α; β) = f(gijα; β) 1 291848 1.003686 i=1 0.75 1 0.7531207 Yn 1 − − gi 0.75 291848 0.7531207 = gα 1e β Γ(α)βα i i=1 0.5 1 0.5006322 P n 0.5 291848 0.5006322 Yn gi 1 − − i=1 = f gnf g gα 1e β : 0.25 1 0.2493589 Γ(α)βα i 0.25 291848 0.2493589 i=1 The log likelihood function is ln L(α; β) = −n lnfΓ(α)βαg The moment generating function: Xn 1 Xn +(α − 1) ln g − g i β i M (t) = E(etg) i=1 i=1 G P − n @ ln L(α; β) nα i=1 gi Z 1 = + = 0 1 − − 1 − 2 α 1 ( β t)g @β β β = α g e dg P 0 Γ(α)β n g ) Ω^ = i=1 i ; Z 1 n 1 − − 1−βt α 1 ( β )g = α g e dg which is the same with its moment estimator. 0 Γ(α)β 0 Xn @ ln L(α; β) − · Γ (α) − 1 = n n ln β + ln gi = ( )α @α Γ(α) 1 − βt i=1 P 0 n Xn Γ (α) i=1 gi 0 − − −α−1 − j = −n · − n lnf g + ln gi = 0 E(G) = MG(0) = α(1 βt) ( β) t=0 = αβ Γ(α) nα i=1 0 P P E(G2) = M 00 (0) = αβ2(α + 1) Γ (α) n g n ln g G ) − + ln α = lnf i=1 i g − i=1 i Γ(α) n n − − − −α−2 − j = αβ( α 1)(1 βt) ( β) t=0 The digamma function is defined to be 0 V ar(G) = E(G2)−E(G)2 = αβ2(α+1−α) = αβ2 d Γ (α) (α) = ln Γ(α) = : dα Γ(α) E(G)2 V ar(G) α = ; β = V ar(G) E(G) Now we only need to deal with the digamma function. The likelihood function of the Nakagami It can be shown that distribution is too complicate to find the maximum likelihood estimator of m. Let 2 2 P P E(N ) n n m = : i=1 gi i=1 ln gi V ar(N 2) − (α) + ln α = 4 = lnf g − : n n Since the moment generation function of the Nak- Abramowitz and Stegun find that agami distribution does not exist, it’s necessary to ap- 1 1 1 ply the gamma distribution in the process of finding − (α) + ln α = 4 ≈ + − + ··· : 2α 12α2 120α4 the moment estimator of m. If a random sample of n1, n2,..., n1000 is se- One term is used to obtain the first order maximum lected spread parameter Ω, the moment estimators of likelihood estimator. shape parameters are listed in Table 1. The value of Ω 1 1 won’t affect the estimation of m. 4 = ) m^ 1 =α ^1 = 2^α1 24 E-ISSN: 2224-2902 68 Volume 13, 2016 WSEAS TRANSACTIONS on BIOLOGY and BIOMEDICINE Li-Fei Huang, Jen-Jen Lin Table 2: The 1st and 2nd order maximum likelihood estimator of m m Ω m^ 1 m^ 2 1 1 0.8687865 1.001858 1 291848 0.8687865 1.001858 0.75 1 0.628175 0.7499995 Table 3: The bias and the standard deviation of the 0.75 291848 0.628175 0.7499995 moment estimator of m 0.5 1 0.3945828 0.4998179 m Ω bias of m^ stdev. of m^ 0.5 291848 0.3945828 0.4998179 1 1 0 0.06 0.25 1 0.1763376 0.2499231 1 291848 0 0.06 0.25 291848 0.1763376 0.2499231 0.75 1 0 0.05 0.75 291848 0 0.05 0.5 1 0.002 0.04 Two terms are used to obtain the second order maxi- 0.5 291848 0.002 0.04 mum likelihood estimator. 0.25 1 0.003 0.025 0.25 291848 0.003 0.025 4 1 1 ) 2 ) = + 2 12∆^α2 = 6^α2 + 1 2^α2 12^α2 p 3 + 9 + 124 12∆^α2−6^α −1 = 0 ) m^ =α ^ = 2 2 2 2 124 If a random sample of n1, n2,..., n1000 is se- lected from a Nakagami distribution with the shape parameter m and the spread parameter Ω, the max- imum likelihood estimators of shape parameters are listed in Table 2. Where m^ 2 is adjusted by its bias. The value of Ω won’t affect the estimation of m. 3 The distribution of the Nakagami- m estimator Table 4: The bias and the standard deviation of the second order maximum likelihood estimator of m In order to compare the moment estimator and the maximum likelihood estimator, their distributions m Ω bias of m^ 2 stdev. of m^ 2 need to be found. The Kolmogorov-Smirnov test is 1 1 0.01 0.04 applied to find the distribution of the Nakagami-m es- 1 291848 0.01 0.04 timator. 0.75 1 0.015 0.03 0.75 291848 0.015 0.03 3.1 The moment estimator 0.5 1 0.021 0.02 The moment estimator is normally distributed with a 0.5 291848 0.021 0.02 smaller bias and a larger standard deviation. 0.25 1 0.031 0.0075 0.25 291848 0.031 0.0075 3.2 The maximum likelihood estimator The first order maximum likelihood estimator is not discussed because it’s too far away from the theoreti- cal value of m. The second order maximum likelihood estimator is normally distributed with a larger bias and a smaller standard deviation.
Details
-
File Typepdf
-
Upload Time-
-
Content LanguagesEnglish
-
Upload UserAnonymous/Not logged-in
-
File Pages7 Page
-
File Size-