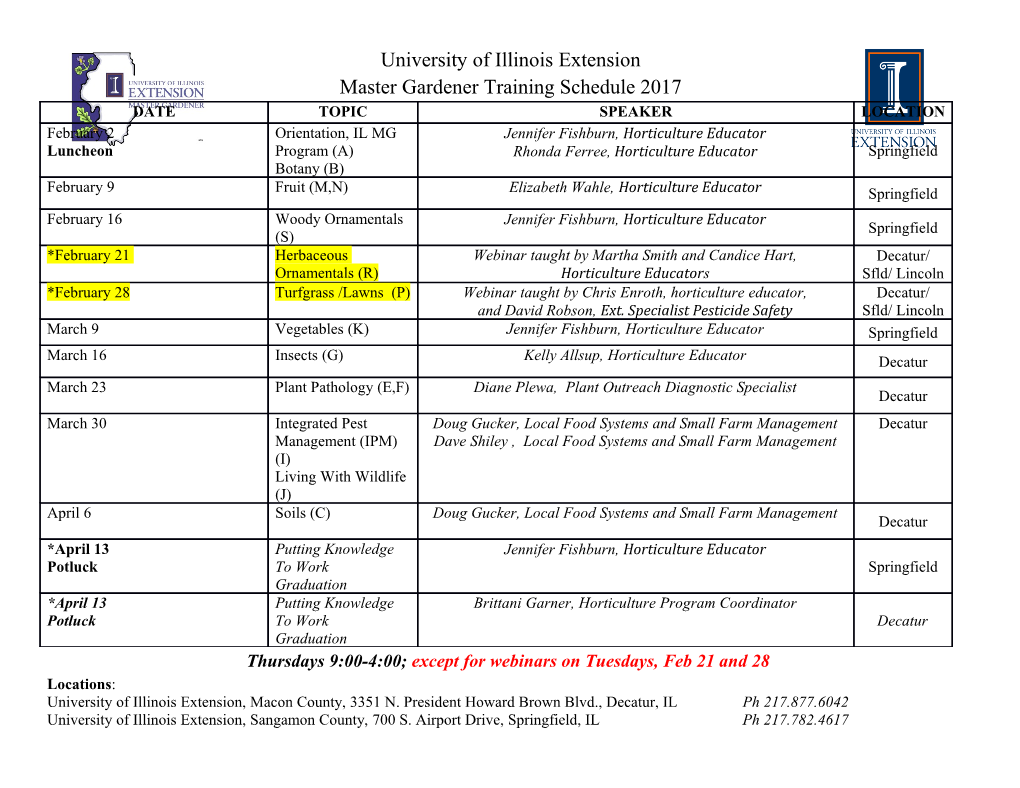
UvA-DARE (Digital Academic Repository) Normalized information distance Vitányi, P.M.B.; Balbach, F.J.; Cilibrasi, R.L.; Li, M. Publication date 2008 Link to publication Citation for published version (APA): Vitányi, P. M. B., Balbach, F. J., Cilibrasi, R. L., & Li, M. (2008). Normalized information distance. Institute for Logic, Language and Computation. http://arxiv.org/abs/0809.2553 General rights It is not permitted to download or to forward/distribute the text or part of it without the consent of the author(s) and/or copyright holder(s), other than for strictly personal, individual use, unless the work is under an open content license (like Creative Commons). Disclaimer/Complaints regulations If you believe that digital publication of certain material infringes any of your rights or (privacy) interests, please let the Library know, stating your reasons. In case of a legitimate complaint, the Library will make the material inaccessible and/or remove it from the website. Please Ask the Library: https://uba.uva.nl/en/contact, or a letter to: Library of the University of Amsterdam, Secretariat, Singel 425, 1012 WP Amsterdam, The Netherlands. You will be contacted as soon as possible. UvA-DARE is a service provided by the library of the University of Amsterdam (https://dare.uva.nl) Download date:26 Sep 2021 Chapter 3 Normalized Information Distance Paul M. B. Vita´nyi, Frank J. Balbach, Rudi L. Cilibrasi, and Ming Li Abstract The normali ed in!ormation distance is a uni"ersal distance measure !or ob#ects o! all kinds. $t is based on %olmogoro" com&le'ity and thus uncom&utable, but there are (ays to utili e it. First, com&ression algorithms can be used to a&&ro'imate the %olmogoro" com&le'ity i! the ob#ects ha"e a string re&resentation. )econd, !or names and abstract conce&ts, &age count statistics !rom the *orld *ide *eb can be used. These &ractical reali ations o! the normali ed in!ormation distance can then be a&&lied to machine learning tasks, e'&ecially clustering, to &er!orm !eature+!ree and &arameter+!ree data mining. This cha&ter discusses the theoretical !oundations o! the normali ed in!ormation distance and both &ractical reali ations. $t &resents numerous e'am&les o! success!ul real+(orld a&&lications based on these distance measures, ranging !rom bioin!ormatics to music clustering to machine translation. 3.1 Introduction The ty&ical data mining algorithm uses e'&licitly gi"en !eatures o! the data to assess their similarity and disco"er &atterns among them. $t also comes (ith many &arameters !or the user to tune to s&eci,c needs according to the domain at hand. $n this cha&ter, by contrast, (e are discussing algorithms that neither use !eatures o! the data nor &ro"ide any &arameters to be tuned, but that ne"ertheless o!ten out&er!orm algorithms o! the a!orementioned kind. $n addition, the methods &resented here are not #ust heuristics that ha&&en to (ork, but they are !ounded in the mathematical theory o! %olmogoro" com&le'ity. The &roblems discussed in this cha&ter (ill mostly, yet not e'clusi"ely, be clustering tasks, in (hich naturally the notion o! distance bet(een ob#ects &lays a dominant role. Paul M. B. Vita´nyi C*$, %ruislaan -./, .012 )J 3msterdam, The 4etherlands; e+mail6 paul"7c(i.nl Frank J. Balbach 8ni"ersity o! *aterloo, *aterloo, 94, Canada5 e+mail6 fbalbach7u(aterloo.ca :su&&orted by a &ostdoctoral !ello(shi& o! the ;erman 3cademic <'change )er"ice :=33=>> Rudi L. Cilibrasi C*$, %ruislaan -./, .012 )J 3msterdam, The 4etherlands; e+mail6 cilibrar7cilibrar.com Ming Li 8ni"ersity o! *aterloo, *aterloo, 94, Canada5 e+mail6 mli7u(aterloo.ca /1 -0 Paul M. B. Vita´nyi, Frank J. Balbach, Rudi L. Cilibrasi, and Ming Li There are good reasons to a"oid &arameter laden methods. )etting the &arameters re?uires an intimate understanding o! the underlying algorithm. )etting them incorrectly can result in missing the right &atterns or, &erha&s (orse, in detecting !alse ones. Moreo"er, com&aring t(o &arametri ed algorithms is di!,cult because di!!erent &arameter settings can gi"e a (rong im&ression that one algorithm is better than another, (hen in !act one is sim&ly ad#usted &oorly. Com&arisons using the o&timal &arameter settings !or each algorithm are o! little hel& because these settings are hardly e"er kno(n in real situations. Lastly, t(eaking &arameters might tem&t users to im&ose their assum&tions and e'&ectations on the algorithm. There are also good reasons to a"oid !eature based methods. =etermining the rele"ant !eatures re?uires domain kno(ledge, and determining ho( rele"ant they are o!ten re?uires guessing. $m&lementing the !ea+ ture e'traction in an algorithm can be di!,cult, error+&rone, and is o!ten time consuming. $t also limits the a&&licability o! an algorithm to a s&eci,c ,eld. @o( can an algorithm &er!orm (ell i! it does not e'tract the im&ortant !eatures o! the data and does not allo( us to t(eak its &arameters to hel& it do the right thingA 9! course, &arameter and !eature !ree algorithms cannot mind read, so i! (e a &riori kno( the !eatures, ho( to e'tract them, and ho( to combine them into e'actly the distance measure (e (ant, (e should do #ust that. For e'am&le, i! (e ha"e a list o! cars (ith their color, motor rating, etc. and (ant to cluster them by color, (e can easily do that in a straight!or(ard (ay. Parameter and !eature !ree algorithms are made (ith a di!!erent scenario in mind. $n this exploratory data mining scenario (e are con!ronted (ith data (hose im&ortant !eatures and ho( to e'tract them are unkno(n to us :&erha&s there are not e"en !eatures>. *e are then stri"ing not !or a certain similarity measure, but !or the similarity measure bet(een the ob#ects. =oes such an absolute measure o! similarity e'ist at allA Bes, it does, in theory. $t is called the in!ormation distance, and the idea behind it is that t(o ob#ects are similar i! there is a sim&le descri&tion o! ho( to trans!orm each one o! them into the other one. $!, ho(e"er, all such descri&tions are com&le', the ob#ects are deemed dissimilar. For e'am&le, an image and its negati"e are "ery similar because the trans!ormation can be described as Cin"ert e"ery &i'el.D By contrast, a descri&tion o! ho( to trans!orm a blank can"as into da VinciEs Mona Lisa (ould in"ol"e the com&lete, and com&arably large, descri&tion o! that &ainting. The latter e'am&le already &oints to some issues one has to take care o!, like asymmetry and normali a+ tion. 3symmetry re!ers to the !act that, a!ter all, the in"erse trans!ormation o! the Mona Lisa into a blank can"as can be described rather sim&ly. 4ormali ation re!ers to the !act that the trans!ormation descri&tion si e must be seen in relation to the si e o! the &artici&ating ob#ects. )ection /.F details ho( these and other issues are dealt (ith and e'&lains in (hich sense the resulting information distance measure is uni"ersal. The !ormulation o! this distance measure (ill in"ol"e the mathematical theory o! %olmogoro" com&le'ity, (hich is generally concerned (ith shortest e!!ecti"e descri&tions. While the de,nition o! the in!ormation distance is rather theoretical and cannot be reali ed in &ractice, one can still use its theoretical idea and a&&ro'imate it (ith &ractical methods. T(o such a&&roaches are discussed in subse?uent sections. They di!!er in (hich &ro&erty o! the %olmogoro" com&le'ity they use and to (hat kind o! ob#ects they a&&ly. The ,rst a&&roach, &resented in )ect. /./, e'&loits the relation bet(een %olmogoro" com&le'ity and data com&ression and conse?uently em&loys common com&ression algorithms to measure distances bet(een ob#ects. This method is a&&licable (hene"er the data to be clustered are gi"en in a com&ressible !orm, !or instance, as a te't or other literal descri&tion. The second a&&roach, &resented in )ect. /.-, e'&loits the relation bet(een %olmogoro" com&le'ity and &robability. $t uses statistics generated by common *eb search engines to measure distances bet(een ob+ #ects. This method is a&&licable to non+literal ob#ects, names and conce&ts, (hose &ro&erties and interrela+ tions are gi"en by common sense and human kno(ledge. / 4ormali ed $n!ormation =istance -. 3.2 Normalized Information Distance %olmogoro" com&le'ity measures the absolute in!ormation content o! indi"idual ob#ects. For the &ur&ose o! data mining, es&ecially clustering, (e (ould also like to be able to measure the absolute in!ormation distance bet(een indi"idual ob#ects. )uch a notion should be uni"ersal in the sense that it contains all other alternati"e or intuiti"e notions o! com&utable distances as s&ecial cases. )uch a notion should also ser"e as an absolute measure o! the in!ormational, or cogniti"e, distance bet(een discrete ob#ects x and y.
Details
-
File Typepdf
-
Upload Time-
-
Content LanguagesEnglish
-
Upload UserAnonymous/Not logged-in
-
File Pages34 Page
-
File Size-