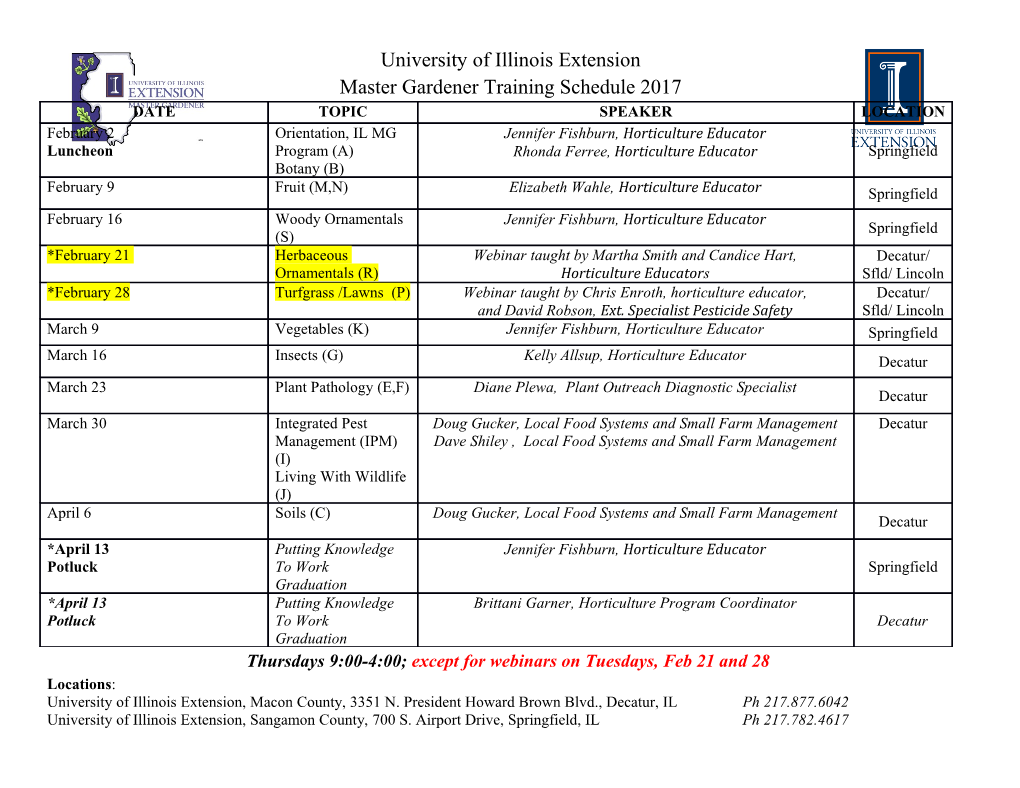
Machine learning tools for biomarker discovery Chloé-Agathe Azencott To cite this version: Chloé-Agathe Azencott. Machine learning tools for biomarker discovery. Machine Learning [stat.ML]. Sorbonne Université UPMC, 2020. tel-02354924v2 HAL Id: tel-02354924 https://hal.archives-ouvertes.fr/tel-02354924v2 Submitted on 24 Jan 2020 HAL is a multi-disciplinary open access L’archive ouverte pluridisciplinaire HAL, est archive for the deposit and dissemination of sci- destinée au dépôt et à la diffusion de documents entific research documents, whether they are pub- scientifiques de niveau recherche, publiés ou non, lished or not. The documents may come from émanant des établissements d’enseignement et de teaching and research institutions in France or recherche français ou étrangers, des laboratoires abroad, or from public or private research centers. publics ou privés. MACHINE LEARNING TOOLS FOR BIOMARKER DISCOVERY Chloé-Agathe Azencott Habilitation à Diriger des Recherches Déposée à l’UFR Ingénierie de Sorbonne Université Spécialité Informatique January 30, 2020 Membres du jury M. Mario Marchand (Université Laval, Canada) Rapporteur Mme Nataša Pržulj (University College London, UK, and Barcelona Supercomputing Center, Spain) Rapporteuse M. Bertrand Thirion (Inria Saclay, France) Rapporteur M. Michel Blum (Université Grenoble Alpes, France) Examinateur M. Grégory Nuel (Sorbonne Université, France) Examinateur Mme Marylyn Ritchie (University of Pennsylvania, USA) Examinatrice RÉSUMÉ Mes travaux de recherche s’inscrivent dans le cadre du développement de techniques d’apprentissage statistique (« machine learning ») pour la recherche thérapeutique. Ils visent en particulier à proposer des outils informatiques permettant d’exploiter des jeux de données pour en extraire des hypothèses biologiques expliquant au niveau génomique ou moléculaire les différences entre échantillons observées à un niveau macroscopique. De tels outils sont nécessaires à la mise en œuvre de la médecine de précision, qui requiert d’identifier les caractéristiques, génomiques ou autres, expliquant les différences de pronostic ou de réponse thérapeutique entre patients présentant les mêmes symptômes. Ces questions peuvent souvent être formulées comme des problèmes de sélection de variables. Les jeux de données utilisés, cependant, contiennent généralement largement plus de variables que d’échantillons, ce qui pose des difficultés statistiques. Pour répondre à ces défis, mes travaux s’orientent autour de trois axes. Premièrement, les connaissances accumulées sur les systèmes biologiques peuvent sou- vent être représentées sous la forme de réseaux biologiques. Sous l’hypothèse que les variables connectées par ces réseaux sont susceptibles d’agir conjointement sur un phé- notype, nous proposons d’utiliser ces réseaux pour guider un algorithme de sélection de variables. Il s’agit ici d’utiliser des contraintes qui encouragent les variables sélectionnées à être connectées sur un réseau donné. La formulation que nous avons proposée, qui s’inscrit dans le cadre plus large de ce que j’appelle la pertinence régularisée, permet de résoudre efficacement le problème de sélection de variables sur des jeux de données comportant des centaines de milliers de variables. Deuxièment, pour compenser le faible nombre d’échantillons disponibles, les méthodes dites multitâches résolvent simultanément plusieurs problèmes, ou tâches, proches. Nous avons étendu la pertinence régularisée à ce contexte. Je me suis aussi intéressée au cas où il est possible de définir une similarité entre tâches, afin d’imposer que les variables sélectionnées pour deux tâches soient d’autant plus similaires que les deux tâches sont semblables. Ces approches sont pertinentes dans le cas de l’étude de la réponse à différents traitements médicamenteux : on peut alors utiliser la similarité entre les structures moléculaires de ces médicaments, sujet que j’ai étudié pendant ma thèse. Enfin, la plupart des approches de sélection de variables utilisées dans le contexte de la génomique ne peuvent expliquer le phénomène d’intérêt que par des effets linéaires. Cependant, de nombreux travaux indiquent que les régions du génome peuvent interagir de façon non-linéaire. Modéliser de telles interactions, que l’on qualifie d’épistatiques, aggrave cependant les problèmes statistiques déjà rencontrés précédemment, et crée aussi des problèmes computationnels : il devient difficile d’évaluer toutes les combinaisons possibles de variables. Mes travaux portent aussi bien sur les difficultés calculatoires que sur les difficultés statistiques rencontrées dans la modélisation d’interactions quadratiques entre paires de régions du génomes. Plus récemment, nous avons aussi développé des approches permettant la modélisation d’interactions plus complexes grâce à des méthodes à noyaux. iii ACKNOWLEDGMENTS This document summarizes the results of fourteen years of work in machine learning applications to bioinformatics and chemoinformatics. The number of people that should be thanked is staggering, and I will therefore have to omit a large number of them. First and foremost, I am deeply indepted to my many collaborators, without whom none of the work nor ideas I present here would have happened. It is with great pleasure that I thank Fabian Aicheler, Tero Aittokallio, André Altmann, Nadine Andrieu, Pierre Baldi, Annalisa Barla, Víctor Bellón, Valentina Boeva, Karsten M. Borgwardt, Lawrence Cayton, Clément Chatelain, Jonathan H. Chen, Héctor Climente-González, Kenny Daily, Mark Daly, Ahmed Debit, Stefani Dritsa, Laramie Duncan, Diane Duroux, Fajwel Fogel, Samuele Fiorini, Stephen Friend, Xavier Gidrol, Udo Gieraths, Anna Goldenberg, Dominik Grimm, Anne-Sophie Hamy-Petit, Tony Kam-Thong, Nazanin Karbalai, Samuel Kaski, Yoshinobu Kawahara, Alexandre Ksikes, Matthew A. Kayala, Christophe Le Priol, Fabienne Lesueur, Ting-Wan Lin, Felipe Llinares López, Christine Lonjou, Daniel MacArthur, Thibaud Mar- tinez, Bertram Müller-Myhsok, Asma Nouira, Thea Norman, Charlotte Proudhon, Benno Pütz, Liva Ralaivola, Antonio Rausell, Antoine Recanati, Fabien Reyal, Daniel Rovera, Sushmita Roy, Kaitlin Samocha, Philipp G. Sämann, Bernard Schölkopf, Nino Shervashidze, Carl-Johann Simon-Gabriel, Lotfi Slim, Jordan Smoller, Gustavo Stolovitzky, Véronique Stoven, Mahito Sugiyama, S. Joshua Swamidass, Sheryl Tsai, Kristel Van Steen, Jean- Philippe Vert, Makoto Yamada, and Weiyi Zhang. My work was in part inspired by two DREAM challenges. I hence also wish to acknowledge all of the many people involved in the various stages of these challenges; all members of the NIEHS-NCATS-UNC DREAM Toxicogenetics Collaboration and The Rheumatoid Arthritis Challenge Consortium; and more particularly, Christopher Bare, Jing Cui, Federica Eduati, Andre O. Falcao, Mike Kellen, Lara M. Mangravite, Michael P. Menden, Gaurav Pandey, Dimitrios Pappas, Abhishek Pratap, Julio Saez-Rodriguez, Solveig K. Siebert, Eli Stahl, Christine Suver, and Yang Xie. Along the years, my work has been supported by Agence Nationale pour la Recherche, the Alexander von Humboldt Stiftung, the Deutsche Forschungsgemeinschaft, and the European Research Council, as well as by Sancare and Sanofi-Adventis. I am grateful for their funding. I am very happy to thank all current and past members of my current lab, the Centre for Bioinformatics of Mines ParisTech. I would particularly like to emphasize the role of Véronique Stoven, Jean-Philippe Vert, and Thomas Walter, for their warm welcome in their group, for their constant support, for all I have learned and am still learning from them, and for the friendly atmosphere they kindle at CBIO. With this HDR, I am expected to demonstrate my ability to conduct research independently, and to supervise trainees. I could not show the latter without the trust that my (co- supervised) trainees I have placed in me, and I therefore thank Víctor Bellón, my first PhD student; Christophe Le Priol, Héctor Climente-González, Lotfi Slim, and Asma Nouira, who followed suit; as well as Jean-Daniel Granet, Thibaud Martinez, Killian Poulaud, v Antoine Récanati, Athénaïs Vaginay and Weiyi Zhang. Among former CBIO members, Marine Le Morvan, Benoît Playe, Erwan Scornet, and Nelle Varoquaux deserve special thanks as well, for scientific and non-scientific conversations alike. I am also pleased to thank Emmanuel Barillot, who makes our inclusion in the U900 team of Institut Curie possible. The Machine Learning and Computational Biology lab led by Karsten Borgwardt and formerly in Tübingen is where I started becoming an independent researcher. Warm thanks go to all the team, particularly Karsten; but also Aasa Feragen, Dominik Grimm, Theofanis Karaletsos, Christoph Lippert, Barbara Rakitsch, Nino Shervashidze, Oliver Stegle, and Mahito Sugiyama, whom I am happy to count as friends and colleagues. Thank you also to Fabian Aicheler, Udo Gieraths, and Valeri Velkov, who were the first students whose research I supervised. I do not discuss here much of the work I conducted at UC Irvine for my PhD thesis – there are already two hundred pages or so that you can read on the topic. Still, I am thankful to Pierre Baldi for the environment he provided, and to Jon Chen, Kenny Daily, Matt Kayala, Ramzi Nasr, Vishal Patel, and Josh Swamidass, for both hard work and friendship. For the two years of sisterhood, fighting together to increase the visibility of women in machine learning and data science, I thank past and current members of the Paris WiMLDS team: Chiara Biscaro, Natalie Cerneka, Caroline Chavier, Fanny Riols,
Details
-
File Typepdf
-
Upload Time-
-
Content LanguagesEnglish
-
Upload UserAnonymous/Not logged-in
-
File Pages108 Page
-
File Size-