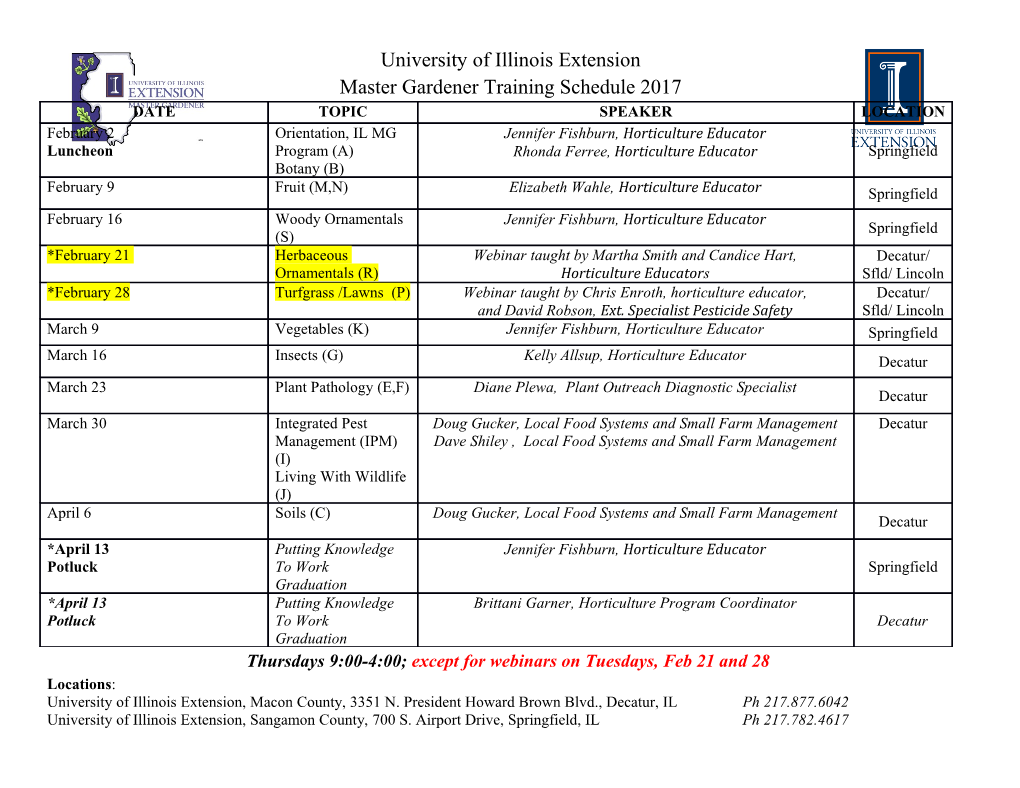
Probability, algorithmic complexity, and subjective randomness Thomas L. Griffiths Joshua B. Tenenbaum [email protected] [email protected] Department of Psychology Brain and Cognitive Sciences Department Stanford University Massachusetts Institute of Technology Abstract accounts that can express the strong prior knowl- We present a statistical account of human random- edge that contributes to our inferences. The struc- ness judgments that uses the idea of algorithmic tures that people find simple form a strict (and flex- complexity. We show that an existing measure of ible) subset of those easily expressed in a computer the randomness of a sequence corresponds to the as- sumption that non-random sequences are generated program. For example, the sequence of heads and by a particular probabilistic finite state automaton, tails TTHTTTHHTH appears quite complex to us, even and use this as the basis for an account that evalu- though, as the parity of the first 10 digits of π, it ates randomness in terms of the length of programs is easily generated by a computer. Identifying the for machines at different levels of the Chomsky hi- kinds of regularities that contribute to our sense of erarchy. This approach results in a model that pre- dicts human judgments better than the responses simplicity will be an important part of any cognitive of other participants in the same experiment. theory, and is in fact necessary since Kolmogorov complexity is not computable (Kolmogorov, 1965). The development of information theory prompted There is a crucial middle ground between Kol- cognitive scientists to formally examine how humans mogorov complexity and the arbitrary encoding encode experience, with a variety of schemes be- schemes to which Simon (1972) objected. We will ing used to predict subjective complexity (Leeuwen- explore this middle ground using an approach that berg, 1969), memorization difficulty (Restle, 1970), combines rational statistical inference with algorith- and sequence completion (Simon & Kotovsky, 1963). mic information theory. This approach gives an in- This proliferation of similar, seemingly arbitrary tuitive transparency to measures of complexity by theories was curtailed by Simon's (1972) observation expressing them in terms of probabilities, and uses that the inevitable high correlation between mea- computability to establish meaningful differences be- sures of information content renders them essentially tween them. We will test this approach on judg- equivalent. The development of algorithmic infor- ments of the randomness of binary sequences, since mation theory (see Li & Vitanyi, 1997, for a detailed randomness is one of the key applications of Kol- account) has revived some of these ideas, with code mogorov complexity: Kolmogorov (1965) suggested lengths playing a central role in recent accounts of that random sequences are irreducibly complex, a human concept learning (Feldman, 2000), subjective notion that has inspired several psychological theo- randomness (Falk & Konold, 1997), and the role of ries (eg. Falk & Konold, 1997). We will analyze sub- simplicity in cognition (Chater, 1999). Algorithmic jective randomness as an inference about the source information theory avoids the arbitrariness of ear- of a sequence X, comparing its probability of being lier approaches by using a single universal code: the generated by a random source, P (X random), with complexity of an object (called the Kolmogorov com- its probability of generation by a morej regular pro- plexity after Kolmogorov, 1965) is the length of the cess, P (X regular). Since probabilities map directly shortest computer program that can reproduce it. to code lengths,j P (X regular) uniquely identifies a Chater and Vitanyi (2003) argue that a preference measure of complexity.j This formulation allows us for simplicity can be seen throughout cognition, from to identify the properties of an existing complexity perception to language learning. Their argument is measure (Falk & Konold, 1997), and extend it to based upon the important constraints that simplicity capture more of the statistical structure detected provides for solving problems of induction, which are by people. While Kolmogorov complexity is ex- central to cognition. Kolmogorov complexity gives a pressed in terms of programs for a universal Turing formal means of addressing \asymptotic" questions machine, many of the regularities people detect are about induction, such as why anything is learnable computable by simpler devices. We will use Chom- at all, but the constraints it imposes are too weak sky's (1956) hierarchy of formal languages to orga- to support the rapid inferences that characterize hu- nize our analysis, testing a set of nested models that man cognition. In order to explain how human be- can be interpreted in terms of the length of programs ings learn so much from so little, we need to consider for automata at different levels of the hierarchy. Complexity and randomness Falk & Konold (1997) DP model The idea of using a code based upon the length of Finite state model computer programs was independently proposed by Solomonoff (1964), Kolmogorov (1965), and Chaitin (1969), although it has come to be associated with Kolmogorov. A sequence X has Kolmogorov com- plexity K(X) equal to the length of the shortest program p for a (prefix) universal Turing machine U that produces X and then halts, Subjective randomness K(X) = min l(p); (1) p:U(p)=X 2 4 6 8 10 12 14 16 18 20 where l(p) is the length of p in bits. Kolmogorov Number of alternations complexity can be used to define algorithmic proba- bility, with the probability of X being Figure 1: Mean randomness ratings from Falk and Konold (1987, Experiment 1), shown with the pre- K(X) l(p) R(X) = 2− = max 2− : (2) dictions of DP and the finite state model. p:U(p)=X with 10 alternations. The mean DP has a similar There is no requirement that R(X) sum to one over profile, achieving a maximum at 12 alternations and all sequences; many probability distributions that giving a correlation of r = 0:93. correspond to codes are unnormalized, assigning the missing probability to an undefined sequence. Subjective randomness as Kolmogorov complexity can be used to mathemat- a statistical inference ically define the randomness of sequences, identify- Psychologists have claimed that the way we think ing a sequence X as random if l(X) K(X) is small − about chance is inconsistent with probability the- (Kolmogorov, 1965). While not necessarily follow- ory (eg. Kahneman & Tversky, 1972). For ex- ing the form of this definition, psychologists have ample, people are willing to say that X1=HHTHT preserved its spirit in proposing that the perceived is more random than X2=HHHHH, while they are randomness of a sequence increases with its complex- equally likely to arise by chance: P (X1 random) = ity. Falk and Konold (1997) consider a particular 1 5 j P (X2 random) = ( 2 ) . However, many of the ap- measure of complexity they call the “difficulty pre- parentlyj irrational aspects of human judgments can dictor" (DP ), calculated by counting the number of be understood by considering the possibility that runs (sub-sequences containing only heads or tails), people are assessing a different kind of probability { and adding twice the number of alternating sub- instead of P (X random), we evaluate P (random X) sequences. For example, the sequence TTTHHHTHTH (Griffiths & Tenenbaum,j 2001). j is a run of tails, a run of heads, and an alternating The statistical basis of subjective randomness be- sub-sequence, DP = 4. If there are several parti- comes clear if we view randomness judgments in tions into runs and alternations, DP is calculated terms of a signal detection task (cf. Lopes, 1982; 1 on the partition that results in the lowest score. Lopes & Oden, 1987). On seeing a stimulus X, Falk and Konold (1997) showed that DP corre- we consider two hypotheses: X was produced by lates remarkably well with subjective randomness a random process, or X was produced by a regular judgments. Figure 1 shows the results of Falk and process. Finding regularities is an important part Konold (1997, Experiment 1), in which 97 partici- of identifying predictable processes, a fundamental pants each rated the apparent randomness of ten bi- component of induction (Lopes, 1982). The deci- nary sequences of length 21, with each sequence con- sion about the source of X can be formalized as a taining between 2 and 20 alternations (transitions Bayesian inference, from heads to tails or vice versa). The mean rat- ings show the classic preference for overalternating P (random X) P (X random) P (random) j = j ; (3) sequences: the sequences perceived as most random P (regular X) P (X regular) P (regular) are those with 14 alternations, while a truly random j j process would be most likely to produce sequences in which the posterior odds in favor of a random gen- erating process are obtained from the likelihood ratio 1We modify DP slightly from the definition of Falk and the prior odds. The only part of the right hand and Konold (1997), who seem to require alternating sub- side of the equation affected by X is the likelihood sequences to be of even length. The equivalence results shown below also hold for their original version, but it ratio, which led Griffiths and Tenenbaum (2001) to makes the counter-intuitive interpretation of HTHTH as define the subjective randomness of X as a run of a single head, followed by an alternating sub- sequence, DP = 3. Under our formulation it would be P (X random) random(X) = log j ; (4) parsed as an alternating sequence, DP = 2. P (X regular) j being the evidence that X provides towards the con- 1 for zi = 1; 3; 5 and 0 for zi = 2; 4; 6 we have a clusion that it was produced by a random process.
Details
-
File Typepdf
-
Upload Time-
-
Content LanguagesEnglish
-
Upload UserAnonymous/Not logged-in
-
File Pages6 Page
-
File Size-