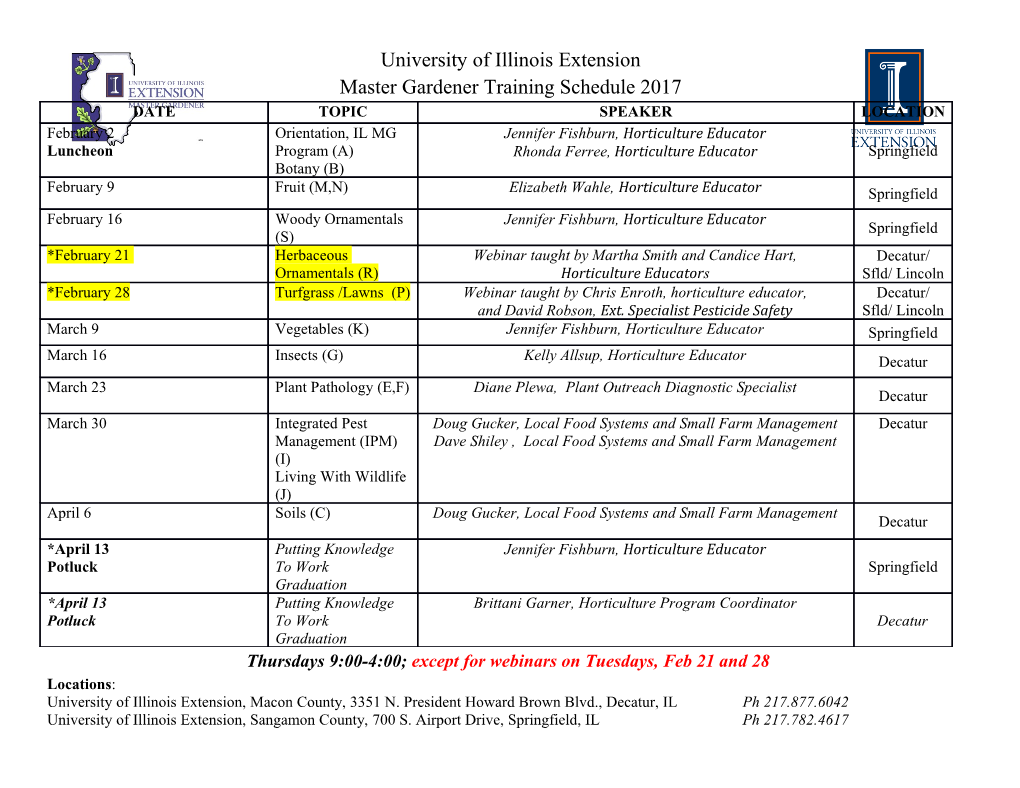
Optimal mass transport as a distance measure between images Axel Ringh1 1Department of Mathematics, KTH Royal Institute of Technology, Stockholm, Sweden. 21st of June 2018 INRIA, Sophia-Antipolis, France Acknowledgements This is based on joint work with Johan Karlsson1. [1] J. Karlsson, and A. Ringh. Generalized Sinkhorn iterations for regularizing inverse problems using optimal mass transport. SIAM Journal on Imaging Sciences, 10(4), 1935-1962, 2017. I acknowledge financial support from Swedish Research Council (VR) Swedish Foundation for Strategic Research (SSF) Code: https://github.com/aringh/Generalized-Sinkhorn-and-tomography The code is based on ODL: https://github.com/odlgroup/odl 1Department of Mathematics, KTH Royal Institute of Technology, Stockholm, Sweden 2 / 24 Outline Background Inverse problems Optimal mass transport Sinkhorn iterations - solving discretized optimal transport problems Sinkhorn iterations as dual coordinate ascent Inverse problems with optimal mass transport priors Example in computerized tomography 3 / 24 Problems of interest are ill-posed inverse problems: a solution might not exist, the solution might not be unique, the solution does not depend continuously on data. Simply put: A−1 does not exist as a continuous bijection! Comes down to: find approximate inverse Ay so that g = A(f ) + 'noise' =) Ay(g) ≈ f : Background Inverse problems Consider the problem of recovering f 2 X from data g 2 Y , given by g = A(f ) + 'noise' Notation: X is called the reconstruction space. Y is called the data space. A : X ! Y is the forward operator. A∗ : Y ! X denotes the adjoint operator 4 / 24 Background Inverse problems Consider the problem of recovering f 2 X from data g 2 Y , given by g = A(f ) + 'noise' Notation: X is called the reconstruction space. Y is called the data space. A : X ! Y is the forward operator. A∗ : Y ! X denotes the adjoint operator Problems of interest are ill-posed inverse problems: a solution might not exist, the solution might not be unique, the solution does not depend continuously on data. Simply put: A−1 does not exist as a continuous bijection! Comes down to: find approximate inverse Ay so that g = A(f ) + 'noise' =) Ay(g) ≈ f : 4 / 24 Background Variational regularization A common technique to solve ill-posed inverse problems is to use variational regularization: arg min G(A(f ); g) + λF(f ) f 2X G : Y × Y ! R, data discrepancy functional. F : X ! R, regularization functional. λ is the regularization parameter. Controls trade-off between data matching and regularization. Common example in imaging is total variation regularization: 2 G(h; g) = kh − gk2, F(f ) = krf k1. If A is linear this is a convex problem! 5 / 24 One way: consider arg min G(A(f ); g) + λF(f ) + γH(f~; f ) f 2X f~ is prior/template H defines \closeness" to f~. What is a good choice for H? Scenarios where potentially of interest. incomplete measurements, e.g. limited angle tomography. spatiotemporal imaging: T data is a time-series of data sets: fgt gt=0. For each set, the underlying image has undergone a deformation. y each data set gt normally \contains less information": A (gt ) is a poor reconstruction. Approach: solve coupled inverse problems T T X h i X arg min G(A(fj ); gj ) + λF(fj ) + γH(fj−1; fj ) f ;:::;f 2X 0 T j=0 j=1 Background Incorporating prior information in variational schemes How can one incorporate prior information in such a scheme? 6 / 24 Scenarios where potentially of interest. incomplete measurements, e.g. limited angle tomography. spatiotemporal imaging: T data is a time-series of data sets: fgt gt=0. For each set, the underlying image has undergone a deformation. y each data set gt normally \contains less information": A (gt ) is a poor reconstruction. Approach: solve coupled inverse problems T T X h i X arg min G(A(fj ); gj ) + λF(fj ) + γH(fj−1; fj ) f ;:::;f 2X 0 T j=0 j=1 Background Incorporating prior information in variational schemes How can one incorporate prior information in such a scheme? One way: consider arg min G(A(f ); g) + λF(f ) + γH(f~; f ) f 2X f~ is prior/template H defines \closeness" to f~. What is a good choice for H? 6 / 24 Background Incorporating prior information in variational schemes How can one incorporate prior information in such a scheme? One way: consider arg min G(A(f ); g) + λF(f ) + γH(f~; f ) f 2X f~ is prior/template H defines \closeness" to f~. What is a good choice for H? Scenarios where potentially of interest. incomplete measurements, e.g. limited angle tomography. spatiotemporal imaging: T data is a time-series of data sets: fgt gt=0. For each set, the underlying image has undergone a deformation. y each data set gt normally \contains less information": A (gt ) is a poor reconstruction. Approach: solve coupled inverse problems T T X h i X arg min G(A(fj ); gj ) + λF(fj ) + γH(fj−1; fj ) f ;:::;f 2X 0 T j=0 j=1 6 / 24 One suggestion: measure it pointwise, e.g., using an Lp metric Z 1=p p kf0 − f1kp = jf0(x) − f1(x)j dx : D Draw-backs: for example unsensitive to shifts. Example: It gives the same distance from f0 to f1 and f2: kf0 − f1k1 = kf0 − f2k1 = 8. Background Measuring distances between functions: the Lp metrics Given two functions f0(x) and f1(x), what is a suitable way to measure the distance between the two? 7 / 24 Draw-backs: for example unsensitive to shifts. Example: It gives the same distance from f0 to f1 and f2: kf0 − f1k1 = kf0 − f2k1 = 8. Background Measuring distances between functions: the Lp metrics Given two functions f0(x) and f1(x), what is a suitable way to measure the distance between the two? One suggestion: measure it pointwise, e.g., using an Lp metric Z 1=p p kf0 − f1kp = jf0(x) − f1(x)j dx : D 7 / 24 Background Measuring distances between functions: the Lp metrics Given two functions f0(x) and f1(x), what is a suitable way to measure the distance between the two? One suggestion: measure it pointwise, e.g., using an Lp metric Z 1=p p kf0 − f1kp = jf0(x) − f1(x)j dx : D Draw-backs: for example unsensitive to shifts. Example: It gives the same distance from f0 to f1 and f2: kf0 − f1k1 = kf0 − f2k1 = 8. 7 / 24 where φ is mass preserving map from f0 to f1: Z Z f1(x)dx = f0(x)dx for all A ⊂ X : x2A φ(x)2A Let c(x0; x1): X × X ! R+ describes the cost for transporting a unit mass from location x0 to x1. 3 3 2.5 2.5 Given two functions f0; f1 : X ! +, find the function φ : X ! X R 2 2 minimizing the transport cost Nonconvex problem! 1.5 1.5 Z 1 1 c(x; φ(x))f (x)dx 0 0.5 0.5 X 0 0 0 5 10 0 0.5 1 1.5 2 2.5 3 10 8 6 4 2 0 0 0.5 1 1.5 2 2.5 3 Background Optimal mass transport - Monge formulation Gaspard Monge: formulated optimal mass transport 1781. Optimal transport of soil for construction of forts and roads. Gaspard Monge 8 / 24 where φ is mass preserving map from f0 to f1: Z Z f1(x)dx = f0(x)dx for all A ⊂ X : x2A φ(x)2A Let c(x0; x1): X × X ! R+ describes the cost for transporting a unit mass from location x0 to x1. 3 3 2.5 2.5 Given two functions f0; f1 : X ! +, find the function φ : X ! X R 2 2 minimizing the transport cost Nonconvex problem! 1.5 1.5 Z 1 1 c(x; φ(x))f (x)dx 0 0.5 0.5 X 0 0 0 5 10 0 0.5 1 1.5 2 2.5 3 10 8 6 4 2 0 0 0.5 1 1.5 2 2.5 3 Background Optimal mass transport - Monge formulation Gaspard Monge: formulated optimal mass transport 1781. Optimal transport of soil for construction of forts and roads. Gaspard Monge 8 / 24 where φ is mass preserving map from f0 to f1: Z Z f1(x)dx = f0(x)dx for all A ⊂ X : x2A φ(x)2A Let c(x0; x1): X × X ! R+ describes the cost for transporting a unit mass from location x0 to x1. 3 3 2.5 2.5 Given two functions f0; f1 : X ! +, find the function φ : X ! X R 2 2 minimizing the transport cost Nonconvex problem! 1.5 1.5 Z 1 1 c(x; φ(x))f (x)dx 0 0.5 0.5 X 0 0 0 5 10 0 0.5 1 1.5 2 2.5 3 10 8 6 4 2 0 0 0.5 1 1.5 2 2.5 3 Background Optimal mass transport - Monge formulation Gaspard Monge: formulated optimal mass transport 1781. Optimal transport of soil for construction of forts and roads. Gaspard Monge 8 / 24 3 3 2.5 2.5 2 2 Nonconvex problem! 1.5 1.5 1 1 0.5 0.5 0 0 0 5 10 0 0.5 1 1.5 2 2.5 3 10 where φ is mass preserving map from f0 to f1: 8 6 Z Z 4 2 f (x)dx = f (x)dx for all A ⊂ X : 0 1 0 0 0.5 1 1.5 2 2.5 3 x2A φ(x)2A Background Optimal mass transport - Monge formulation Gaspard Monge: formulated optimal mass transport 1781.
Details
-
File Typepdf
-
Upload Time-
-
Content LanguagesEnglish
-
Upload UserAnonymous/Not logged-in
-
File Pages63 Page
-
File Size-