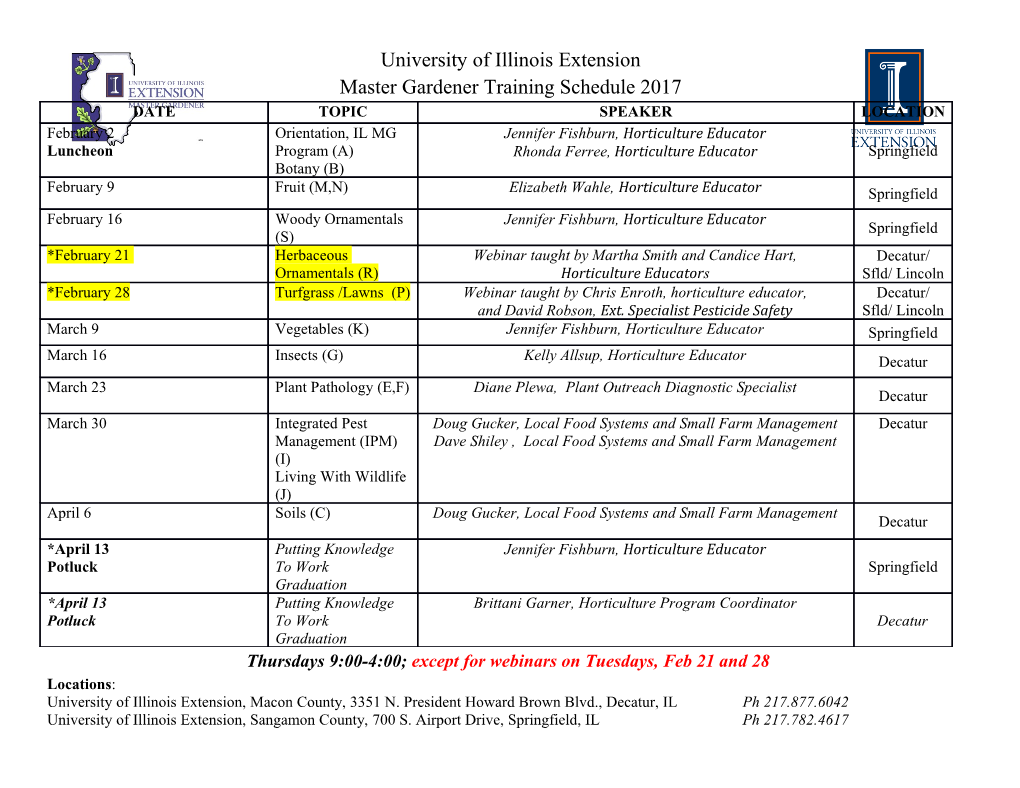
The value of pre-launch Twitter volumes in predicting initials sales of new cars MSC Business Studies Marketing Thesis by Jorrit Stein 10618015 First supervisor: Ms. E.Korkmaz Second supervisor: Dr. Umut Konus Final version January 30, 2015 1 Statement of originality This document is written by Student Jorrit Stein who declares to take full responsibility for the contents of this document. I declare that the text and the work presented in this document is original and that no sources other than those mentioned in the text and its references have been used in creating it. The Faculty of Economics and Business is responsible solely for the supervision of completion of the work, not for the contents. 2 Index Abstract ................................................................................................................................................... 4 Introduction ............................................................................................................................................. 5 Literature review ................................................................................................................................... 10 Predicting car sales ............................................................................................................................ 10 Advertisement ................................................................................................................................... 13 Web data based forecasting .............................................................................................................. 14 Twitter ............................................................................................................................................... 18 Forecasting with Twitter data ........................................................................................................... 19 Conceptual framework and hypotheses ............................................................................................... 21 Car model tweets .............................................................................................................................. 24 Brand tweets about car ..................................................................................................................... 26 Mass media ....................................................................................................................................... 27 Twitter data. ...................................................................................................................................... 30 Car sales data .................................................................................................................................... 30 Sample ............................................................................................................................................... 31 Variables ............................................................................................................................................ 33 Results ................................................................................................................................................... 39 Graphical description ........................................................................................................................ 40 Hypothesis testing ............................................................................................................................. 45 Discussion and conclusions ................................................................................................................... 52 Managerial implications ........................................................................................................................ 56 Further research .................................................................................................................................... 58 References ............................................................................................................................................. 59 Appendix 1: Search queries Twitter ...................................................................................................... 63 Appendix 2: Correlation plots ............................................................................................................... 65 Appendix 3: Key findings literature review ........................................................................................... 67 3 Abstract The recent technological developments, the rise of big data and the increased use of the internet and social media has led to a new era in the prediction of consumer behavior. Organizations and scholars increasingly see the value of collecting consumer information and using this data to make predictions about future consumer behavior. This study will look at the possibility to make predictions with online word of mouth on Twitter. Specifically, this research will try to find a relation between pre- launch Twitter volumes and the initial sales of new car models. The two Twitter volumes used in this research are the consumer and brand tweets about a new car model. Furthermore, we examine the relationship between brand media expenses and both pre-launch WOM on Twitter and post-launch initial car sales. Data was collected from seventeen new car models introduced in the Netherlands in 2013 en 2014. A descriptive, correlation and regression analysis were applied to test the relationships. The results of our research show no strong support for the ability to predict initials car sales of new car with pre-launch Twitter volumes generated by either consumers or brands. Although our outcomes do indicate that pre-launch WOM on Twitter seems to have a positive influence on the initial sales of new cars. Furthermore, we did not find a relationship between pre-launch brand expenses and initial sales. We did find a strong positive relationship between post-market brand investment in mass media promotion of a new car and the initial new car sales. The thesis results indicate that post-launch media expenses by a particular brand is a better predictor of initials car sales than the pre-launch consumer and brand tweet volumes and the pre-launch media expenses. 4 Introduction Nowadays, companies are collecting more and more consumer data. This development is caused by the widespread digitalization of world. The storage of big data combined with the technological development, new processing techniques and the increased usage of the internet and social network sites have led to a new era in the prediction of consumer behavior (Goldman Sachs Group, 2014). This digital development provides new opportunities, which has attracted a lot of attention from scholars during the last decades, but there is still plenty of room for new research. Business management teams increasingly see the value of collecting consumer information and using this data to make predictions about future consumer behavior. Consumer data is increasingly seen as a resource that can lead to a sustainable competitive advantage (The Economist Intelligence Unit, 2013). Consumer data is becoming such a resource in the business environment, as companies are collecting an increasing amount of divers, exclusive and unique consumer information, which is used to improve business practices. Consumer data, and in particular the results of its analysis, is a valuable resource for making strategic decision on business level which can results in the prolonged existence of a company (Barney, 1991). Recently, scholars are increasingly interested in research topics concerning predictive consumer analytics, due to the potential value of consumer data. As mentioned before the success of social media is an important pillar for the rise of consumer data. The various social media platforms offer consumers the opportunity to generate and spread information to a large audience in an unprecedented manner. This online generated worth of mouth (WOM) appears to be a promising source of information for the prediction of consumer behavior as WOM has already proven to be very valuable in the offline world. Recent studies on the diffusion of innovations have found that the volume of WOM correlates significantly with consumer activity and market outcome (Anderson, 2003; Neelamegham and Chintagunta, 1999). WOM constitutes the basis of interpersonal 5 communications that has an important influence on product evaluations and purchase decisions by consumers. According to Grewal, Cline and Davies (2003) WOM is more powerful than business communication, because WOM is considered more credible and valuable by consumers. The power WOM is also frightening for marketers, as informal discussions among consumers are difficult to control and can either make a product popular or unsuccessful in the market. As mentioned earlier, recent studies on the diffusion of innovations have found significant correlations between the volume of WOM and related market results. This research on the diffusion of innovation is based on the new product diffusion theory developed by Bass (2004) and Rogers (2004). The literature of Bass (2004) suggest that innovators in the early stage of a product life cycle are mainly affected by mass media. And after using the new products, the innovators pass their opinions to latecomers via the WOM channels. Rogers (2004) recognizes WOM as a channel of communication in the product life cycle, particularly
Details
-
File Typepdf
-
Upload Time-
-
Content LanguagesEnglish
-
Upload UserAnonymous/Not logged-in
-
File Pages68 Page
-
File Size-