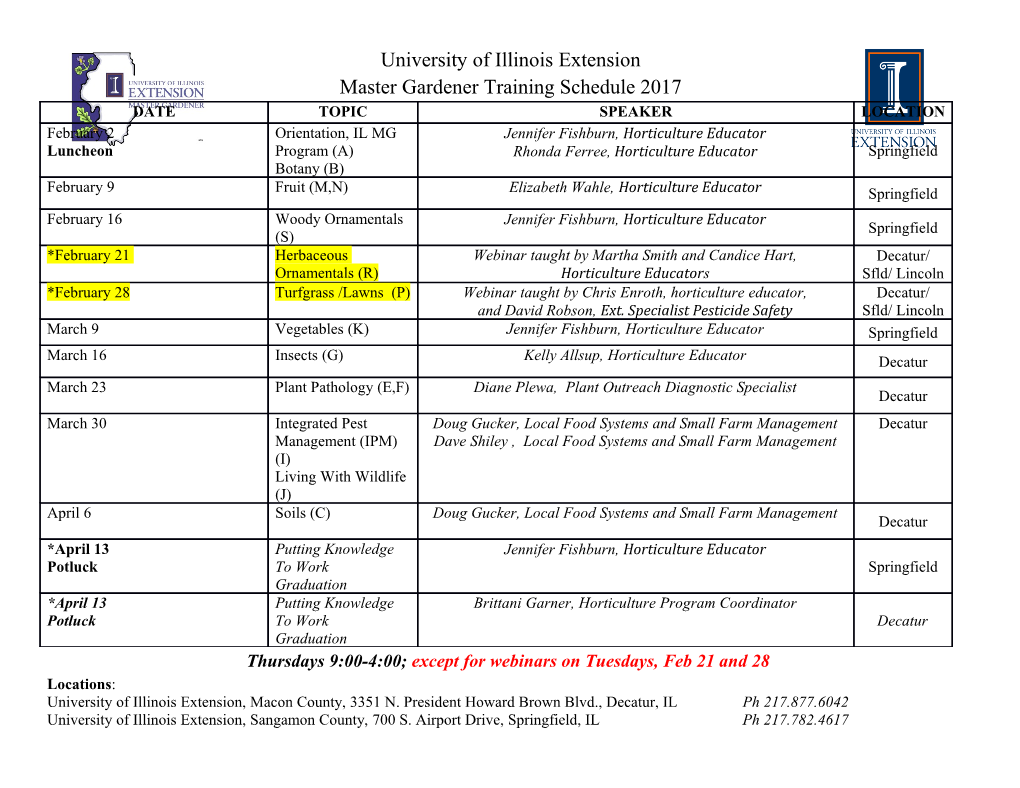
A High-quality Digital Library Supporting Computing Education: The Ensemble Approach Yinlin Chen Dissertation submitted to the faculty of the Virginia Polytechnic Institute and State University in partial fulfillment of the requirements for the degree of Doctor of Philosophy in Computer Science & Application Edward A. Fox, Chair Sanmay Das Weiguo Fan Christopher L. North Ricardo Da Silva Torres July 27, 2017 Blacksburg, Virginia 24061 Keywords: Educational Digital Library; ACM Classification System; Amazon Mechanical Turk; Classification; Transfer learning; Active learning; YouTube; SlideShare; Digital Library Service Quality; Cloud Computing Copyright 2017 Yinlin Chen A High-quality Digital Library Supporting Computing Education: The Ensemble Approach Yinlin Chen ABSTRACT Educational Digital Libraries (DLs) are complex information systems which are designed to support individuals' information needs and information seeking behavior. To have a broad impact on the communities in education and to serve for a long period, DLs need to structure and organize the resources in a way that facilitates the dissemination and the reuse of resources. Such a digital library should meet defined quality dimensions in the 5S (Societies, Scenarios, Spaces, Structures, Streams) framework - including completeness, consistency, efficiency, extensibility, and reliability - to ensure that a good quality DL is built. In this research, we addressed both external and internal quality aspects of DLs. For internal qualities, we focused on completeness and consistency of the collection, catalog, and repos- itory. We developed an application pipeline to acquire user-generated computing-related resources from YouTube and SlideShare for an educational DL. We applied machine learn- ing techniques to transfer what we learned from the ACM Digital Library dataset. We built classifiers to catalog resources according to the ACM Computing Classification Sys- tem from the two new domains that were evaluated using Amazon Mechanical Turk. For external qualities, we focused on efficiency, scalability, and reliability in DL services. We proposed cloud-based designs and applications to ensure and improve these qualities in DL services using cloud computing. The experimental results show that our proposed methods are promising for enhancing and enriching an educational digital library. This work received support from ACM, as well as the National Science Foundation under Grant Numbers DUE-0836940, DUE-0937863, and DUE-0840719, and IMLS LG-71-16-0037- 16. A High-quality Digital Library Supporting Computing Education: The Ensemble Approach Yinlin Chen GENERAL AUDIENCE ABSTRACT Educational Digital Libraries (DLs) are designed to serve users finding educational materials. To have a broad impact on the communities in education for a long period, DLs need to structure and organize the resources in a way that facilitates their dissemination and reuse. Such a digital library should be built on a well-defined framework to ensure that the services it provides are of good quality. In this research, we focused on the quality aspects of DLs. We developed an application pipeline to acquire resources contributed by the users from YouTube and SlideShare for an educational DL. We applied machine learning techniques to build classifiers in order to cata- log DL collections using a uniform classification system: the ACM Computing Classification System. We also used Amazon Mechanical Turk to evaluate the classifier’s prediction result and used the outcome to improve classifier performance. To ensure efficiency, scalability, and reliability in DL services, we proposed cloud-based designs and applications to enhance DL services. The experimental results show that our proposed methods are promising for enhancing and enriching an educational digital library. This work received support from ACM, as well as the National Science Foundation under Grant Numbers DUE-0836940, DUE-0937863, and DUE-0840719, and IMLS LG-71-16-0037- 16. Acknowledgments It has been a long journey; I feel so grateful that I have had the opportunity to work with my wonderful advisor, Dr. Edward A. Fox. Without him, I would never have been able to finish my Ph.D. study. I am so thankful for his guidance, support, and inspiration during these years. He is my mentor and role model; he taught me many things, not only in research, but also about life experiences. I cannot thank him enough. I would like to thank my committee members: Dr. Weiguo Fan, Dr. Ricardo Da Silva Torres, Dr. Christopher North, and Dr. Sanmay Das for their assistance and valuable feedback. I would like to thank Dr. Lillian (\Boots") Cassel for her support through the Ensemble project, and all the professors and students I have worked with during these years. It was a remarkable research experience in my life. I would like to thank the Association for Computing Machinery (ACM) for sharing the metadata from the ACM Digital Library for this research. This research work received support from the National Science Foundation (NSF) and the Institute of Museum and Library Services (IMLS) under Grant Numbers DUE-0836940, DUE-0937863, DUE-0840719, and IMLS LG-71-16-0037-16. I would like to thank Uma Murthy, Monika Akbar, and Seungwon Yang who worked with me on research projects during these years. I also thank Susan Marion for her assistance, and my colleagues at the Digital Library Research Laboratory, for their feedback and friendship throughout my study. iv I would like to thank my parents for their endless support and encouragement throughout my life. I am thankful for my two lovely children, Nathan and Nina, who always bring pleasure to me and give me strength. Finally, I would like especially to thank my wife, Chungwen Hsu, for her sacrifice, patience, and understanding during all these years. v Table of Contents Chapter 1 Introduction 1 1.1 Research motivation . 2 1.2 Research goals . 3 1.3 Research questions and hypotheses . 4 1.3.1 Research questions . 4 1.3.2 Hypotheses . 4 1.4 Research approach . 5 1.5 Dissertation organization . 7 Chapter 2 Background and Related work 8 2.1 Background . 8 2.1.1 Digital Libraries . 8 2.1.2 5S framework . 11 2.1.3 Dimensions of quality . 11 2.1.4 DL interoperability . 12 2.1.5 Dublin Core metadata terms . 13 2.1.6 OAI-PMH . 14 2.1.7 Ensemble: the computing portal . 15 2.1.8 Web 2.0 . 17 2.2 Related work . 18 2.2.1 Quality in the digital library . 18 2.2.2 Classification techniques . 20 vi 2.2.3 Feature selection . 21 2.2.4 Topic modeling . 22 2.2.5 Transfer learning . 23 2.2.6 Active learning . 24 Chapter 3 Building ACM digital library classifiers 27 3.1 Introduction . 27 3.1.1 The 2012 ACM Computing Classification System (CCS) . 28 3.1.2 ACM DL metadata dataset (ACM dataset) . 29 3.2 Research Method . 36 3.2.1 Data preparation . 37 3.2.2 Data cleaning . 40 3.2.3 Imbalanced data . 40 3.2.4 Feature extraction . 41 3.2.5 Feature selection . 42 3.2.6 Classifiers . 42 3.2.7 Evaluation metrics . 42 3.3 Experimental setup . 43 3.4 Experimental results . 46 3.5 Summary . 49 Chapter 4 Collection building for educational resources 52 4.1 Introduction . 53 4.1.1 Ensemble architecture . 53 4.1.2 Educational resources in Web 2.0 . 55 4.2 Research Method . 57 4.2.1 Query construction . 58 4.2.2 Topic modeling . 59 4.2.3 Crawlers . 60 vii 4.2.4 Document similarity . 62 4.3 Experimental setup . 63 4.4 Experimental design . 63 4.4.1 ACM datasets . 63 4.4.2 Experiment workflow . 64 4.5 Experimental results . 66 4.5.1 YouTube experiment result . 66 4.5.2 SlideShare experiment result . 69 4.5.3 Discussion . 70 4.6 Summary . 71 Chapter 5 Transfer the learning to Web 2.0 classifiers 73 5.1 Introduction . 73 5.2 Related work . 75 5.2.1 Definition of the transfer learning . 75 5.2.2 Domain adaptation . 76 5.2.3 Amazon Mechanical Turk (MTurk) . 76 5.3 Research method overview . 77 5.4 MTurk . 78 5.4.1 Dataset . 79 5.4.2 Workflow . 79 5.4.3 MTurk HIT template design . 80 5.4.4 MTurk experimental setup . 82 5.4.5 MTurk experiment result . 83 5.5 Domain adaptation classifier . 88 5.5.1 Research method . 88 5.5.2 Experimental setup . 90 5.5.3 Experimental design . 90 5.5.4 Experimental results . 92 viii 5.6 Summary . 92 Chapter 6 Ensure the QoS in a digital library using cloud computing 94 6.1 Introduction . 94 6.2 Related work . 95 6.2.1 Definition . 96 6.2.2 Cloud computing services . 97 6.3 Design approach . 100 6.4 Evaluation metrics . 102 6.5 Architecture design for efficiency . 102 6.5.1 Instance-based design . ..
Details
-
File Typepdf
-
Upload Time-
-
Content LanguagesEnglish
-
Upload UserAnonymous/Not logged-in
-
File Pages155 Page
-
File Size-