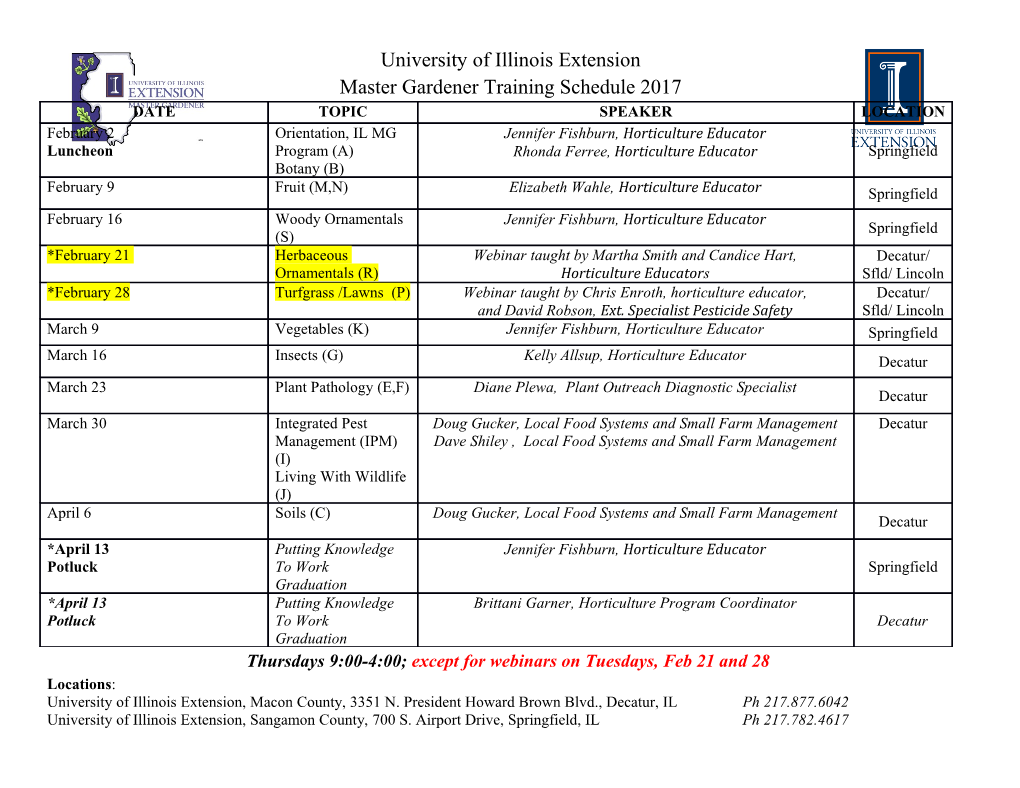
Future Technologies Conference (FTC) 2017 29-30 November 2017j Vancouver, Canada Super Generalized Central Limit Theorem: Limit Distributions for Sums of Non-Identical Random Variables with Power-Laws Masaru Shintani and Ken Umeno Department of Applied Mathematics and Physics, Graduate School of Informatics, Kyoto University Yoshida-honmachi, Sakyo-ku, Kyoto 606–8501, Japan Email: [email protected], [email protected] Abstract—In nature or societies, the power-law is present ubiq- its characteristic function φ(t) as: uitously, and then it is important to investigate the characteristics 1 Z 1 of power-laws in the recent era of big data. In this paper we S(x; α; β; γ; µ) = φ(t)e−ixtdx; prove the superposition of non-identical stochastic processes with 2π −∞ power-laws converges in density to a unique stable distribution. This property can be used to explain the universality of stable laws such that the sums of the logarithmic return of non-identical where φ(t; α; β; γ; µ) is expressed as: stock price fluctuations follow stable distributions. φ(t) = exp fiµt − γαjtjα(1 − iβsgn(t)w(α; t))g Keywords—Power-law; big data; limit distribution tan (πα=2) if α 6= 1 w(α; t) = − 2/π log jtj if α = 1: I. INTRODUCTION The parameters α; β; γ and µ are real constants satisfying There are a lot of data that follow the power-laws in 0 < α ≤ 2, −1 ≤ β ≤ 1, γ > 0, and denote the indices the world. Examples of recent studies include, but are not for power-law in stable distributions, the skewness, the scale limited to the financial market [1]–[7], the distribution of parameter and the location, respectively. When α = 2 and β = people’s assets [8], the distribution of waiting times between 0, the probability density function obeys a normal distribution. earthquakes occurring [9] and the dependence of the number Note that explicit forms of stable distributions are not known of wars on its intensity [10]. It is then important to investigate for general parameters α and β except for a few cases such as the general characteristics of power-laws. the Cauchy distribution (α = 1; β = 0). In particular, as for the data in the financial market, A stable random variable satisfies the following property Mandelbrot [1] firstly argued that the distribution of the price for the scale and the location parameters. A random variable fluctuations of cotton follows a stable law. Since the 1990’s, X follows S(α; β; γ; µ), when there has been a controversy as to whether the central limit 8 theorem or the generalized central limit theorem (GCLT) [11] γX0 + µ if α 6= 1 d < as sums of power-law distributions can be applied to the X = 2 (1) γX + µ + βγ ln γ if α = 1; data of the logarithmic return of stock price fluctuations. In : 0 π particular, Mantegna and Stanley argued that the logarithmic return follows a stable distribution with the power-law index where X0 = S(α; β; 1; 0). When the random variables Xj α < 2 [2], [3], and later they denied their own argument satisfy Xj ∼ S(x; α; βj; γj; 0), the superposition Zn = (X1 + by introducing the cubic laws (α = 3) [4]. Even recently, 1 ··· + Xn)=n α of independent random variables fXjgj=1;··· ;n some researchers [5]–[7] have argued whether a distribution that have different parameters except for α is also in the stable of the logarithmic returns follows power-laws with α > 2 or distribution family as: stable laws with α < 2. On the other hand, it is necessary to ^ prepare very large data sets to elucidate true tail behavior of Zn ∼ S(α; β; γ;^ µ^); (2) distributions [12]. In this respect, the recent study [7] showed that the large and high-frequency arrowhead data of the Tokyo where the parameters β;^ γ^ and µ^ are expressed as: 1 < α < 2 stock exchange (TSE) support stable laws with . 1 Pn β γα (Pn γα ) α In this study, we show such an argument that the sums ^ j=1 j j j=1 j β = Pn α ; γ^ = and of the logarithmic return of multiple stock price fluctuations j=1 γj n follows stable laws can be described from a theoretical back- 0 if α 6= 1 ground. We will extend the GCLT to sums of independent non- µ^ = n − 2 ln n P β γ if α = 1: identical stochastic processes. We call this Super Generalized nπ j=1 j j Central Limit Theorem (SGCLT). We can prove this immediately by the use of the charac- teristic function for the sums of random variables expressed II. SUMMARY OF STABLE DISTRIBUTIONS AND THE as the product of their characteristic functions: GCLT n Y 1 ^ α A probability density function S(x; α; β; γ; µ) of random φ(t; α; β; γ;^ µ^) = φ t=n ; α; βj; γj; 0 : variable X following a stable distribution [13] is defined with j=1 478 j P a g e Future Technologies Conference (FTC) 2017 29-30 November 2017j Vancouver, Canada We focus on the GCLT. Let f of x be a probability density with 'i being a characteristic function of Xi as the ∗ ∗ function of a random variable X for 0 < α < 2: expected value of exp(itXi), and parameters β ; γ ; βi; γi are ( −(α+1) expressed as: c+x for x ! 1 f(x) ' (3) α −(α+1) EC ;C [βiγ ] 1 c−jxj for x ! −∞; ∗ + − i ∗ α α β = α ; γ = EC+;C− [γi ] ; EC+;C− [γi ] 1 with real constants c ; c > 0. Then, according to the α + − c+i − c−i π(c+i + c−i) GCLT [11], the superposition of independent, identically dis- βi = ; γi = πα ; c+i + c−i 2α sin( )Γ(α) tributed random variables X1; ··· ;Xn converges in density to 2 a unique stable distribution S(x; α; β; γ; 0) for n ! 1, that is where EC+;C− [X] denotes the expectation value of X with Pn respect to random parameter distributions Pc+ and Pc− . i=1 Xi − An d Yn = 1 −! S(α; β; γ; 0) for n ! 1; n α 8 ROOF 0 if 0 < α < 1 (4) IV. P < 2 An = n = ln('X (1=n)) if α = 1 Although the following is not mathematically rigorous, we : nE[X] if 1 < α < 2; give the following intuitive proof. The probability distribution function of random variables where 'X is a characteristic function of X as the expected fXjgj=1;··· ;N satisfying the Conditions 1-2 are expressed as: value of exp(itX), E[X] is the expectation value of X, = is an imaginary part of the argument, and parameters β and γ ( c x−(α+1) for x ! +1 are expressed as: f (x) ' +j j −(α+1) 1 c−jjxj for x ! −∞; c − c π(c + c ) α β = + − ; γ = + − ; c + c 2α sin( πα )Γ(α) + − 2 where c+j > 0 and c−j > 0 satisfy E[C+] > 0 and E[C−] > 0. The superposition SN is then defined as: with Γ being the Gamma function. When α = 2, we obtain R 2 R 2 PN µ = xf(x)dx, σ = x f(x)dx and the superposition Yn j=1 Xj − AN of the independent, identically distributed random variables SN = 1 ; N α converges in density to a normal distribution: 8 0 if 0 < α < 1 Pn < PN Xi − nµ d N = ln(' (1=N)) if α = 1 Y = i=1p −!N (0; 1); for n ! 1: AN = j=1 j n nσ : PN j=1 E[Xj] if 1 < α < 2; III. OUR GENERALIZATION where 'j is a characteristic function of Xj. On the We consider an extension of this existing theorem for sums other hand, let N 0 be M × N with some M and of non-identical random variables. In what follows we assume fXijgi=1;··· ;M;j=1;··· ;N be samples given by the same parent that the random variables fXigi=1;··· ;n satisfy the following to Xj for each j. Then fXijgi=1;··· ;M;j=1;··· ;N are indepen- two conditions. dent, identically distributed for i = 1; ··· ;M at a fixed index j. Then, we define the superposition SN 0 as follows: Condition 1: The random variables C+ > 0, C− > 0 M N obey, respectively the distributions Pc (c), Pc (c), and satisfy P P + − i=1 j=1 Xij − AN 0 [C ] < 1, [C ] < 1. S 0 = ; E + E − N 0 1 N α Condition 2: The probability distribution function fi(x) of 8 0 if 0 < α < 1 < the random variables Xi satisfies in 0 < α < 2: 2 PN AN 0 = M N j=1 (= ln('j (1=(MN)))) if α = 1 ( −(α+1) : PN c+ix for x ! 1 M E[Xj] if 1 < α < 2: f (x) ' j=1 i −(α+1) c−ijxj for x ! −∞; Here, we do not consider the convergence of SN in density 0 for N ! 1, but consider the superposition S 0 for N ! 1, where c+i and c−i are samples obtained by C+ and C−. N since the superposition SN will converge to the same limiting The main claim of this paper is the following generalization distribution of SN 0 if SN converges in density. of GCLT: The following superposition Sn of non-identical random variables with power-laws converges in density to a We focus on the convergence in density of SN 0 for M ! unique stable distribution S(x; α; β∗; γ∗; 0) for n ! 1, where 1 and N ! 1 as follows.
Details
-
File Typepdf
-
Upload Time-
-
Content LanguagesEnglish
-
Upload UserAnonymous/Not logged-in
-
File Pages5 Page
-
File Size-