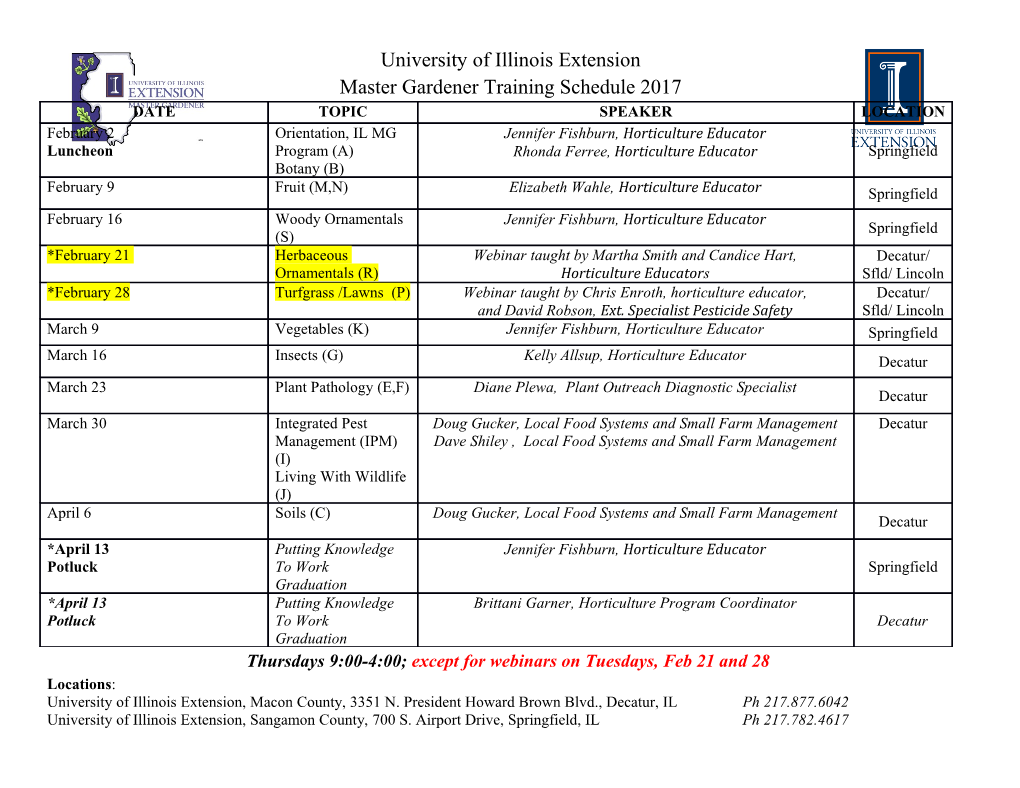
Probabilistic Graphical Models Statistical and Algorithmic Foundations of Deep Learning Eric Xing Lecture 11, February 19, 2020 Reading: see class homepage © Eric Xing @ CMU, 2005-2020 1 ML vs DL © Eric Xing @ CMU, 2005-2020 2 Outline q An overview of DL components q Historical remarks: early days of neural networks q Modern building blocks: units, layers, activations functions, loss functions, etc. q Reverse-mode automatic differentiation (aka backpropagation) q Similarities and differences between GMs and NNs q Graphical models vs. computational graphs q Sigmoid Belief Networks as graphical models q Deep Belief Networks and Boltzmann Machines q Combining DL methods and GMs q Using outputs of NNs as inputs to GMs q GMs with potential functions represented by NNs q NNs with structured outputs q Bayesian Learning of NNs q Bayesian learning of NN parameters q Deep kernel learning © Eric Xing @ CMU, 2005-2020 3 Outline q An overview of DL components q Historical remarks: early days of neural networks q Modern building blocks: units, layers, activations functions, loss functions, etc. q Reverse-mode automatic differentiation (aka backpropagation) q Similarities and differences between GMs and NNs q Graphical models vs. computational graphs q Sigmoid Belief Networks as graphical models q Deep Belief Networks and Boltzmann Machines q Combining DL methods and GMs q Using outputs of NNs as inputs to GMs q GMs with potential functions represented by NNs q NNs with structured outputs q Bayesian Learning of NNs q Bayesian learning of NN parameters q Deep kernel learning © Eric Xing @ CMU, 2005-2020 4 Perceptron and Neural Nets q From biological neuron to artificial neuron (perceptron) Inputs McCulloch & Pitts (1943) x1 Linear Hard Combiner Limiter w1 Output å Y w2 q x2 Threshold q From biological neuron network to artificial neuron networks Synapse Synapse Dendrites Axon Axon i g n a l s l n a g i i g n a l s l n a g i Soma Soma Dendrites tp u n I S O u t u u t p O S Synapse Middle Layer Input Layer Output Layer © Eric Xing @ CMU, 2005-2020 5 The perceptron learning algorithm q Recall the nice property of sigmoid function q Consider regression problem f: XàY, for scalar Y: q We used to maximize the conditional data likelihood q Here … © Eric Xing @ CMU, 2005-2020 6 The perceptron learning algorithm xd = input td = target output od = observed output wi = weight i Incremental mode: Do until converge: § For each training example d in D 1. compute gradient ÑEd[w] Batch mode: 2. Do until converge: where 1. compute gradient ÑED[w] 2. © Eric Xing @ CMU, 2005-2020 7 Neural Network Model Inputs Output Age 34 .6 . S 4 .2 0.6 .1 .5 Gender 2 .2 S .3 .8 .7 S “Probability 4 of Stage .2 beingAlive” Dependent Independent Weights HiddenL Weights variable variables ayer Prediction © Eric Xing @ CMU, 2005-2020 8 “Combined logistic models” Inputs Output Age 34 .6 .5 0.6 .1 Gender 2 S .7 .8 “Probability 4 of Stage beingAlive” Dependent Independent Weights HiddenL Weights variable variables ayer Prediction © Eric Xing @ CMU, 2005-2020 9 “Combined logistic models” Inputs Output Age 34 .5 .2 0.6 Gender 2 .3 S .8 “Probability 4 of Stage .2 beingAlive” Dependent Independent Weights HiddenL Weights variable variables ayer Prediction © Eric Xing @ CMU, 2005-2020 10 “Combined logistic models” Inputs Output Age 34 .6 .5 .2 0.6 .1 Gender 1 .3 S .7 .8 “Probability 4 of Stage .2 beingAlive” Dependent Independent Weights HiddenL Weights variable variables ayer Prediction © Eric Xing @ CMU, 2005-2020 11 Not really, no target for hidden units... Age 34 .6 . S 4 .2 0.6 .1 .5 Gender 2 .2 S .3 .8 .7 S “Probability 4 of Stage .2 beingAlive” Dependent Independent Weights HiddenL Weights variable variables ayer Prediction © Eric Xing @ CMU, 2005-2020 12 Backpropagation: Reverse-mode differentiation q Artificial neural networks are nothing more than complex functional compositions that can be represented by computation graphs: 2 4 @fn @fn @fi1 1 5 = x f(x) @x @f @x Input 3 i1 Outputs i1 ⇡(n) variables 2X Intermediate computations © Eric Xing @ CMU, 2005-2020 13 Backpropagation: Reverse-mode differentiation q Artificial neural networks are nothing more than complex functional compositions that can be represented by computation graphs: 2 4 @fn @fn @fi1 x 1 5 f(x) = 3 @x @fi @x i ⇡(n) 1 12X q By applying the chain rule and using reverse accumulation, we get @f @f @f @f @f @f n = n i1 = n i1 i1 = ... @x @fi1 @x @fi1 @fi2 @x i ⇡(n) i1 ⇡(n) i2 ⇡(i1) 12X 2X 2X q The algorithm is commonly known as backpropagation q What if some of the functions are stochastic? q Then use stochastic backpropagation! (to be covered in the next part) q Modern packages can do this automatically (more later) © Eric Xing @ CMU, 2005-2020 14 Modern building blocks of deep networks x1 w1 q Activation functions w2 f(Wx + b) q Linear and ReLU x2 f q Sigmoid and tanh x w q Etc. 3 3 output output input input Linear Rectified linear (ReLU) © Eric Xing @ CMU, 2005-2020 15 Modern building blocks of deep networks q Activation functions q Linear and ReLU q Sigmoid and tanh q Etc. q Layers fully connected q Fully connected convolutional q Convolutional & pooling q Recurrent q ResNets q Etc. source: recurrent colah.github.io blocks with residual connections © Eric Xing @ CMU, 2005-2020 16 Modern building blocks of deep networks Putting things together: q Activation functions loss q Linear and ReLU activation q Sigmoid and tanh q Etc. q Layers q Fully connected concatenation q Convolutional & pooling q Recurrent q ResNets fully connected q Etc. convolutional q Loss functions q Cross-entropy loss avg& max pooling q Mean squared error q Etc. (a part of GoogleNet) © Eric Xing @ CMU, 2005-2020 17 Modern building blocks of deep networks Putting things together: l Arbitrary combinations of q Activation functions the basic building blocks q Linear and ReLU l Multiple loss functions – q Sigmoid and tanh multi-target prediction, q Etc. transfer learning, and more q Layers l Given enough data, q Fully connected deeper architectures just q Convolutional & pooling keep improving q Recurrent l Representation learning: q ResNets the networks learn increasingly more q Etc. abstract representations q Loss functions of the data that are q Cross-entropy loss “disentangled,” i.e., amenable to linear q Mean squared error separation. q Etc. (a part of GoogleNet) © Eric Xing @ CMU, 2005-2020 18 Feature learning l Arbitrary combinations of q Successful learning of intermediate representations the basic building blocks [Lee et al ICML 2009, Lee et al NIPS 2009] l Multiple loss functions – multi-target prediction, transfer learning, and more l Given enough data, deeper architectures just keep improving l Representation learning: the networks learn increasingly more abstract representations of the data that are “disentangled,” i.e., amenable to linear separation. © Eric Xing @ CMU, 2005-2020 19 Outline q An overview of the DL components q Historical remarks: early days of neural networks q Modern building blocks: units, layers, activations functions, loss functions, etc. q Reverse-mode automatic differentiation (aka backpropagation) q Similarities and differences between GMs and NNs q Graphical models vs. computational graphs q Sigmoid Belief Networks as graphical models q Deep Belief Networks and Boltzmann Machines q Combining DL methods and GMs q Using outputs of NNs as inputs to GMs q GMs with potential functions represented by NNs q NNs with structured outputs q Bayesian Learning of NNs q Bayesian learning of NN parameters q Deep kernel learning © Eric Xing @ CMU, 2005-2020 20 Graphical models vs. Deep nets Graphical models Deep neural networks • Representation for encoding l Learn representations that meaningful knowledge and the facilitate computation and associated uncertainty in a performance on the end-metric graphical form (intermediate representations are not guaranteed to be meaningful) © Eric Xing @ CMU, 2005-2020 21 Graphical models vs. Deep nets Graphical models Deep neural networks q Representation for encoding l Learn representations that meaningful knowledge and the facilitate computation and associated uncertainty in a performance on the end-metric graphical form (intermediate representations are not guaranteed to be meaningful) q Learning and inference are based l Learning is predominantly based on a rich toolbox of well-studied on the gradient descent method (structure-dependent) techniques (aka backpropagation); (e.g., EM, message passing, VI, Inference is often trivial and done MCMC, etc.) via a “forward pass” q Graphs represent models l Graphs represent computation © Eric Xing @ CMU, 2005-2020 22 Graphical models vs. Deep nets Graphical models X1 X2 Utility of the graph log P (X)= log φ(xi)+ log (xi,xj) X3 i i,j q A vehicle for synthesizing a global loss X X function from local structure q potential function, feature function, etc. X5 X4 q A vehicle for designing sound and efficient inference algorithms q Sum-product, mean-field, etc. q A vehicle to inspire approximation and penalization q Structured MF, Tree-approximation, etc. q A vehicle for monitoring theoretical and empirical behavior and accuracy of inference ! " ~$("|') Utility of the loss function q A major measure of quality of the learning algorithm and the model q = argmax $ (') q q © Eric Xing @ CMU, 2005-2020 23 Graphical models vs. Deep nets Deep neural networks Utility of the network l A vehicle to conceptually synthesize complex decision hypothesis l stage-wise projection and aggregation l A vehicle for organizing computational operations l stage-wise update of latent states l A vehicle for designing processing steps and computing modules l Layer-wise parallelization l No obvious utility in evaluating DL inference algorithms Utility of the Loss Function l Global loss? Well it is complex and non- convex..
Details
-
File Typepdf
-
Upload Time-
-
Content LanguagesEnglish
-
Upload UserAnonymous/Not logged-in
-
File Pages81 Page
-
File Size-