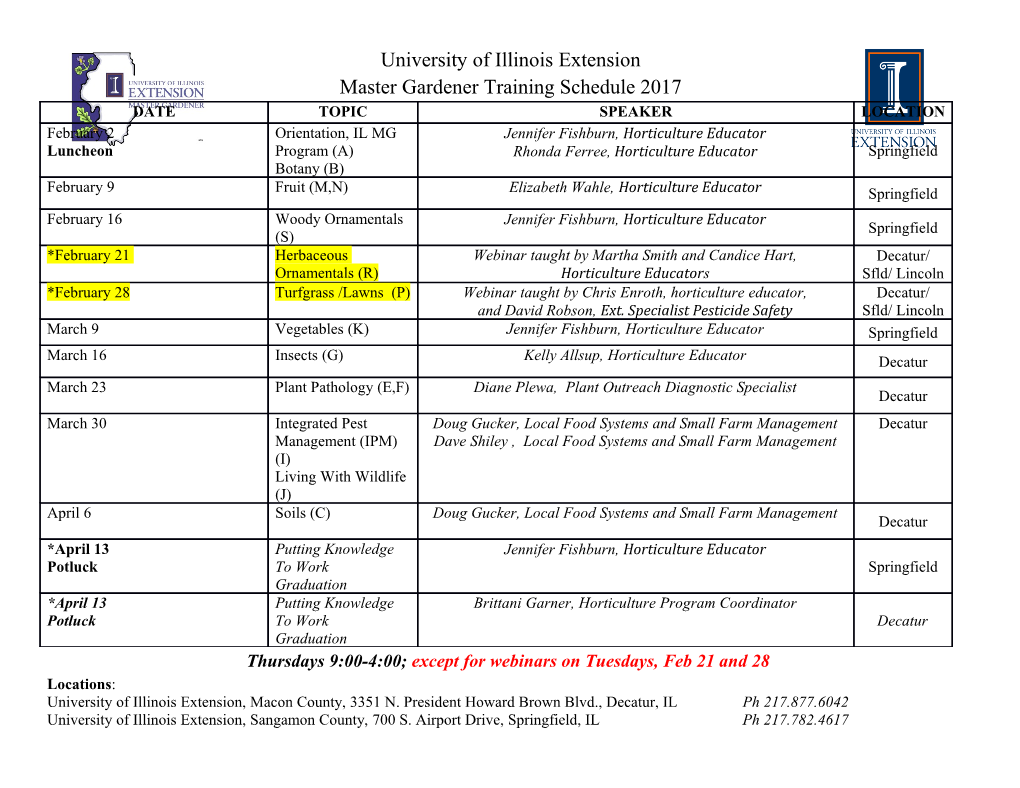
Journal of Statistics and Mathematics ISSN: 0976-8807 & E-ISSN: 0976-8815, Volume 3, Issue 3, 2012, pp.-130-133. Available online at http://www.bioinfo.in/contents.php?id=85 SOME CHARACTERISTIC PROPERTIES OF THE EXPONENTIAL FAMILY ALI A. A-RAHMAN Institute of Statistical Studies and Research, Cairo University, Egypt *Corresponding Author: Email- [email protected] Received: November 05, 2012; Accepted: December 03, 2012 Abstract- In this paper, two recurrence relations characterizing a certain class of distribution family are presented. The first one is a recur- m rence relation between conditional moments of ℎ(X) given X < y. The second is a relationship between the conditional moments E(h (Yk ) |Yk t) m1 th and E(h (Yk ) | Yk t) where Yk is the k order statistic from a sample of size n. Finally the concept of conditional variance of ℎ(Yk) given Yk > t is used to characterize this family. Some results concerning Modified Weibull, Weibull, Rayleigh, exponential, Linear failure rate, 1st type Pear- sonian distributions, Burr, Pareto, Power and uniform distributions are obtained as special cases. Keywords- Characterization, truncated moments, conditional variance, order statistics, recurrence relations, Modified Weibull, Linear failure rate, Pearson distribution of the first type, Burr, and Power distributions. Citation: Ali A. A-Rahman (2012) Some Characteristic Properties of the Exponential Family. Journal of Statistics and Mathematics, ISSN: 0976-8807 & E-ISSN: 0976-8815, Volume 3, Issue 3, pp.-130-133. Copyright: Copyright©2012 Ali A. A-Rahman. This is an open-access article distributed under the terms of the Creative Commons Attribution License, which permits unrestricted use, distribution and reproduction in any medium, provided the original author and source are credited. Introduction Such that: Characterization theorems play a vital role in many fields such as 1. c is a positive constant. mathematical statistics, reliability and actuarial science. They form 2. h(x) is a real valued differentiable function defined on (a, b) an essential tool of statistical inference as they provide characteris- with: lim h(x) h(a) tic properties of distributions that enable researchers to identify the (a) xa particular models. Some excellent references are, e.g., Azlarov and lim h(x) Volodin [6], Galambos and Kotz [11], Kagan, Linnik, and Rao [15] (b) xb and Mchlachlan and Peel [19], among others. (c) E (h(X)) exists and finite. Different methods have been used to identify several types of dis- It is easy to see that several well known distributions (like Modified tributions. Gupta [13], Ouyang [23], Talwalker [26] and Elbatal et. Weibull, Linear failure rate, Rayleigh, exponential, Burr, Pareto,… al.[9], among others have used the concept of right truncated mo- etc) arise from the above family by suitable choices for the function ments to identify some different probability distributions such as h(x), the value of the parameter c and the domain (a, b). Weibull, Burr, Pareto, exponential, power and uniform distributions. In fact characterizations by right truncated moments is very im- Main Results portant in practical since, e.g., in reliability studies some measuring Recurrence relations are very interesting in Statistics. They are devices may be unable to record values greater than time t. Actual- used to reduce the number of operations required to obtain a gen- ly there are some measuring devices that can’t be able to record eral form for the function under study. Moreover, recurrence rela- values smaller than time t. This has motivated several authors to tions together with some initial conditions define functions uniqeu- deal with the problem of characterizing distributions using left trun- ally. This has motivated several authors to use this concept to cated moments, see, e.g., Osaki and Li [22], Ahmed [2],Dimaki and characterize some probability distributions see, e.g., Al-Hussaini et. Xekalaki [7], Navaro et. al. [20] and Gupta [13]. Furthermore, char- al. [5], Ahmad [1], Lin [18], Khan, et al. [17] and Fakhry [10]. acterizations of some particular distributions based on conditional The following Theorem identifies the distribution defined by (1) in variance and conditional moments of order statistics have been terms of a recurrence relation between conditional moments of considered by several authors such as Khan and Beg [16], Pakes hm (X), m = 1,2,… given X < y et al. [24], Fakhry [10], El-Arishi [8], Obretenov [21], Wu and Ouyang [27], Ahsanullah and Nevzorov [4] and Govindarajulu [12], Theorem (A) among others. Let X be an absolutely continuous random variable with cumulative Let X be a continuous random variable with distribution function F distribution function F(·), density function f(·), failure rate λ(·) and (x) defined by : reversed failure rate µ(·) such that F(a) =0 and F(b) = 1 and F(·) h(x) - h(a) has continuous first order derivative on (a,b) with F(x) 0 for all x. F(x) 1 - exp - , x (a, b) c (1) Then, the random variable X has the distribution defined by [Eq-1] Journal of Statistics and Mathematics ISSN: 0976-8807 & E-ISSN: 0976-8815, Volume 3, Issue 3, 2012 Bioinfo Publications 130 Some Characteristic Properties of the Exponential Family iff for any natural number m, the following recurrence relation is Remarks (A) satisfied: 1. If we put (X ) h(X ) h(a) and m = 1 in Theorem (A), we m obtain Talwalker’s result [26]. m (y) m m1 h (a) E(h (X ) | X y) h (y) mcE(h (X ) | X y) (2) 2. If we put m 1, h(X) X, a 0 and b in Theorem (A), we obtain the (y) F(y) result of Elbatal, et. al. [9] concerning the exponential distribu- Proof Necessity tion. By definition The next Theorem gives a recurrence relation between conditional y m m m1 h (x)dF(x) moments of h (Yk ) and h (Yk ) given Yk t m a E(h (X ) | X ) y) F(y) Theorem (B) Integrating by parts and using the fact that F(a) = 0, one gets: Let X be an absolutely continuous random variable with cumulative m y distribution function F(·), survival function G(·) and density function m m m1 E(h (X ) | X y) h (y) h (x)h (x)F(x)dx f(·). Let X1, X2…, Xn be a random sample from F(·). Denote by F(y) a Y1<Y2< …< Yn the corresponding ordered sample. Then, the ran- Using [Eq-1] to eliminate F(x), one gets: dom variable X has the distribution defined by [Eq-1] iff for any y m h(x) h(a) natural number m, the following recurrence relation is satisfied : E(hm (X ) | X y) hm (y) hm1 (x) 1 exp ( ) h(x)dx F(y) a c m m mc m1 y E(h (Yk ) |Yk t) h (t) E(h (Yk ) |Yk t), k 1,2,..., n m m y h(x) h(a) n k 1 (4) hm (y) hm1 (x)d h(x) h m1 (x)exp h(x)dx a F(y) a F(y) c Proof Necessity It is easy to see that The conditional density function of the kth order statistic Yk | Yk > t c f (x) c f (x) (Ahsanullah [3]) is given by: h(x) 1 F(x) 1 (3) n k 1 exp [h(x) h(a)] nk fn (Yk |Yk t) nk1 f (y)[G(y)] y(t,b) (5) c [G(t)] Therefore, Therefore b m m y m n k 1 m nk m m h (y) h (a) m c m1 E(h (Yk ) | Yk t) h (y) f (y)[G(y)] dy E(h (X ) | X y) h (y) h (x) f (x) dx [G(t)]nk1 t F(y) F(y) F(y) a b 1 m nk1 [1 F(y)] hm (a) h (y)d[G(y)] hm (y) m c E(hm1(X ) | X y) [G(t)]nk1 t F(y) F(y) Integrating by parts, recalling that G(b)=0, we get: f (y) f (y) (y) and (y) b Recalling that m m m m1 nk1 1 F(y) F(y) E(h (Yk ) | Yk t) h (t) h (y) h(y)[G(t)] dy [G(t)]nk1 t one gets: m Using [Eq-3] to eliminate h’(y) from the 2nd term, one gets: m (y) m m1 h (a) E(h (X ) | X y) h (y) mc E(h (X ) | X y b m m mc m1 nk (y) F(y) E(h (Yk ) |Yk t) h (t) h (y) f (y)[G(y)] dy [G(t)]nk1 t Sufficiency m mc m1 [Eq-2] can be written as an equation of the unknown function F(y) h (t) E(h (Y ) | Y t) k k as follows: n k 1 y y Sufficiency hm (x) f (x)dx [1 F(y)] hm (y) mc hm1 (x) f (x)dx hm (a) [Eq-4] can be written in the following integral form: a a b b (n - k 1) hm (y) f (y)[G(y)]nk dy hm (t)[G(t)]nk1 mc hm1(y) f (y)[G(y)]nk dy Differentiating both sides with respect to y, cancelling out the term t t hm (y) f (y) from both sides, adding to both sides m c hm1(y) f (y) and Differentiating both sides with respect to t, recalling that f(t) = -G’(t), dividing both sides by mc[1 F (y)]hm1 (y) cancelling out (n k 1) hm (t) f (t)[G(t)]nk from both sides, adding f (y) h'(y) m1 nk mch (t) f (t)[G(t)] to both sides, then dividing both sides we get: m-1 nk1 1 F(y) c by m c h (t)[G(t)] f (t) h(t) one gets: Integrating both sides with respect to y from a to x, noting that F(a) G(t) c = 0 and solving the result for F(x), Integrating both sides with respect to t from a to x, recalling that G h(x) h(a) (a) = 1 and solving the result for G(x), one gets: F(x) 1 exp c h(x)-h(a) we get: G(x) exp - ,x (a,b) c This completes the proof.
Details
-
File Typepdf
-
Upload Time-
-
Content LanguagesEnglish
-
Upload UserAnonymous/Not logged-in
-
File Pages4 Page
-
File Size-