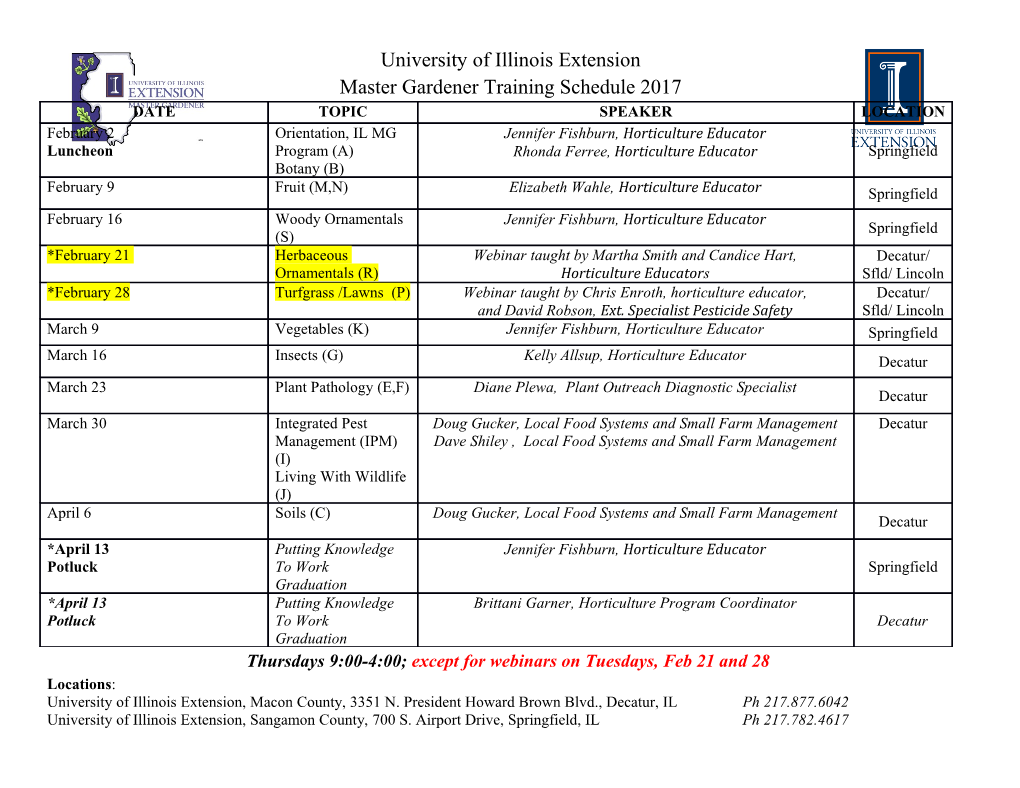
Outline • Review Gaussian Elimination (GE) for solving Ax=b • Optimizing GE for caches on sequential machines - using matrix-matrix multiplication (BLAS and LAPACK) CS 267 • Minimizing communication for sequential GE Dense Linear Algebra: - Not LAPACK, but Recursive LU minimizes bandwidth (latency possible) Parallel Gaussian Elimination • Data layouts on parallel machines • Parallel Gaussian Elimination (ScaLAPACK) • Minimizing communication for parallel GE - Not ScaLAPACK (yet), but “Comm-Avoiding LU” (CALU) - Same idea for minimizing bandwidth and latency in sequential case James Demmel • Summarize rest of dense linear algebra • Dynamically scheduled LU for Multicore www.cs.berkeley.edu/~demmel/cs267_Spr14 • LU for Heterogeneous computers (CPU + GPU) 03/04/2014 CS267 Lecture 13 1 03/04/2014 CS267 Lecture 13 2 Summary of Matrix Multiplication Sca/LAPACK Overview • Goal: Multiply n x n matrices C = A·B using O(n3) arithmetic operations, minimizing data movement • Sequential - Assume fast memory of size M < 3n2, count slow mem. refs. - Thm: need Ω(n3/M1/2) slow mem. refs. and Ω(n3/M3/2) messages - Attainable using “blocked” or “recursive” matrix multiply • Parallel - Assume P processors, O(n2/P) data per processor - Thm: need Ω(n2/P1/2) words sent and Ω(P1/2) messages - Attainable by Cannon, nearly by SUMMA • SUMMA used in practice (PBLAS) - c copies of data ⇒ c1/2 times fewer words, c3/2 fewer messages • Which other linear algebra problems can we do with as little data movement? - Today: Solve Ax=b in detail, summarize what’s known, open3 03/04/2014 CS267 Lecture 13 4 03/04/2014 CS267 Lecture 13 1 Gaussian Elimination (GE) for solving Ax=b Refine GE Algorithm (1) • Add multiples of each row to later rows to make A upper • Initial Version triangular … for each column i • Solve resulting triangular system Ux = c by substitution … zero it out below the diagonal by adding multiples of row i to later rows for i = 1 to n-1 … for each column i … for each row j below row i … zero it out below the diagonal by adding multiples of row i to later rows for j = i+1 to n for i = 1 to n-1 … add a multiple of row i to row j … for each row j below row i tmp = A(j,i); for j = i+1 to n for k = i to n … add a multiple of row i to row j A(j,k) = A(j,k) - (tmp/A(i,i)) * A(i,k) tmp = A(j,i); for k = i to n A(j,k) = A(j,k) - (tmp/A(i,i)) * A(i,k) • Remove computation of constant tmp/A(i,i) from inner loop. for i = 1 to n-1 0 0 0 … 0 . 0 . 0 . 0 for j = i+1 to n . i . 0 . 0 m = A(j,i)/A(i,i) . 0 0 0 0 0 0 0 0 0 0 for k = i to n m 0 0 A(j,k) = A(j,k) - m * A(i,k) After i=1 After i=2 After i=3 After i=n-1 j 03/04/2014 CS267 Lecture 13 5 03/04/2014 CS267 Lecture 13 6 Refine GE Algorithm (2) Refine GE Algorithm (3) • Last version • Last version for i = 1 to n-1 for i = 1 to n-1 for j = i+1 to n for j = i+1 to n m = A(j,i)/A(i,i) m = A(j,i)/A(i,i) for k = i to n for k = i+1 to n A(j,k) = A(j,k) - m * A(i,k) A(j,k) = A(j,k) - m * A(i,k) • Don’t compute what we already know: • Store multipliers m below diagonal in zeroed entries zeros below diagonal in column i for later use for i = 1 to n-1 for i = 1 to n-1 for j = i+1 to n i for j = i+1 to n i m = A(j,i)/A(i,i) A(j,i) = A(j,i)/A(i,i) for k = i+1 to n m for k = i+1 to n m A(j,k) = A(j,k) - m * A(i,k) j A(j,k) = A(j,k) - A(j,i) * A(i,k) j Do not compute zeros Store m here 03/04/2014 CS267 Lecture 13 7 03/04/2014 CS267 Lecture 13 8 2 Refine GE Algorithm (4) Refine GE Algorithm (5) for i = 1 to n-1 • Last version • Last version for j = i+1 to n A(j,i) = A(j,i)/A(i,i) for i = 1 to n-1 for j = i+1 to n for j = i+1 to n for k = i+1 to n A(j,i) = A(j,i)/A(i,i) A(j,k) = A(j,k) - A(j,i) * A(i,k) for k = i+1 to n A(j,k) = A(j,k) - A(j,i) * A(i,k) • Express using matrix operations (BLAS) • Split Loop for i = 1 to n-1 A(i+1:n,i) = A(i+1:n,i) * ( 1 / A(i,i) ) for i = 1 to n-1 … BLAS 1 (scale a vector) A(i+1:n,i+1:n) = A(i+1:n , i+1:n ) for j = i+1 to n i A(j,i) = A(j,i)/A(i,i) - A(i+1:n , i) * A(i , i+1:n) for j = i+1 to n … BLAS 2 (rank-1 update) for k = i+1 to n j A(j,k) = A(j,k) - A(j,i) * A(i,k) Store all m’s here before updating 03/04/2014 CS267 Lecture 13 rest of matrix 9 03/04/2014 CS267 Lecture 13 10 What GE really computes Problems with basic GE algorithm for i = 1 to n-1 for i = 1 to n-1 A(i+1:n,i) = A(i+1:n,i) / A(i,i) … BLAS 1 (scale a vector) A(i+1:n,i) = A(i+1:n,i) / A(i,i) … BLAS 1 (scale a vector) A(i+1:n,i+1:n) = A(i+1:n , i+1:n ) … BLAS 2 (rank-1 update) A(i+1:n,i+1:n) = A(i+1:n , i+1:n ) - A(i+1:n , i) * A(i , i+1:n) … BLAS 2 (rank-1 update) - A(i+1:n , i) * A(i , i+1:n) • Call the strictly lower triangular matrix of multipliers • What if some A(i,i) is zero? Or very small? M, and let L = I+M - Result may not exist, or be “unstable”, so need to pivot • Call the upper triangle of the final matrix U • Current computation all BLAS 1 or BLAS 2, but we know that BLAS 3 (matrix multiply) is fastest (earlier lectures…) • Lemma (LU Factorization): If the above algorithm terminates (does not divide by zero) then A = L*U Peak = * • Solving A*x=b using GE BLAS 3 - Factorize A = L*U using GE (cost = 2/3 n3 flops) - Solve L*y = b for y, using substitution (cost = n2 flops) - Solve U*x = y for x, using substitution (cost = n2 flops) BLAS 2 BLAS 1 • Thus A*x = (L*U)*x = L*(U*x) = L*y = b as desired 03/04/2014 CS267 Lecture 13 11 03/04/2014 CS267 Lecture 13 12 3 Pivoting in Gaussian Elimination Gaussian Elimination with Partial Pivoting (GEPP) • A = [ 0 1 ] fails completely because can’t divide by A(1,1)=0 • Partial Pivoting: swap rows so that A(i,i) is largest in column [ 1 0 ] for i = 1 to n-1 find and record k where |A(k,i)| = max{i ≤ j ≤ n} |A(j,i)| • But solving Ax=b should be easy! … i.e. largest entry in rest of column i if |A(k,i)| = 0 exit with a warning that A is singular, or nearly so • When diagonal A(i,i) is tiny (not just zero), algorithm may elseif k ≠ i terminate but get completely wrong answer swap rows i and k of A • Numerical instability end if • Roundoff error is cause A(i+1:n,i) = A(i+1:n,i) / A(i,i) … each |quotient| ≤ 1 A(i+1:n,i+1:n) = A(i+1:n , i+1:n ) - A(i+1:n , i) * A(i , i+1:n) • Cure: Pivot (swap rows of A) so A(i,i) large • Lemma: This algorithm computes A = P*L*U, where P is a permutation matrix. • This algorithm is numerically stable in practice • For details see LAPACK code at http://www.netlib.org/lapack/single/sgetf2.f • Standard approach – but communication costs? 03/04/2014 CS267 Lecture 13 13 03/04/2014 CS267 Lecture 13 14 Problems with basic GE algorithm Converting BLAS2 to BLAS3 in GEPP • Blocking • What if some A(i,i) is zero? Or very small? - Used to optimize matrix-multiplication - Result may not exist, or be “unstable”, so need to pivot - Harder here because of data dependencies in GEPP • Current computation all BLAS 1 or BLAS 2, but we know that BLAS 3 (matrix multiply) is fastest (earlier lectures…) • BIG IDEA: Delayed Updates for i = 1 to n-1 A(i+1:n,i) = A(i+1:n,i) / A(i,i) … BLAS 1 (scale a vector) - Save updates to “trailing matrix” from several consecutive A(i+1:n,i+1:n) = A(i+1:n , i+1:n ) … BLAS 2 (rank-1 update) BLAS2 (rank-1) updates - A(i+1:n , i) * A(i , i+1:n) - Apply many updates simultaneously in one BLAS3 (matmul) operation Peak • Same idea works for much of dense linear algebra BLAS 3 - Not eigenvalue problems or SVD – need more ideas • First Approach: Need to choose a block size b BLAS 2 - Algorithm will save and apply b updates BLAS 1 - b should be small enough so that active submatrix consisting of b columns of A fits in cache - b should be large enough to make BLAS3 (matmul) fast 03/04/2014 CS267 Lecture 13 15 03/04/2014 CS267 Lecture 13 16 4 Blocked GEPP (www.netlib.org/lapack/single/sgetrf.f) Efficiency of Blocked GEPP (all parallelism “hidden” inside the BLAS) for ib = 1 to n-1 step b … Process matrix b columns at a time end = ib + b-1 … Point to end of block of b columns * = Speed (LAPACK/LU) / Speed(best effort) apply BLAS2 version of GEPP to get A(ib:n , ib:end) = P’ * L’ * U’ 1.2 Speed(Matmul) / HW Peak … let LL denote the strict lower triangular part of A(ib:end , ib:end) + I Speed(LAPACK LU) / Speed(MatMul) A(ib:end , end+1:n) = LL-1 * A(ib:end , end+1:n) … update next b rows of U A(end+1:n , end+1:n ) = A(end+1:n , end+1:n ) 1 - A(end+1:n , ib:end) * A(ib:end , end+1:n) … apply delayed updates with single matrix-multiply 0.8 … with inner dimension b 0.6 Efficiency 0.4 (For a correctness proof, see on-line notes from CS267 / 1996.) 0.2 0 Cnvx C4 Cnvx C4 Cray C90 Cray C90 Alpha RS6000 SGI PC (1 p) (4 p) (1 p) (16 p) 03/04/2014 17 03/04/2014 CS267 Lecture 13 18 CS267 Lecture 13 Communication Lower Bound for GE Does LAPACK’s GEPP Minimize Communication? • Matrix Multiplication can be “reduced to” GE for ib = 1 to n-1 step b … Process matrix b columns at a time end = ib + b-1 … Point to end of block of b columns • Not a good way to do matmul but it shows that GE apply BLAS2 version of GEPP to get A(ib:n , ib:end) = P’ * L’ * U’ needs at least as much communication as matmul … let LL denote the strict lower triangular part of A(ib:end , ib:end) + I A(ib:end , end+1:n) = LL-1 * A(ib:end , end+1:n) … update next b rows of U • Does blocked GEPP minimize communication? A(end+1:n , end+1:n ) = A(end+1:n , end+1:n ) - A(end+1:n , ib:end) * A(ib:end , end+1:n) … apply delayed updates with single matrix-multiply … with inner dimension b • Case 1: n ≥ M - huge matrix – attains lower bound I 0 -B I I 0 -B - b = M1/2 optimal, dominated by matmul A I 0 = A I · I A·B • Case 2: n ≤ M1/2 - small matrix – attains lower bound 0 0 I 0 0 I I - Whole matrix
Details
-
File Typepdf
-
Upload Time-
-
Content LanguagesEnglish
-
Upload UserAnonymous/Not logged-in
-
File Pages34 Page
-
File Size-