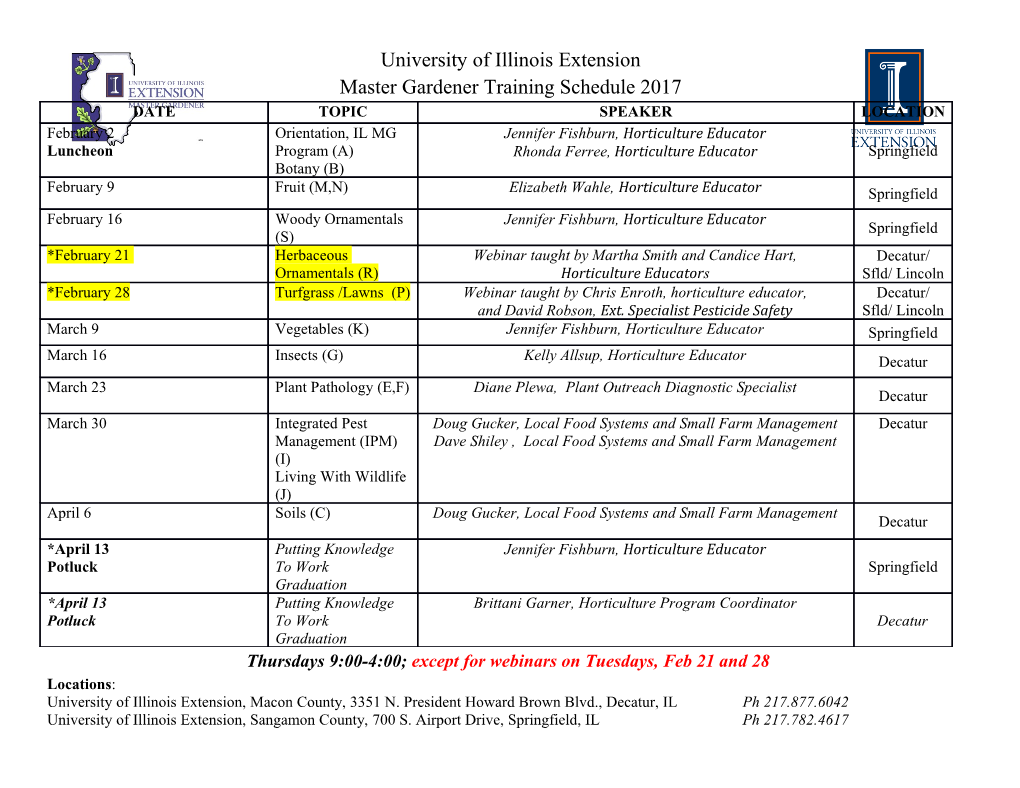
GPU Computing Tutorial M. Schwinzerl, R. De Maria CERN [email protected], [email protected] December 13, 2018 M. Schwinzerl, R. De Maria (CERN) GPU Computing December 13, 2018 1 / 34 Outline • Goal • Identify Problems benefitting from GPU Computing • Understand the typical structure of a GPU enhanced Program • Introduce the two most established Frameworks • Basic Idea about Performance Analysis • Teaser: SixTrackLib • Code: https://github.com/martinschwinzerl/intro_gpu_computing M. Schwinzerl, R. De Maria (CERN) GPU Computing December 13, 2018 2 / 34 Introduction: CPUs vs. GPUs (Spoilers Ahead!) GPUs have: • More arithmetic units (in particular single precision SP) • Less logic for control flow • Less registers per arithmetic units • Less memory but larger bandwidth GPU hardware types: • Low-end Gaming: <100$, still equal or better computing than desktop CPU in SP and DP • High-end Gaming : 100-800$, substantial computing in SP, DP= 1/32 SP • Server HPC: 6000-8000$, substantial computing in SP, DP= 1/2 SP, no video • Hybrid HPC: 3000-8000$, substantial computing in SP, DP= 1/2 SP • Server AI: 6000-8000$, substantial computing in SP, DP= 1/32 SP, no video M. Schwinzerl, R. De Maria (CERN) GPU Computing December 13, 2018 3 / 34 Introduction: GPU vs CPU (Example) Specification CPU GPU Cores or compute units 2-64 16-80 Arithmetic units per core 2-8 64 GFlops SP 24-2000 1000 - 15000 GFlops DP 12-1000 100 - 7500 • Obtaining theoretical performance is guaranteed only for a limited number of problems • Code complexity, Number of temporary varaibles, Branches, Latencies, Memory access patterns / Synchronization, .... • Question 1: How to write and run programs for GPUs? • Question 2: What kind of problems are suitable for GPU computing? M. Schwinzerl, R. De Maria (CERN) GPU Computing December 13, 2018 4 / 34 Example: Non-Scary GPU programming using CuPy1 import cupy as cp import numpy as np N = 100000 # length of the vectors # create vectors of random numbers and transfer them to the GPU: x = cp.asarray( np.random.rand( N ) ) y = cp.asarray( np.random.rand( N ) ) # perform the calculations on the GPU: z = x + y # transfer the result back from the GPU z cmp = z.asnumpy( z ) 1CuPy: Cuda + Python https://cupy.chainer.org/ M. Schwinzerl, R. De Maria (CERN) GPU Computing December 13, 2018 5 / 34 Overview: Coding frameworks • Cuda: NVIDIA, GPUs only, C99 / C++1x language, unified memory (free) • OpenCL: vendor and hardware neutral (AMD, NVIDIA, Intel, CPU(pocl, Intel)), v1.2: C99-like language (free) • SyCL: open standard, beta commercial implementation (Intel, AMD) (no NVIDIA) • ROCm: open source (from AMD) (HIP compatible AMD, Nvidia) • clang+SPIRV+vulkan: vendor neutral (AMD, NVIDIA) not easy • clang+PTX: Nvidia not easy • OpenACC and OpenMP: directive based, offloading, open standard, compiler directives • numba, cupy (pure python) • pyopencl (python + OpenCL kernels), pycuda (python + Cuda kernels) M. Schwinzerl, R. De Maria (CERN) GPU Computing December 13, 2018 6 / 34 Comparison: OpenCL and Cuda OpenCL Cuda • Hardware Neutral: GPUs, CPUs, FPGAs, • Hardware: only NVIDIA GPUs Signal Processors • Software: only implementation from • Standardized by Khronos Group NVIDIA, • Programs should work across • Tools, Libraries and Toolkits also available implementations ! \Least Common • Software and hardware tightly integrated Denominator' Programming" (versioning!) • Now: OpenCL 1.2 (C99 - like language • No emulator/CPU target on recent for device programs), future: OpenCL implementations 2.x, OpenCL-Next? • Version 10.0 with C99 and C++11/14 • Primarily: Run-time compilation like language • Single-file compilation (SyCL) • Primarily: Single-file compilation • Run-time compilation available (NVRTC) M. Schwinzerl, R. De Maria (CERN) GPU Computing December 13, 2018 7 / 34 Example: Vector-Vector Addition (Overview) M. Schwinzerl, R. De Maria (CERN) GPU Computing December 13, 2018 8 / 34 Example: Vector-Vector Addition (Kernel, SPMD) M. Schwinzerl, R. De Maria (CERN) GPU Computing December 13, 2018 9 / 34 Detail: OpenCL and Cuda Kernels for ~z = ~x + ~y OpenCL Kernel __kernel void add_vec_kernel( __global double const* restrictx, __global double const* restricty, __global double* restrictz, int constn) { int const gid= get_global_id( 0 ); if( gid<n)z[ gid]=x[ gid]+y[ gid]; } Cuda Kernel __global__ void add_vec_kernel( double const* __restrict__x, double const* __restrict__y, double* __restrict__z, int constn) { int const gid= blockIdx.x* blockDim.x+ threadIdx.x; if( gid<n)z[ gid]=x[ gid]+y[ gid]; } M. Schwinzerl, R. De Maria (CERN) GPU Computing December 13, 2018 10 / 34 OpenCL: Building the Program, Getting the Kernel /*---------------------------------------------------------------------*/ /* Build the program and get the kernel:*/ cl::Context context( device); cl::CommandQueue queue( context, device); std::string const kernel_source= "__kernel void add_vec_kernel(\r\n" " __global double const* restrictx, __global double const* restricty," " __global double* restrictz, int constn)\r\n" "{\r\n" " int const gid= get_global_id(0);\r\n" " if( gid<n){\r\n" "z[ gid]=x[ gid]+y[ gid];\r\n" "}\r\n" "}\r\n"; std::string const compile_options="-w-Werror"; cl::Program program( context, kernel_source); cl_int ret= program.build( compile_options.c_str()); assert( ret ==CL_SUCCESS); cl::Kernel kernel( program,"add_vec_kernel"); M. Schwinzerl, R. De Maria (CERN) GPU Computing December 13, 2018 11 / 34 Example: Vector-Vector Addition (SPMD,Teams,Grid) • Internally: Groups of W Threads operating in lock-step • NVidia: Warp, W ∼ 32 • AMD: Wavefront, W ∼ 64 • Other: Team, .... • For the user, threads are organized in a 3D geometrical structure • Cuda: Grid (Blocks, Threads) • OpenCL: Work-groups of Threads in a 3D grid • The 3D grid is motivated by image (video) applications • Flexible ! can be treated like a 1D grid M. Schwinzerl, R. De Maria (CERN) GPU Computing December 13, 2018 12 / 34 Example: Vector-Vector Addition (SPMD, Branching) One Consequence of SPMD: Branching can be Expensive Example below: One Wave of W threads: M. Schwinzerl, R. De Maria (CERN) GPU Computing December 13, 2018 13 / 34 M. Schwinzerl, R. De Maria (CERN) GPU Computing December 13, 2018 14 / 34 OpenCL: On the Host: prepare Device, Context, Queue /*---------------------------------------------------------------------*/ /* Select the first device on the first platform:*/ std::vector< cl::Platform> platforms; cl::Platform::get(&platforms); std::vector< cl::Device> devices; bool device_found= false; cl::Device device; for( auto const& available_platform: platforms) { devices.clear(); available_platform.getDevices(CL_DEVICE_TYPE_ALL,&devices); if(!devices.empty()) { device= devices.front(); device_found= true; break; } } assert( device_found); /*---------------------------------------------------------------------*/ /* Build the program and get the kernel:*/ cl::Context context( device); cl::CommandQueue queue( context, device); M. Schwinzerl, R. De Maria (CERN) GPU Computing December 13, 2018 15 / 34 Cuda: Just checking .... /*---------------------------------------------------------------------*/ /* use the"default"/"first" Cuda device for the program:*/ int device= int{ 0 }; ::cudaGetDevice(&device); cudaError_t cu_err = ::cudaDeviceSynchronize(); assert( cu_err == ::cudaSuccess); M. Schwinzerl, R. De Maria (CERN) GPU Computing December 13, 2018 16 / 34 Allocating the Buffers on the Device OpenCL: /*---------------------------------------------------------------------*/ /* Allocate the buffers on the device*/ /* x_arg, y_arg, z_arg... handles on the host side managing buffers in* * the device memory*/ cl::Buffer x_arg( context,CL_MEM_READ_WRITE, sizeof( double)*N, nullptr); cl::Buffer y_arg( context,CL_MEM_READ_WRITE, sizeof( double)*N, nullptr); cl::Buffer z_arg( context,CL_MEM_READ_WRITE, sizeof( double)*N, nullptr); Cuda: /*---------------------------------------------------------------------*/ /* Allocate the buffers on the device*/ /* x_arg, y_arg, z_arg... handles on the host side managing buffers in* * the device memory*/ double* x_arg= nullptr; double* y_arg= nullptr; double* z_arg= nullptr; ::cudaMalloc(&x_arg, sizeof( double)*N); ::cudaMalloc(&y_arg, sizeof( double)*N); ::cudaMalloc(&z_arg, sizeof( double)*N); M. Schwinzerl, R. De Maria (CERN) GPU Computing December 13, 2018 17 / 34 Transfering ~x and ~y to the Device Memory OpenCL: /* Transferx andy from the host to the device*/ ret= queue.enqueueWriteBuffer( x_arg,CL_TRUE, std::size_t{ 0 }, x.size()* sizeof( double),x.data()); assert( ret ==CL_SUCCESS); ret= queue.enqueueWriteBuffer( y_arg,CL_TRUE, std::size_t{ 0 }, y.size()* sizeof( double),y.data()); assert( ret ==CL_SUCCESS); Cuda: /*---------------------------------------------------------------------*/ /* Transferx andy from host to device*/ ::cudaMemcpy( x_arg,x.data(), sizeof( double)*N, cudaMemcpyHostToDevice); ::cudaMemcpy( y_arg,y.data(), sizeof( double)*N, cudaMemcpyHostToDevice); M. Schwinzerl, R. De Maria (CERN) GPU Computing December 13, 2018 18 / 34 Running the kernel OpenCL: /*---------------------------------------------------------------------*/ /* Prepare the kernel for execution: bind the arguments to the kernel*/ kernel.setArg( 0, x_arg); kernel.setArg( 1, y_arg); kernel.setArg( 2, z_arg); kernel.setArg( 3,N); /*--------------------------------------------------------------------*/ /* execute the kernel on the device*/ cl::NDRange offset= cl::NullRange; cl::NDRange local= cl::NullRange; ret= queue.enqueueNDRangeKernel( kernel, offset,N, local);
Details
-
File Typepdf
-
Upload Time-
-
Content LanguagesEnglish
-
Upload UserAnonymous/Not logged-in
-
File Pages34 Page
-
File Size-