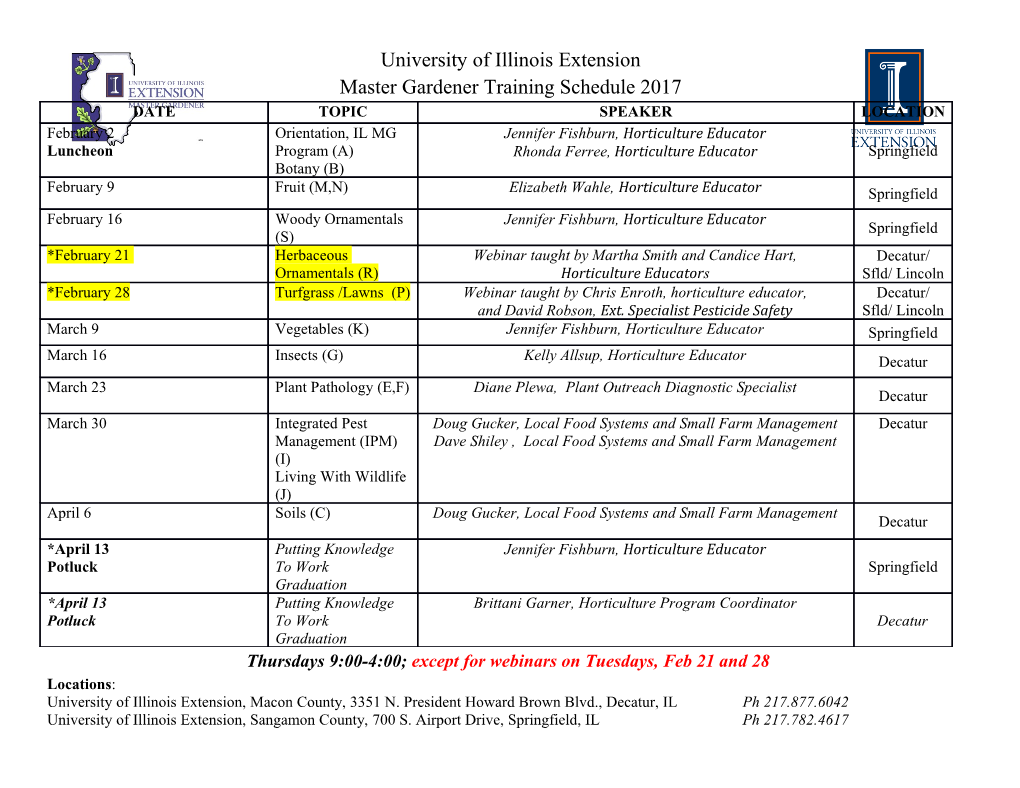
Announcements • HW2 will be posted later today – Constructing a mosaic by warping images. Filtering Computer Vision I CSE252A Lecture 9 CS252A, Winter 2005 Computer Vision I CS252A, Winter 2005 Computer Vision I Mosaic Image Filtering CS252A, Winter 2005 Computer Vision I CS252A, Winter 2005 Computer Vision I Noise • Simplest noise model • Issues – independent stationary – this model allows noise values that could be greater than additive Gaussian noise maximum camera output or – the noise value at each less than zero pixel is given by an – for small standard deviations, independent draw from this isn’t too much of a the same normal problem - it’s a fairly good model probability distribution – independence may not be justified (e.g. damage to lens) – may not be stationary (e.g. thermal gradients in the ccd) (From Bill Freeman) CS252A, Winter 2005 Computer Vision I CS252A, Winter 2005 Computer Vision I 1 Linear Filters • General process: • Example: smoothing by – Form new image whose pixels averaging are a weighted sum of original – form the average of pixels in a pixel values, using the same neighbourhood set of weights at each point. • Example: smoothing with a • Properties Gaussian – Output is a linear function of – form a weighted average of the input pixels in a neighbourhood – Output is a shift-invariant function of the input (i.e. shift • Example: finding a the input image two pixels to derivative the left, the output is shifted – form a weighted average of two pixels to the left) pixels in a neighbourhood (Freeman) CS252A, Winter 2005 Computer Vision I CS252A, Winter 2005 Computer Vision I Convolution Convolution: R= K*I m=2 1 2 1 I R * -1 -2 -1 Kernel (K) Note: Typically Kernel m/2 m/2 is relatively small in Kernel size Image (I) R(i, j) = ∑∑K(h,k)I(i −h, j −k) vision applications. is m+1 by m+1 h=−−m/2 k= m/2 CS252A, Winter 2005 Computer Vision I CS252A, Winter 2005 Computer Vision I Convolution: R= K*I Convolution: R= K*I m=2 m=2 I R I R m/2 m/2 m/2 m/2 Kernel size R(i, j) = ∑∑K(h,k)I(i −h, j −k) Kernel size R(i, j) = ∑∑K(h,k)I(i −h, j −k) is m+1 by m+1 h=−−m/2 k= m/2 is m+1 by m+1 h=−−m/2 k= m/2 CS252A, Winter 2005 Computer Vision I CS252A, Winter 2005 Computer Vision I 2 Convolution: R= K*I Convolution: R= K*I m=2 m=2 I R I R m/2 m/2 m/2 m/2 Kernel size R(i, j) = ∑∑K(h,k)I(i −h, j −k) Kernel size R(i, j) = ∑∑K(h,k)I(i −h, j −k) is m+1 by m+1 h=−−m/2 k= m/2 is m+1 by m+1 h=−−m/2 k= m/2 CS252A, Winter 2005 Computer Vision I CS252A, Winter 2005 Computer Vision I Convolution: R= K*I Convolution: R= K*I m=2 m=2 I R I R m/2 m/2 m/2 m/2 Kernel size R(i, j) = ∑∑K(h,k)I(i −h, j −k) Kernel size R(i, j) = ∑∑K(h,k)I(i −h, j −k) is m+1 by m+1 h=−−m/2 k= m/2 is m+1 by m+1 h=−−m/2 k= m/2 CS252A, Winter 2005 Computer Vision I CS252A, Winter 2005 Computer Vision I Convolution: R= K*I Convolution: R= K*I m=2 m=2 I R I R m/2 m/2 m/2 m/2 Kernel size R(i, j) = ∑∑K(h,k)I(i −h, j −k) Kernel size R(i, j) = ∑∑K(h,k)I(i −h, j −k) is m+1 by m+1 h=−−m/2 k= m/2 is m+1 by m+1 h=−−m/2 k= m/2 CS252A, Winter 2005 Computer Vision I CS252A, Winter 2005 Computer Vision I 3 Convolution: R= K*I Convolution: R= K*I m=2 m=2 I R I R m/2 m/2 m/2 m/2 Kernel size R(i, j) = ∑∑K(h,k)I(i −h, j −k) Kernel size R(i, j) = ∑∑K(h,k)I(i −h, j −k) is m+1 by m+1 h=−−m/2 k= m/2 is m+1 by m+1 h=−−m/2 k= m/2 CS252A, Winter 2005 Computer Vision I CS252A, Winter 2005 Computer Vision I Impulse Response 0000 000000 000 00000000 000 1 1 *= CS252A, Winter 2005 Computer Vision I CS252A, Winter 2005 (Swiped from Bill Freeman) Computer Vision I CS252A, Winter 2005 (Swiped from Bill Freeman) Computer Vision I CS252A, Winter 2005 (Swiped from Bill Freeman) Computer Vision I 4 CS252A, Winter 2005 (Swiped from Bill Freeman) Computer Vision I CS252A, Winter 2005 (Swiped from Bill Freeman) Computer Vision I CS252A, Winter 2005 (Swiped from Bill Freeman) Computer Vision I CS252A, Winter 2005 Computer Vision I CS252A, Winter 2005 Computer Vision I CS252A, Winter 2005 (Swiped from Bill Freeman) Computer Vision I 5 CS252A, Winter 2005 (Swiped from Bill Freeman) Computer Vision I CS252A, Winter 2005 (Swiped from Bill Freeman) Computer Vision I CS252A, Winter 2005 Computer Vision I CS252A, Winter 2005 Computer Vision I Smoothing by Averaging Kernel: CS252A, Winter 2005 Computer Vision I CS252A, Winter 2005 Computer Vision I 6 Filtering to reduce noise Additive noise • I = S + N. Noise doesn’t depend on signal. • Noise is what we’re not interested in. • We’ll consider: – We’ll discuss simple, low-level noise today: Light fluctuations; Sensor noise; Quantization effects; Finite precision Ii = si + ni with E(ni ) = 0 – Not complex: shadows; extraneous objects. si deterministic. • A pixel’s neighborhood contains ni ,n j independent for ni ≠ n j information about its intensity. ni ,n j identically distributed • Averaging noise reduces its effect. CS252A, Winter 2005 Computer Vision I CS252A, Winter 2005 Computer Vision I Guassian Noise: sigma=1 Guassian Noise: sigma=16 CS252A, Winter 2005 Computer Vision I CS252A, Winter 2005 Computer Vision I Does it reduce noise? Average Filter • Mask with positive • Intuitively, takes out small variations. entries, that sum 1. ˆ F I (i, j) = I (i, j) + N (i, j) with N(i, j) ~ N(0, σ ) • Replaces each pixel m / 2 m / 2 1 ˆ with an average of 1 1 1 O(i, j) = 2 ∑∑I (i − h, j − k ) + N (i − h, j − k ) = m h =−− m / 2 k = m / 2 its neighborhood. 1 1/9 1 1 1 m / 2 m / 2 1 m / 2 m / 2 = Iˆ(i − h, j − k ) + N (i − h, j − k ) • If all weights are 1 1 1 m2 ∑∑m2 ∑∑ h =−− m / 2 k =−m / 2 142h =−4m / 24k =4m / 2 434 4 4 equal, it is called a Nˆ (i , j ) BOX filter. E ( Nˆ (i, j)) = 0 2 ˆ 2 1 2 σ ˆ σ (Camps) E ( N (i, j)) = 2 mσ = ⇒ N (i, j) ~ N (0, ) m m m CS252A, Winter 2005 Computer Vision I CS252A, Winter 2005 (Camps) Computer Vision I 7 Smoothing with a Gaussian Smoothing by Averaging Kernel: • Notice “ringing” – apparently, a grid is superimposed • Smoothing with an average actually doesn’t compare at all well with a defocussed lens – what does a point of light produce? • A Gaussian gives a good model of a fuzzy blob CS252A, Winter 2005 Computer Vision I CS252A, Winter 2005 Computer Vision I An Isotropic Gaussian Smoothing with a Gaussian Kernel: • The picture shows a smoothing kernel proportional to x2 + y2 exp − 2 2σ (which is a reasonable model of a circularly symmetric fuzzy blob) CS252A, Winter 2005 Computer Vision I CS252A, Winter 2005 Computer Vision I Efficient Implementation The effects of smoothing Each row shows smoothing • Both, the BOX filter and the Gaussian filter with gaussians of different are separable: width; each column shows different realizations of – First convolve each row with a 1D filter an image of gaussian noise. – Then convolve each column with a 1D filter. CS252A, Winter 2005 Computer Vision I CS252A, Winter 2005 Computer Vision I 8 Fourier Tansform Fourier basis element Discrete Fourier Transform (DFT) of I[x,y] e−i2π (ux+vy) Transform is sum of orthogonal basis functions Vector (u,v) • Magnitude gives frequency • Direction gives orientation. Inverse DFT x,y: spatial domain u,v: frequence domain Implemented via the “Fast Fourier Transform” algorithm (FFT) CS252A, Winter 2005 Computer Vision I CS252A, Winter 2005 Computer Vision I And larger still... Here u and v are larger than in the previous slide. CS252A, Winter 2005 Computer Vision I CS252A, Winter 2005 Computer Vision I Using Fourier Representations Dominant Orientation Limitations: not useful for local segmentation CS252A, Winter 2005 Computer Vision I 9.
Details
-
File Typepdf
-
Upload Time-
-
Content LanguagesEnglish
-
Upload UserAnonymous/Not logged-in
-
File Pages9 Page
-
File Size-