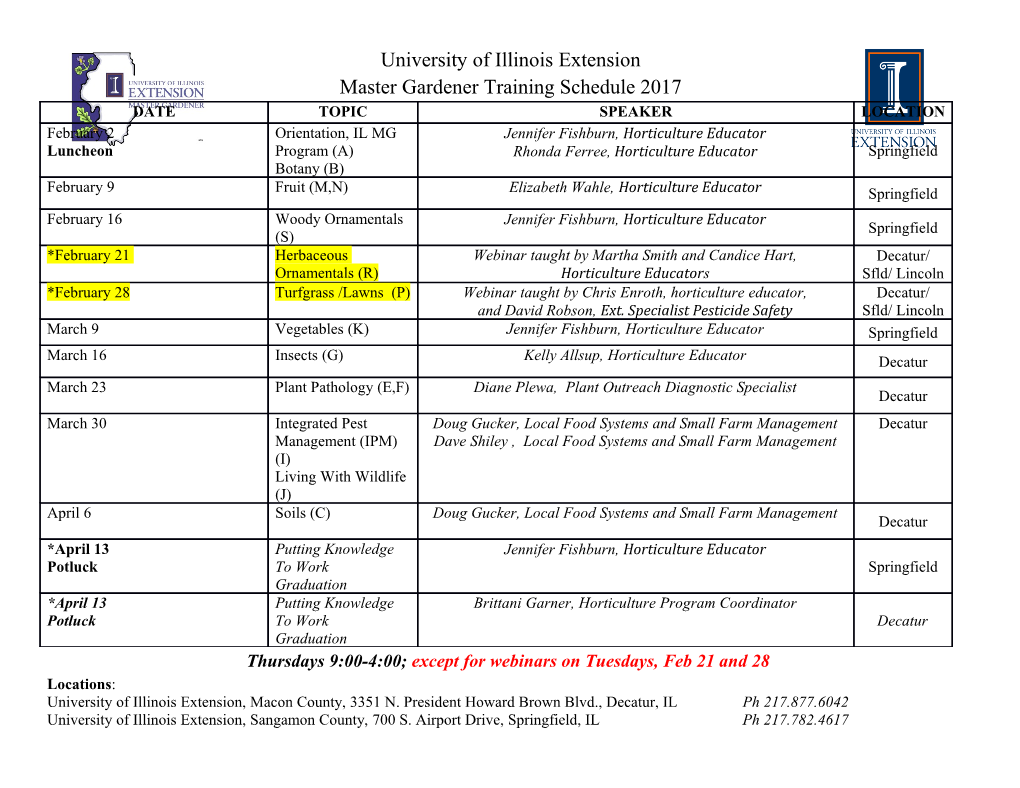
STATISTICAL SCIENCE Volume 33, Number 3 August 2018 A Review of Self-Exciting Spatio-Temporal Point Processes and Their Applications .................................................................................Alex Reinhart 299 Comment on “A Review of Self-Exciting Spatiotemporal Point Process and Their Applications”byAlexReinhart.............................................Yosihiko Ogata 319 Comment on “A Review of Self-Exciting Spatio-Temporal Point Process and Their Applications”byAlexReinhart..........................................Jiancang Zhuang 323 Comment on “A Review of Self-Exciting Spatio-Temporal Point Processes and Their Applications”byAlexReinhart..................................Frederic Paik Schoenberg 325 Self-Exciting Point Processes: Infections and Implementations .............Sebastian Meyer 327 Rejoinder: A Review of Self-Exciting Spatio-Temporal Point Processes and Their Applications..................................................................Alex Reinhart 330 On the Relationship between the Theory of Cointegration and the Theory of Phase Synchronization..................Rainer Dahlhaus, István Z. Kiss and Jan C. Neddermeyer 334 Confidentiality and Differential Privacy in the Dissemination of Frequency Tables ......................Yosef Rinott, Christine M. O’Keefe, Natalie Shlomo and Chris Skinner 358 Piecewise Deterministic Markov Processes for Continuous-Time Monte Carlo .....................Paul Fearnhead, Joris Bierkens, Murray Pollock and Gareth O. Roberts 386 Fractionally Differenced Gegenbauer Processes with Long Memory: A Review ................................................G. S. Dissanayake, M. S. Peiris and T. Proietti 413 A Unified Theory of Confidence Regions and Testing for High-Dimensional Estimating Equations...............................Matey Neykov, Yang Ning, Jun S. Liu and Han Liu 427 AConversationwithTomLouis................................................Lance A. Waller 444 AConversationwithJimPitman.................................................David Aldous 458 Statistical Science [ISSN 0883-4237 (print); ISSN 2168-8745 (online)], Volume 33, Number 3, August 2018. Published quarterly by the Institute of Mathematical Statistics, 3163 Somerset Drive, Cleveland, OH 44122, USA. Periodicals postage paid at Cleveland, Ohio and at additional mailing offices. POSTMASTER: Send address changes to Statistical Science, Institute of Mathematical Statistics, Dues and Subscriptions Office, 9650 Rockville Pike—Suite L2310, Bethesda, MD 20814-3998, USA. Copyright © 2018 by the Institute of Mathematical Statistics Printed in the United States of America Statistical Science Volume 33, Number 3 (299–467) August 2018 Volume 33 Number 3 August 2018 A Review of Self-Exciting Spatio-Temporal Point Processes and Their Applications Alex Reinhart On the Relationship between the Theory of Cointegration and the Theory of Phase Synchronization Rainer Dahlhaus, István Z. Kiss and Jan C. Neddermeyer Confidentiality and Differential Privacy in the Dissemination of Frequency Tables Yosef Rinott, Christine M. O’Keefe, Natalie Shlomo and Chris Skinner Piecewise Deterministic Markov Processes for Continuous-Time Monte Carlo Paul Fearnhead, Joris Bierkens, Murray Pollock and Gareth O. Roberts Fractionally Differenced Gegenbauer Processes with Long Memory: A Review G. S. Dissanayake, M. S. Peiris and T. Proietti A Unified Theory of Confidence Regions and Testing for High Dimensional Estimating Equations Matey Neykov, Yang Ning, Jun S. Liu and Han Liu A Conversation with Tom Louis Lance A. Waller A Conversation with Jim Pitman David Aldous EDITOR Cun-Hui Zhang Rutgers University ASSOCIATE EDITORS Peter Bühlmann Peter Müller Eric Tchetgen Tchetgen ETH Zürich University of Texas Harvard School of Public Jiahua Chen Sonia Petrone Health University of British Columbia Bocconi University Alexandre Tsybakov Rong Chen Nancy Reid Université Paris 6 Rutgers University University of Toronto Jon Wakefield Rainer Dahlhaus Jason Roy University of Washington University of Heidelberg University of Pennsylvania Jon Wellner Robin Evans Richard Samworth University of Washington University of Oxford University of Cambridge Yihong Wu Edward I. George Bodhisattva Sen Yale University University of Pennsylvania Columbia University Minge Xie Peter Green Glenn Shafer Rutgers University University of Bristol and Rutgers Business Bin Yu University of Technology School–Newark and University of California, Sydney New Brunswick Berkeley Theo Kypraios Royal Holloway College, Ming Yuan University of Nottingham University of London University of Steven Lalley David Siegmund Wisconsin-Madison University of Chicago Stanford University Tong Zhang Ian McKeague Dylan Small Tencent AI Lab Columbia University University of Pennsylvania Harrison Zhou Vladimir Minin Michael Stein Yale University University of California, Irvine University of Chicago MANAGING EDITOR T. N. Sriram University of Georgia PRODUCTION EDITOR Patrick Kelly EDITORIAL COORDINATOR Kristina Mattson PAST EXECUTIVE EDITORS Morris H. DeGroot, 1986–1988 Morris Eaton, 2001 Carl N. Morris, 1989–1991 George Casella, 2002–2004 Robert E. Kass, 1992–1994 Edward I. George, 2005–2007 Paul Switzer, 1995–1997 David Madigan, 2008–2010 Leon J. Gleser, 1998–2000 Jon A. Wellner, 2011–2013 Richard Tweedie, 2001 Peter Green, 2014–2016 Statistical Science 2018, Vol. 33, No. 3, 299–318 https://doi.org/10.1214/17-STS629 © Institute of Mathematical Statistics, 2018 A Review of Self-Exciting Spatio-Temporal Point Processes and Their Applications Alex Reinhart Abstract. Self-exciting spatio-temporal point process models predict the rate of events as a function of space, time, and the previous history of events. These models naturally capture triggering and clustering behavior, and have been widely used in fields where spatio-temporal clustering of events is ob- served, such as earthquake modeling, infectious disease, and crime. In the past several decades, advances have been made in estimation, inference, sim- ulation, and diagnostic tools for self-exciting point process models. In this review, I describe the basic theory, survey related estimation and inference techniques from each field, highlight several key applications, and suggest directions for future research. Key words and phrases: Epidemic-Type Aftershock Sequence, conditional intensity, Hawkes process, stochastic declustering. REFERENCES BRAY,A.andSCHOENBERG, F. P. (2013). Assessment of point process models for earthquake forecasting. Statist. Sci. 28 510– ADELFIO,G.andCHIODI, M. (2015a). Alternated estimation 520. MR3161585 in semi-parametric space–time branching-type point processes BRAY,A.,WONG,K.,BARR,C.D.andSCHOENBERG,F.P. with application to seismic catalogs. Stoch. Environ. Res. Risk (2014). Voronoi residual analysis of spatial point process mod- Assess. 29 443–450. els with applications to California earthquake forecasts. Ann. ADELFIO,G.andCHIODI, M. (2015b). FLP estimation of semi- Appl. Stat. 8 2247–2267. MR3292496 parametric models for space–time point processes and diagnos- BROCKMANN,D.,HUFNAGEL,L.andGEISEL, T. (2006). The tic tools. Spat. Stat. 14 119–132. MR3429716 scaling laws of human travel. Nature 439 462–465. BACRY,E.,MASTROMATTEO,I.andMUZY, J.-F. (2015). Hawkes processes in finance. Mark. Microstruct. Liq. 1 1550005. CHEN,J.M.,HAWKES,A.G.,SCALAS,E.andTRINH,M. (2017). Performance of information criteria used for model se- BADDELEY,A.,MØLLER,J.andPAKES, A. G. (2007). Properties of residuals for spatial point processes. Ann. Inst. Statist. Math. lection of Hawkes process models of financial data. Available at 60 627–649. arXiv:1702.06055. HIODI DELFIO BADDELEY,A.,RUBAK,E.andMØLLER, J. (2011). Score, C ,M.andA , G. (2011). Forward likelihood-based pseudo-score and residual diagnostics for spatial point process predictive approach for space–time point processes. Environ- models. Statist. Sci. 26 613–646. metrics 22 749–757. MR2843141 BADDELEY,A.,TURNER,R.,MØLLER,J.andHAZELTON,M. CLARKE,R.V.andCORNISH, D. B. (1985). Modeling offenders’ (2005). Residual analysis for spatial point processes. J. R. Stat. decisions: A framework for research and policy. Crime Justice Soc. Ser. B. Stat. Methodol. 67 617–666. MR2210685 6 147–185. BAUWENS,L.andHAUTSCH, N. (2009). Modelling financial high CLEMENTS,R.A.,SCHOENBERG,F.P.andVEEN, A. (2012). frequency data using point processes. In Handbook of Financial Evaluation of space–time point process models using super- Time Series (T. Mikosch, J.-P. Kreiß, R. A. Davis and T. G. An- thinning. Environmetrics 23 606–616. dersen, eds.) 953–979. Springer, Berlin. COHEN,L.E.andFELSON, M. (1979). Social change and crime BERNASCO,W.,JOHNSON,S.D.andRUITER, S. (2015). Learn- rate trends: A routine activity approach. Am. Sociol. Rev. 44 ing where to offend: Effects of past on future burglary locations. 588–608. Appl. Geogr. 60 120–129. COHEN,J.,GORR,W.L.andOLLIGSCHLAEGER, A. M. (2007). BRAGA,A.A.,PAPACHRISTOS,A.V.andHUREAU,D.M. Leading indicators and spatial interactions: A crime-forecasting (2014). The effects of hot spots policing on crime: An updated model for proactive police deployment. Geogr. Anal. 39 105– systematic review and meta-analysis. Justice Q. 31 633–663. 127. Alex Reinhart is a Ph.D. Student, Department of Statistics & Data Science, Carnegie Mellon University, 5000 Forbes Ave, Pittsburgh, Pennsylvania 15213, USA (e-mail: [email protected]). COWLING,A.andHALL, P. (1996). On pseudodata methods for LEWIS,P.A.W.andSHEDLER, G. S. (1979). Simulation of removing boundary effects in kernel density estimation.
Details
-
File Typepdf
-
Upload Time-
-
Content LanguagesEnglish
-
Upload UserAnonymous/Not logged-in
-
File Pages35 Page
-
File Size-