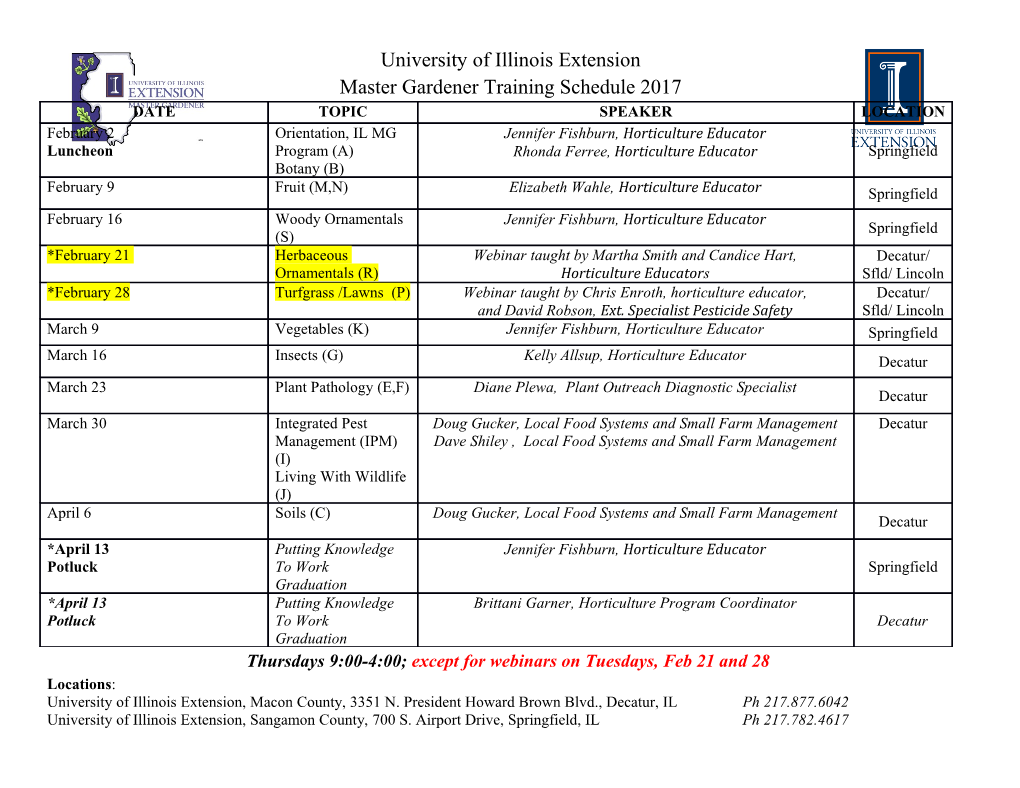
Martingale problems and stochastic equations • Characterizing stochastic processes by their martingale properties • Markov processes and their generators • Forward equations • Other types of martingale problems • Change of measure • Martingale problems for conditional distributions • Stochastic equations for Markov processes • Filtering • Time change equations • Bits and pieces • Supplemental material • Exercises • References •First •Prev •Next •Go To •Go Back •Full Screen •Close •Quit 1 Supplemental material • Background: Basics of stochastic processes • Stochastic integrals for Poisson random measures • Technical lemmas •First •Prev •Next •Go To •Go Back •Full Screen •Close •Quit 2 1. Characterizing stochastic processes by their martingale prop- erties • Levy’s´ characterization of Brownian motion • Definition of a counting process • Poisson processes • Martingale properties of the Poisson process • Strong Markov property for the Poisson process • Intensities • Counting processes as time changes of Poisson processes • Martingale characterizations of a counting process • Multivariate counting processes Jacod(1974/75),Anderson and Kurtz(2011, 2015) •First •Prev •Next •Go To •Go Back •Full Screen •Close •Quit 3 Brownian motion A Brownian motion is a continuous process with independent, Gaussian increments. A Brownian motion W is standard if the increments W (t + r) − W (t), t; r ≥ 0, have mean zero and variance r. W is a martingale: W W E[W (t + r)jFt ] = E[W (t + r) − W (t)jFt ] + W (t) = W (t); W Ft = σ(W (s): s ≤ t). W has quadratic variation t, that is X 2 [W ]t = lim (W (t ^ ti+1) − W (t ^ ti)) = t sup jti+1−tij=0 i in probability, or more precisely, X 2 lim sup j (W (t ^ ti+1) − W (t ^ ti)) − tj = 0 sup jti+1−tij=0 t≤T i in probability for each T > 0. W (t)2 − t is a martingale. •First •Prev •Next •Go To •Go Back •Full Screen •Close •Quit 4 L´evy’s characterization of Brownian motion Theorem 1.1 Let M be a continuous local martingale with [M]t = t. Then M is standard Brownian motion. Remark 1.2 Note that τn 2 τn E[M (t) ] = E[[M ]t] = E[τn ^ t] ≤ t τn 2 τn 2 and E[sups≤t M (s) ] ≤ 4E[M (t) ] ≤ 4t and it follows by the dominated convergence theorem and Fatou’s lemma that M is a square integrable martingale. •First •Prev •Next •Go To •Go Back •Full Screen •Close •Quit 5 Proof. Applying Ito’sˆ formula, Z t 1 Z t eiθM(t) = 1 + iθeiθM(s)dM(s) − θ2 eiθM(s)ds; 0 2 0 where the second term on the right is a martingale. Consequently, Z t+r iθM(t+r) iθM(t) 1 2 iθM(s) E[e jFt] = e − θ E[e jFt]ds 2 t and Z t+r iθ(M(t+r)−M(t) 1 2 iθM(s)−M(t) 't(θ; r) ≡ E[e jFt] = 1 − θ E[e jFt]ds 2 t Z r 1 2 = 1 − θ 't(θ; u)du 2 0 − 1 θ2r so 't(θ; r) = e 2 . It follows that for 0 = t0 < t1 < ··· < tm, m Y iθ (M(t )−M(t ) Y − 1 θ2(t −t ) E[ e k k k−1 ] = e 2 k k k−1 k=1 and hence M has independent Gaussian increments. •First •Prev •Next •Go To •Go Back •Full Screen •Close •Quit 6 Definition of a counting process Definition 1.3 N is a counting process if N(0) = 0 and N is constant except for jumps of +1. Dc[0; 1) will denote the space of possible counting paths that are finite for all time. c c D1[0; 1) ⊃ D [0; 1) is the larger space allowing the paths to hit infinity in finite time. •First •Prev •Next •Go To •Go Back •Full Screen •Close •Quit 7 Poisson processes A Poisson process is a model for a series for random observations occurring in time. For example, the process could model the arrivals of customers in a bank, the arrivals of telephone calls at a switch, or the counts registered by radiation detection equipment. x x x x x x x x t Let N(t) denote the number of observations by time t. In the figure above, N(t) = 6. Note that for t < s, N(s)−N(t) is the number of observations in the time interval (t; s]. We make the following assumptions about the model. 1) Observations occur one at a time. 2) Numbers of observations in disjoint time intervals are independent random variables, i.e., if t0 < t1 < ··· < tm, then N(tk) − N(tk−1), k = 1; : : : ; m are independent random variables. 3) The distribution of N(t + a) − N(t) does not depend on t. •First •Prev •Next •Go To •Go Back •Full Screen •Close •Quit 8 Characterization of a Poisson process Theorem 1.4 Under assumptions 1), 2), and 3), there is a constant λ > 0 such that, for t < s, N(s) − N(t) is Poisson distributed with parameter λ(s − t), that is, (λ(s − t))k P fN(s) − N(t) = kg = e−λ(s−t): k! k k+1 Proof. Let Nn(t) be the number of time intervals ( n ; n ]; k = 0;:::; [nt] that contain at least one observation. Then Nn(t) is binomially distributed with parameters n 1 and pn = P fN( n ) > 0g. Then n P fN(1) = 0g = P fNn(1) = 0g = (1 − pn) and npn ! λ ≡ − log P fN(1) = 0g, and the rest follows by standard Poisson approximation of the binomial. •First •Prev •Next •Go To •Go Back •Full Screen •Close •Quit 9 Interarrival times Let Sk be the time of the kth observation. Then k−1 X (λt)i P fS ≤ tg = P fN(t) ≥ kg = 1 − e−λt; t ≥ 0: k i! i=0 Differentiating to obtain the probability density function gives 1 λ(λt)k−1e−λt t ≥ 0 f (t) = (k−1)! Sk 0 t < 0 Theorem 1.5 Let T1 = S1 and for k > 1, Tk = Sk−Sk−1. Then T1;T2;::: are independent and exponentially distributed with parameter λ. •First •Prev •Next •Go To •Go Back •Full Screen •Close •Quit 10 Martingale properties of the Poisson process Theorem 1.6 (Watanabe) If N is a Poisson process with parameter λ, then N(t) − λt is a martingale. Conversely, if N is a counting process and N(t) − λt is a martingale, then N is a Poisson process. Proof. iθ(N(t+r)−N(t)) E[e jFt] n−1 X iθ(N(sk+1)−N(sk) iθ iθ(N(sk)−N(t)) = 1 + E[(e − 1 − (e − 1)(N(sk+1) − N(sk))e jFt] k=0 n−1 X iθ iθ(N(sk)−N(t)) + λ(sk+1 − sk)(e − 1)E[e jFt] k=0 The first term converges to zero by the dominated convergence theorem, so we have Z r iθ(N(t+r)−N(t)) iθ iθ(N(t+s)−N(t)) E[e jFt] = 1 + λ(e − 1) E[e jFt]ds 0 iθ(N(t+r)−N(t)) λ(eiθ−1)t and E[e jFt] = e . •First •Prev •Next •Go To •Go Back •Full Screen •Close •Quit 11 Strong Markov property A Poisson process N is compatible with a filtration fFtg, if N is fFtg-adapted and N(t + ·) − N(t) is independent of Ft for every t ≥ 0. Lemma 1.7 Let N be a Poisson process with parameter λ > 0 that is compatible with fFtg, and let τ be a fFtg-stopping time such that τ < 1 a.s. Define Nτ (t) = N(τ + t) − N(τ). Then Nτ is a Poisson process that is independent of Fτ and compatible with fFτ+tg. Proof. Let M(t) = N(t) − λt. By the optional sampling theorem, E[M((τ + t + r) ^ T )jFτ+t] = M((τ + t) ^ T ); so E[N((τ + t + r) ^ T ) − N((τ + t) ^ T )jFτ+t] = λ((τ + t + r) ^ T − (τ + t) ^ T ): By the monotone convergence theorem E[N(τ + t + r) − N(τ + t)jFτ+t] = λr which gives the lemma. •First •Prev •Next •Go To •Go Back •Full Screen •Close •Quit 12 Intensity for a counting process If N is a Poisson process with parameter λ and N is compatible with fFtg, then −λ∆t P fN(t + ∆t) > N(t)jFtg = 1 − e ≈ λ∆t: For a general counting process N, at least intuitively, a nonnegative, fFtg-adapted stochastic process λ(·) is an fFtg-intensity for N if Z t+∆t P fN(t + ∆t) > N(t)jFtg ≈ E[ λ(s)dsjFt] ≈ λ(t)∆t: t Let Sn be the nth jump time of N. Definition 1.8 λ is an fFtg-intensity for N if and only if for each n = 1; 2;:::. Z t^Sn N(t ^ Sn) − λ(s)ds 0 is a fFtg-martingale. •First •Prev •Next •Go To •Go Back •Full Screen •Close •Quit 13 Modeling with intensities Let Z be a stochastic process (cadlag, E-valued for simplicity) that models “exter- nal noise.” Let Dc[0; 1) denote the space of counting paths (zero at time zero and constant except for jumps of +1). Condition 1.9 c λ : [0; 1) × DE[0; 1) × D [0; 1) ! [0; 1) is measurable and satisfies λ(t; z; v) = λ(t; zt; vt), where zt(s) = z(s ^ t) (λ is nonantic- ipating), and Z t λ(s; z; v)ds < 1 0 c for all t ≥ 0, z 2 DE[0; 1) and v 2 D [0; 1). Let Y be a unit Poisson process that is fGug-compatible and assume that Z(s) is G0-measurable for every s ≥ 0.
Details
-
File Typepdf
-
Upload Time-
-
Content LanguagesEnglish
-
Upload UserAnonymous/Not logged-in
-
File Pages314 Page
-
File Size-