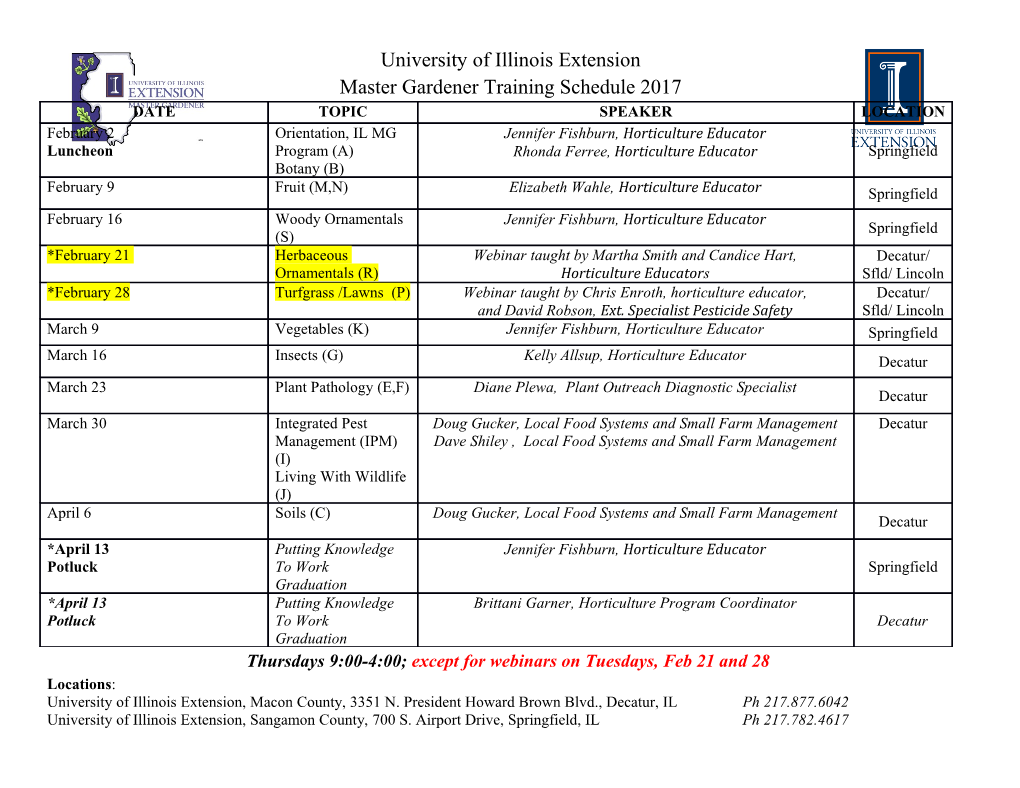
Confidence Distribution Xie and Singh (2013): Confidence distribution, the frequentist distribution estimator of a parameter: A Review Céline Cunen, 15/09/2014 Outline of Article ● Introduction ● The concept of Confidence Distribution (CD) ● A classical Definition and the History of the CD Concept ● A modern definition and interpretation ● Illustrative examples ● Basic parametric examples ● Significant (p-value) functions ● Bootstrap distributions ● Likelihood functions ● Asymptotically third-order accurate confidence distributions ● CD, Bootstrap, Fiducial and Bayesian approaches ● CD-random variable, Bootstrap estimator and fiducial-less interpretation ● CD, fiducial distribution and Belief function ● CD and Bayesian inference ● Inferences using a CD ● Confidence Interval ● Point estimation ● Hypothesis testing ● Optimality (comparison) of CDs ● Combining CDs from independent sources ● Combination of CDs and a unified framework for Meta-Analysis ● Incorporation of Expert opinions in clinical trials ● CD-based new methodologies, examples and applications ● CD-based likelihood caluculations ● Confidence curve ● CD-based simulation methods ● Additional examples and applications of CD-developments ● Summary CD: a sample-dependent distribution that can represent confidence intervals of all levels for a parameter of interest ● CD: a broad concept = covers all approaches that can build confidence intervals at all levels Interpretation ● A distribution on the parameter space ● A Distribution estimator = contains information for many types of inference ● An estimator FOR the parameter of interest, instead of an inherent distribution OF the parameter ● Purely frequentist: – The parameter is a fixed, non-random quantity – 95% CI: the true parameter value will be covered by the CIs 95% of the time Fiducial Inference ● “Fisher's biggest blunder”? ● Fiducial – Faith – A fiducial distribution: describes the level of “faith” attached to different values of the unknown parameter ● Sometimes normalized likelihood function are interpreted as distributions of the parameter – ~ the parameters are both fixed and random quantities ● Interpretation of 95% CI: there is a 95% probability that the parameter lies inside the CI. 2.2 Modern definition ● A function Hn() is called a confidence distribution for a parameter θ if: – R1: Hn() is a cummulative distribution function on the parameter space – R2: at the true parameter value θ = θ0 Hn(θ0) as a function of the sample x follows the uniform distribution U[0,1] ● R2 is important! 2.3.1: Basic parametric examples ● A sample: – With σ² known ● The function satisfies the requirements in the CD-definition – R1: it clearly is a cdf – R2: 2.3.2 p-value functions ● One-sided test H0: θ ≤ b vs H1: θ > b ● P-value function: ● Usually CDs or (aCDs): – Because cdf – And because when b=θ0, H0 is true and p-values are uniformly distributed when H0 is true. 2.3.3 Bootstrap distributions ● True (unknown) parameter ● Original sample, with estimator ● Bootstrap sample: a sample of equal size as the original sample, sampled with replacement from the original sample ● Compute the estimator on each bootstrap samples (get many ) --> the Bootstrap Distribution ● The Bootstrap Distribution is an aCD! 2.3.4 Likelihood functions ● Under some mild conditions: normalized likelihood functions are density functions of asymptotic normal CDs – Method for obtaining CDs from likelihoods ● CD-based inference ≈ likelihood inference 3.1 CD-random Variable, ... ● The CD-random variable: – The CD is not a distribution of θ! ● CD-random variable = Bootstrap estimator, and this is useful because it: – Help understanding CD-inference and develop new methods – Clarifies the interpretation of CDs: CDs are not distributions of θ, so therefore it is not a problem that a transformation g(θ) of θ does not generally lead to a CD for g(θ) 3.2 CD, Fiducial Distribution... ● Methods from fiducial reasoning (and from CDs) are supposed to have good statistical performance in the frequentist sense – CIs should have the exact coverage property – For tests: the actual rate of type I error is equal to the specified level of the test ● Many fiducial distributions are CDs ● Fiducial reasoning: provides a procedure for finding CDs 3.3 CD and Bayesian Inference ● Bayesian credible intervals to not possess the exact coverage property – But asymptotically they can obtain it – Then: posterior distributions are aCDs – Bayes methods can produce CDs! ● Benefits with CBs compared to bayesian methods: – Nuisance parameters 4 Inferences using a CD ● CDs contain information for any type of frequentist inference 4.1 Confidence Intervals ● CDs allows us to construct CIs for all levels of α ● CI constructed from CDs have the exact coverage property: 4.2 Point Estimation ● Median: ● Mean: ● Mode: ● Under some conditions, these are consistent estimators (= converging in probability to the true value) 4.3 Hypothesis Testing ● One-sided test: – The support on C: – Reject H0 if the support on C is less than α – The rejection region corresponds to a level α test – Support = p-value (often) ● Two-sided test – Same story, but with a more complicated rejection region 5 Optimality of CDs ● Can have multiple CDs for the same parameter ● A better CD = a CD more concentrated around the true parameter value 6.1 Combination of CDs ... ● k independent studies, estimate the same parameter of interest θ ● Study i with sample xi and CD Hi(.) ● Propose a general recipe for combining k independent CDs: Combining k p-values - “Fisher's method” ● k p-values from k independent studies ● Under H0: ● Remember that ● So that the teststatistic ● Will be distributed under H0 Example ● 2 studies aim to estimate the μ parameter from a normal model with known sigmas ● Study 1: n1=30, ● Study 2: n2=40, ● With CDs: ● We choose ● And then 6.2 Incorporation of Expert Opinions ● How can one incorporate existing knowledge in an analysis? – Bayesian approaches: prior = existing knowledge – In a frequentist setting: CD! ● CD-approach: – CDe: summarizes the existing information/opinions – CDd: from the data – Combines these two by the methods in the last section ● Advantages: – Easy to implement/ computationally cheap – No need for priors on nuisance parameter! – Avoids “the discrepant posterior phenomenon” 7 New methodologies, Examples... ● Different CD-related methods: – Obtaining likelihood functions from CDs – Presenting CDs: the confidence curve – CD-based simulation methods 8 Summary ● CDs is a broad concept which “contains” many well-known notions and results ● Most types of frequentist inference can be derived from CDs ● Advantages of the CD-approach: – Handles nuisance parameters well – Easy to combine information ● Problems (need of further study): – Multivariate CDs – Cases where it difficult to obtain CDs – Model uncertainty/ diagnosis/ selection .
Details
-
File Typepdf
-
Upload Time-
-
Content LanguagesEnglish
-
Upload UserAnonymous/Not logged-in
-
File Pages26 Page
-
File Size-