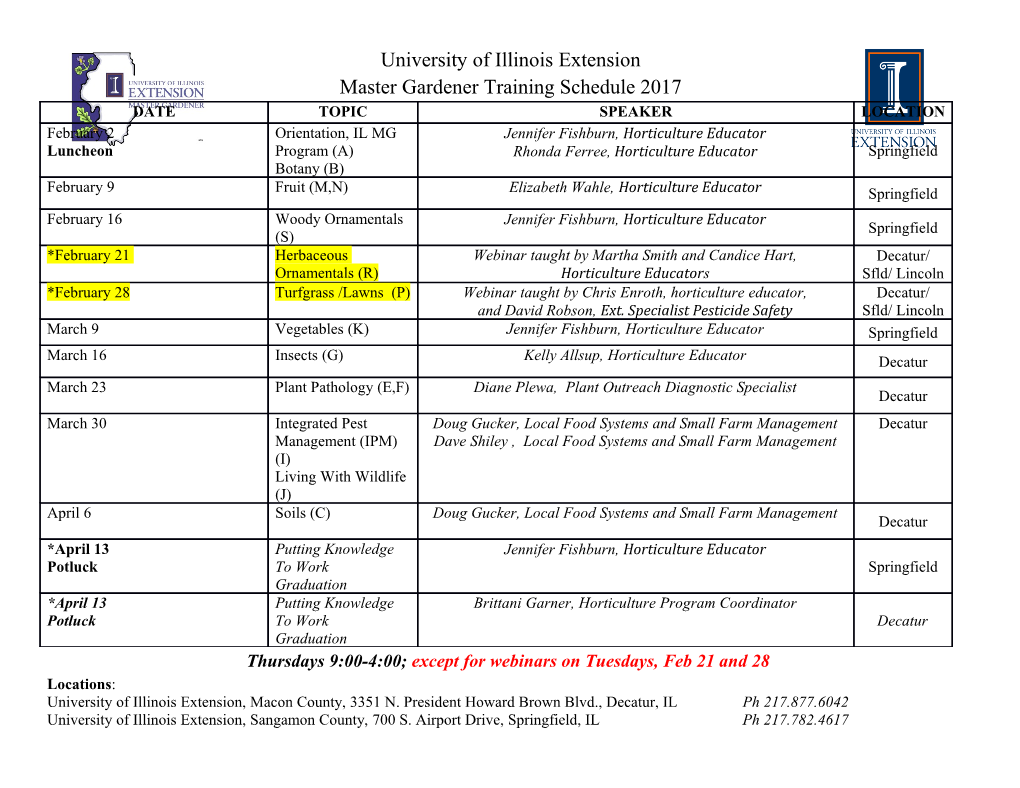
Marginal Likelihood from the Gibbs Output Siddhartha Chib Journal of the American Statistical Association, Vol. 90, No. 432. (Dec., 1995), pp. 1313-1321. Stable URL: http://links.jstor.org/sici?sici=0162-1459%28199512%2990%3A432%3C1313%3AMLFTGO%3E2.0.CO%3B2-2 Journal of the American Statistical Association is currently published by American Statistical Association. Your use of the JSTOR archive indicates your acceptance of JSTOR's Terms and Conditions of Use, available at http://www.jstor.org/about/terms.html. JSTOR's Terms and Conditions of Use provides, in part, that unless you have obtained prior permission, you may not download an entire issue of a journal or multiple copies of articles, and you may use content in the JSTOR archive only for your personal, non-commercial use. Please contact the publisher regarding any further use of this work. Publisher contact information may be obtained at http://www.jstor.org/journals/astata.html. Each copy of any part of a JSTOR transmission must contain the same copyright notice that appears on the screen or printed page of such transmission. The JSTOR Archive is a trusted digital repository providing for long-term preservation and access to leading academic journals and scholarly literature from around the world. The Archive is supported by libraries, scholarly societies, publishers, and foundations. It is an initiative of JSTOR, a not-for-profit organization with a mission to help the scholarly community take advantage of advances in technology. For more information regarding JSTOR, please contact [email protected]. http://www.jstor.org Wed Feb 6 14:39:37 2008 Marginal Likelihood From the Gibbs Output Siddhartha CHIB In the context of Bayes estimation via Gibbs sampling, with or without data augmentation, a simple approach is developed for computing the marginal density of the sample data (marginal likelihood) given parameter draws from the posterior distribution. Consequently, Bayes factors for model comparisons can be routinely computed as a by-product of the simulation. Hitherto, this calculation has proved extremely challenging. Our approach exploits the fact that the marginal density can be expressed as the prior times the likelihood function over the posterior density. This simple identity holds for any parameter value. An estimate of the posterior density is shown to be available if all complete conditional densities used in the Gibbs sampler have closed-form expressions. To improve accuracy, the posterior density is estimated at a high density point, and the numerical standard error of resulting estimate is derived. The ideas are applied to probit regression and finite mixture models. KEY WORDS: Bayes factor; Estimation of normalizing constant; Finite mixture models; Linear regression; Markov chain Monte Carlo; Markov mixture model; Multivariate density estimation; Numerical standard error; Probit regression; Reduced conditional density. 1. INTRODUCTION (1994) showed that the marginal likelihood (equivalently, the marginal density of y) under model Mk, that is, The advent of Markov chain Monte Carlo (MCMC) meth- ods (Gelfand and Smith 1990, Tanner and Wong 1987) to simulate posterior distributions has virtually revolutionized the practice of Bayesian statistics. For the most part, these methods have been used for estimation and out-of-sample can be estimated as prediction, because both of those problems are easily solved given a sample of draws from the posterior distribution. On the other hand, the problem of calculating the marginal like- lihood, which is the normalizing constant of the posterior density and an input to the computation of Bayes factors which is the harmonic mean of the likelihood values. Al- (see, for example, Berger 1985, Kass and Raftery 1995, or though this estimate is a simulation-consistent estimate of O'Hagan 1994), has proved extremely challenging. This is m(y1 Mk), it is not stable, because the inverse likelihood because the marginal likelihood is obtained by integrating does not have finite variance. But consider the quantity the likelihood function with respect to the prior density, proposed by Gelfand and Dey (1993): whereas the MCMC method produces draws from the pos- terior. One way to deal with this problem is to compute Bayes factors without attempting to calculate the marginal like- lihood by introducing a model indicator into the list of unknown parameters. Work along these lines has been re- where p(0)is a density with tails thinner than the product of ported by Carlin and Polson (1991), Carlin and Chib (1995), the prior and the likelihood. This can be shown to have the and many others. To use these methods, however, it is nec- property that ~ G +D m(yMk) as G becomes large without essary to specify all of the competing models at the out- the instability of mNR.Nonetheless, this approach requires set, which may not be always possible, and to carefully a tuning function, which can be quite difficult to determine specify certain tuning constants to ensure that the simula- in high-dimensional problems, and subsequent monitoring tion algorithm mixes suitably in model space. In this arti- to ensure that the numbers are stable. In fact, we have cle, therefore, we concern ourselves with methods that di- found that the somewhat obvious choices of p(.)-a nor- rectly address the calculation of the marginal likelihood. ma1 density or t density with mean and covariance equal Suppose that f (yOk,Mk) is the density function of the to the posterior mean and covariance--do not necessarily satisfy the thinness requirement. Other attempts to mod- data y = (yl,. ,y,) under model Mk (k = 1,2,. ,K) given the model-specific parameter vector Ok. Let the prior ify the harmonic mean estimator, though requiring samples from both the prior and posterior distributions, have been density of Ok (assumed to be proper) be given by ~(0k1 Mk), discussed by Newton and Raftery (1994). and let {QP)}I {Of),' ' ' 7 ':6)} be draws from the The purpose of this article is to demonstrate that a sim- posterior T(Ok~,Mk) using a MCMC ple approach to the marginal likelihood and the say the Gibbs Newton and Raftery Bayes factor is available that is free of the problems just de- scribed. This approach is developed in the setting where the Siddhartha Chib is Professor of Econometrics, John M. Olin School of Business, Washington University, St. Louis, MO 63130. This article has benefited from valuable comments of two anonymous referees, the @ 1995 Aiiierican Statistical Association associate editor, and the editor. In addition, discussions with Jim Albert, Journal of the American Statistical Association Ed Greenberg, and Radford Neal are gratefully acknowledged. December 1995, Vol. 90, No. 432, Theory and Methods 1313 Journal of the American Statistical Association, December 1995 Gibbs sampling algorithm, with or without data augmenta- rived, as shown in Section 3. It is now time to examine the tion, has been used to provide a sample of draws from the method for calculating the posterior density estimate from posterior distribution. To compute the marginal density by the Gibbs output. our approach, it is necessary that all integrating constants of the full conditional distributions in the Gibbs sampler be 2.1 Estimation of ~(O*ly). known. This requirement is usually satisfied in models fit Consider now the estimation of the multivariate density with conjugate priors and covers almost all applications of T(O* y) and the selection of the point O*. As was pointed the Gibbs sampler that have appeared in the literature. out, the BMI expression holds for any 0,and thus the choice The rest of the article is organized as follows. Section 2 of the point is not critical, but efficiency considerations dic- presents the approach, and Section 3 illustrates the deriva- tate that for a given number of posterior draws, the density tion of the numerical standard error of the estimate. Section is likely to be more accurately estimated at a high density 4 presents applications of the approach, first for variable point, where more samples are available, than at a point in selection in probit regression and then for model compar- the tails. It should be noted that a modal value such as the isons in finite mixture models. The final section contains posterior mode, or the maximum likelihood estimate, can brief concluding remarks. be computed from the Gibbs output, at least approximately, if it is easy to evaluate the log-likelihood function for each 2. THE APPROACH draw in the simulation. Alternatively, one can make use of Suppress the model index k and consider the situation the posterior mean provided that there is no concern that it wherein f (y 0)is the sampling density (likelihood function) is a low density point. for the given model and ~(0)is the prior density. To allow We now explain how the posterior density ordinate can for the possibility that posterior simulation requires data be estimated from the Gibbs output, starting with a canoni- augmentation, let z denote latent data and suppose that for cal situation consisting of two blocks of parameters before a given set of vector blocks 0 = (01,02,. ,OB), the Gibbs turning to the general case. We show that the proposed sampling algorithm is applied to the set of (B + 1) com- multivariate density estimation method is easy to imple- plete conditional densities, ment, requires only the available complete conditional den- sities, and produces a simulation consistent estimate of the posterior ordinate. The objective is to compute the marginal density m(y Mk) from the output {o(~),z(g))globtained from (4). 2.1.1 Two Vector Blocks. Suppose that Gibbs sampling The approach developed here consists of two related is applied to the complete conditional densities ideas. First, m(y),by virtue of being the normalizing con- stant of the posterior density, can be written as which is the setting of Tanner and Wong (1987).
Details
-
File Typepdf
-
Upload Time-
-
Content LanguagesEnglish
-
Upload UserAnonymous/Not logged-in
-
File Pages10 Page
-
File Size-