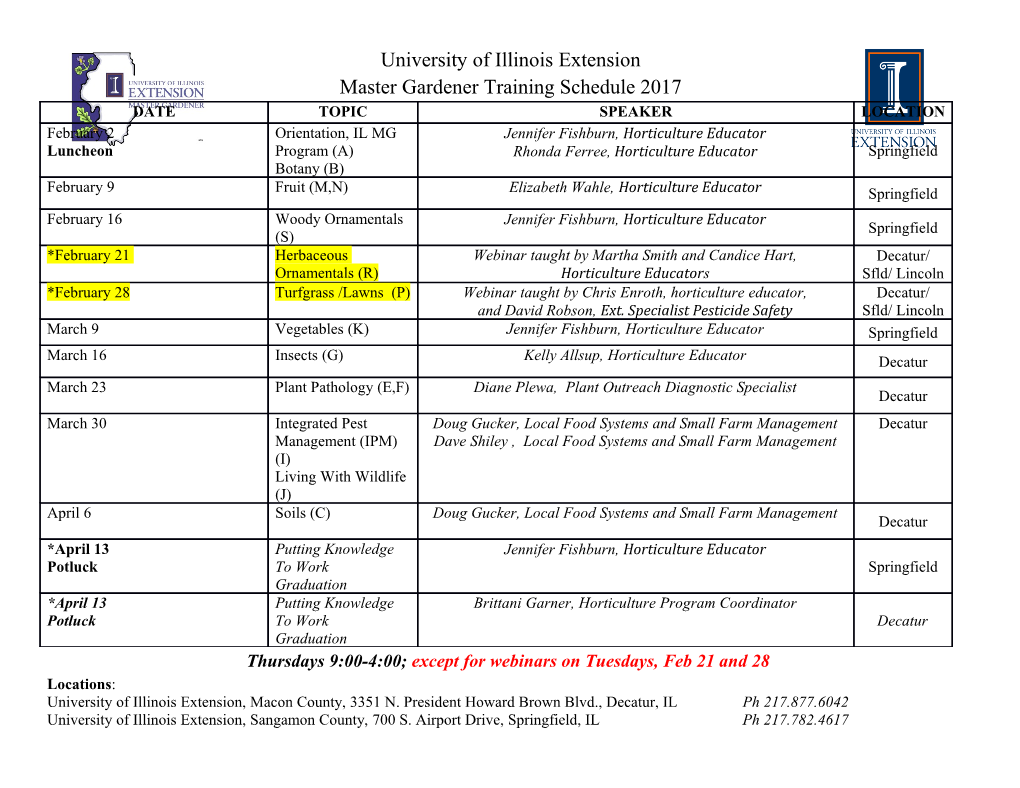
Visual Data Mining: Background, Techniques, and Drug Discovery Applications Mihael Ankerst The Boeing Company Georges Grinstein UMass Lowell and AnVil Inc. Daniel Keim AT&T Research and University of Konstanz A color version of the tutorial notes can be found via http://www.fmi.uni-konstanz.de/~keim KDD’2002 Conference Emails and URLs Data Exploration • Definition Mihael Ankerst – [email protected] Data Exploration is the process of searching and analyzing – http://www.visualclassification.com/ankerst databases to find implicit but potentially useful information Daniel A. Keim – [email protected] • more formally – [email protected] Data Exploration is the process of finding a – http://www.fmi.uni-konstanz.de/~keim • subset D‘ of the database D and George Grinstein – [email protected] • hypotheses Hu(D‘,C) – http://genome.uml.edu that a user U considers useful in an application context C – http://www.anvilinfo.com Mihael Ankerst, The Boeing Company -- Daniel A. Keim, AT&T and Univ. of Konstanz Mihael Ankerst, The Boeing Company -- Daniel A. Keim, AT&T and Univ. of Konstanz Georges Grinstein, UMass Lowell and AnVil Inc. 2 Georges Grinstein, UMass Lowell and AnVil Inc. 5 Overview Abilities of Humans and Computers Part I: Visualization Techniques 1. Introduction 2. Visual Data Exploration Techniques abilities of Data Storage 3. Distortion and Interaction Techniques the computer Numerical Computation 4. Visual Data Mining Systems Searching Part II: Specific Visual Data Mining Techniques 1. Association Rules Planning 2. Classification Diagnosis Logic 3. Clustering Prediction 4. Text Mining 5. Tightly Integrated Visualization Perception Part III: Drug Discovery Applications Creativity 1. Biology and Chemistry General Knowledge 2. Bioinformatics and Cheminformatics 3. Examples human abilities 4. Bioinformatics Packages 5. Cheminformatics Packages Mihael Ankerst, The Boeing Company -- Daniel A. Keim, AT&T and Univ. of Konstanz Mihael Ankerst, The Boeing Company -- Daniel A. Keim, AT&T and Univ. of Konstanz Georges Grinstein, UMass Lowell and AnVil Inc. 3 Georges Grinstein, UMass Lowell and AnVil Inc. 6 Goals of Visualization Techniques Brief Historical Overview of Exploratory Data Visualization Techniques (cf. [WB 95]) • Presentation • pioneering work of Tufte [Tuf 83, Tuf 90] and Bertin [Ber 81] – starting point: facts to be presented are fixed a priori focuses on – process: choice of appropriate presentation techniques – result: high-quality visualization of the data to present facts – visualization of data with inherent 2D-/3D-semantics • Confirmatory Analysis – general rules for layout, color composition, attribute mapping, etc. – starting point: hypotheses about the data • development of visualization techniques for different types – process: goal-oriented examination of the hypotheses of data with an underlying physical model – result: visualization of data to confirm or reject the hypotheses – geographic data, CAD data, flow data, image data, voxel data, etc. • Exploratory Analysis – starting point: no hypotheses about the data • development of visualization techniques for arbitrary – process: interactive, usually undirected search for structures, trends multidimensional data (without an underlying physical model) – result: visualization of data to lead to hypotheses about the data – applicable to databases and other information resources Mihael Ankerst, The Boeing Company -- Daniel A. Keim, AT&T and Univ. of Konstanz Mihael Ankerst, The Boeing Company -- Daniel A. Keim, AT&T and Univ. of Konstanz Georges Grinstein, UMass Lowell and AnVil Inc. 4 Georges Grinstein, UMass Lowell and AnVil Inc. 7 1 Data Preprocessing Techniques Overview • Techniques for Dimension Reduction Part I: Visualization Techniques (Set of d-dim Data Items -> Set of k-dim. Data Items; k<<d) 1. Introduction 2. Visual Data Exploration Techniques • Principal Component Analysis [DE 82] 3. Distortion and Interaction Techniques Determines a minimal set of principal components (linear combinations of the 4. Visual Data Mining Systems original dimensions) which explain the main variations of the data. Part II: Specific Visual Data Mining Techniques • Factor Analysis [Har 67] 1. Association Rules Determines a set of unobservable common factors which explain the main 2. Classification variations of the data. The original dimensions are linear combinations of the 3. Clustering common factors. 4. Text Mining • Multidimensional Scaling [SRN 72] 5. Tightly Integrated Visualization Uses the similarity (or dissimilarity) matrix of the data as defining coordinate Part III: Drug Discovery Applications axes in multidimensional space. The Euclidean distance in that space is a 1. Biology and Chemistry measure of the data items. 2. Bioinformatics and Cheminformatics • Fastmap [FL 95] 3. Examples Fastmap also operates on a given similarity matrix and iteratively reduces the 4. Bioinformatics Packages number of dimensions while preserving the distances as much as possible. 5. Cheminformatics Packages Mihael Ankerst, The Boeing Company -- Daniel A. Keim, AT&T and Univ. of Konstanz Mihael Ankerst, The Boeing Company -- Daniel A. Keim, AT&T and Univ. of Konstanz Georges Grinstein, UMass Lowell and AnVil Inc. 8 Georges Grinstein, UMass Lowell and AnVil Inc. 11 Data Preprocessing Techniques Visual Data Exploration Techniques • Subsetting Techniques (Set of Data Items -> Subset of Data Items) – Sampling (determines a representative subset of a database) – Querying (determines a certain, usually a-priori fixed subset of the • Standard 2D/3D Displays database • Segmentation Techniques • Geometric Transformations (Set of Data-Items -> Set of (Set of Data Items)) – Segmentation based upon attribute values or attribute ranges • Iconic Displays • Aggregation Techniques (Set of Data-Items -> Set of Aggregate Values) • Dense Pixel Displays – Aggregation (sum, count, min, max,...) based upon - attribute values • Stacked Displays - topological properties, etc. – Visualization of Aggregations: - Histograms - Pie Charts, Bar Charts, Line Graphs, etc. Mihael Ankerst, The Boeing Company -- Daniel A. Keim, AT&T and Univ. of Konstanz Mihael Ankerst, The Boeing Company -- Daniel A. Keim, AT&T and Univ. of Konstanz Georges Grinstein, UMass Lowell and AnVil Inc. 9 Georges Grinstein, UMass Lowell and AnVil Inc. 12 Classification Standard 2D/3D Displays Data Type to be Visualized Examples from the VisualInsights WebPage from the VisualInsights Examples 1. one-dimensional 2. two-dimensional Visualization Technique 3. mul ti -di mensional Stacked Display 4. text/web Dense Pixel Display Iconic Display 5. hierarchies/graphs Geometrically-transformed Display 6. algorithm/software Standard 2D/3D Display Standard Projection FilteringZoom Di st ort ion Link&Brush Interaction and Distortion Technique Mihael Ankerst, The Boeing Company -- Daniel A. Keim, AT&T and Univ. of Konstanz Mihael Ankerst, The Boeing Company -- Daniel A. Keim, AT&T and Univ. of Konstanz Georges Grinstein, UMass Lowell and AnVil Inc. 10 Georges Grinstein, UMass Lowell and AnVil Inc. 13 2 Geometric Transformations Geometric Transformations Basic Idea: Prosection Views [FB 94, STDS 95] n o d n Visualization of geometric transformations and o L e g e l l projections of the data o C l a i r e p m I , e c n • Scatterplot-Matrices [And 72, Cle 93] e p S . R f o n o • Landscapes [Wis 95] i s s i m r e p • Projection Pursuit Techniques [Hub 85] y b d e s used used by permission of R. Spence, Imperial London College (D techniques for finding meaningful projections of multidimensional data) u • Prosection Views [FB 94, STDS 95 schematic representation example • Hyperslice [WL 93] matrix of all orthogonal projections where the result of the selected multidimensional range is colored differently • Parallel Coordinates [Ins 85, ID 90] (combination of selections and projections) Mihael Ankerst, The Boeing Company -- Daniel A. Keim, AT&T and Univ. of Konstanz Mihael Ankerst, The Boeing Company -- Daniel A. Keim, AT&T and Univ. of Konstanz Georges Grinstein, UMass Lowell and AnVil Inc. 14 Georges Grinstein, UMass Lowell and AnVil Inc. 17 Geometric Transformations Geometric Transformations Hyperslice [ 93] Scatterplot-Matrices [Cle 93] matrix of scatterplots (x-y-diagrams) of the k-dim. data [total of (k2/2-k) scatterplots] ermission of Ward,M. Polytechnic Worcester Institute used used by permission of J. J. van Wijk Used byUsed matrix of k² slices through the k-dim. Data (the slices are determined interactively) Mihael Ankerst, The Boeing Company -- Daniel A. Keim, AT&T and Univ. of Konstanz Mihael Ankerst, The Boeing Company -- Daniel A. Keim, AT&T and Univ. of Konstanz Georges Grinstein, UMass Lowell and AnVil Inc. 15 Georges Grinstein, UMass Lowell and AnVil Inc. 18 Geometric Transformations Geometric Transformations Landscapes [Wis 95] Parallel Coordinates [Ins 85, ID 90] n equidistant axes which are parallel to one of the screen axes and correspond to the attributes the axes are scaled to the [minimum, maximum] - range of the news articles corresponding attribute visualized as a landscape every data item corresponds to a polygonal line which intersects each of the axes at the point which corresponds to the value for the attribute Used by permissionUsed of Wright,B. Decisions Visible Inc. • • • • visualization of the data as perspective landscape • the data needs to be transformed into a (possibly artificial) 2D spatial representation which preserves the characteristics Attr. 1 Attr. 2Attr. 3 Attr. k of the data Mihael
Details
-
File Typepdf
-
Upload Time-
-
Content LanguagesEnglish
-
Upload UserAnonymous/Not logged-in
-
File Pages69 Page
-
File Size-