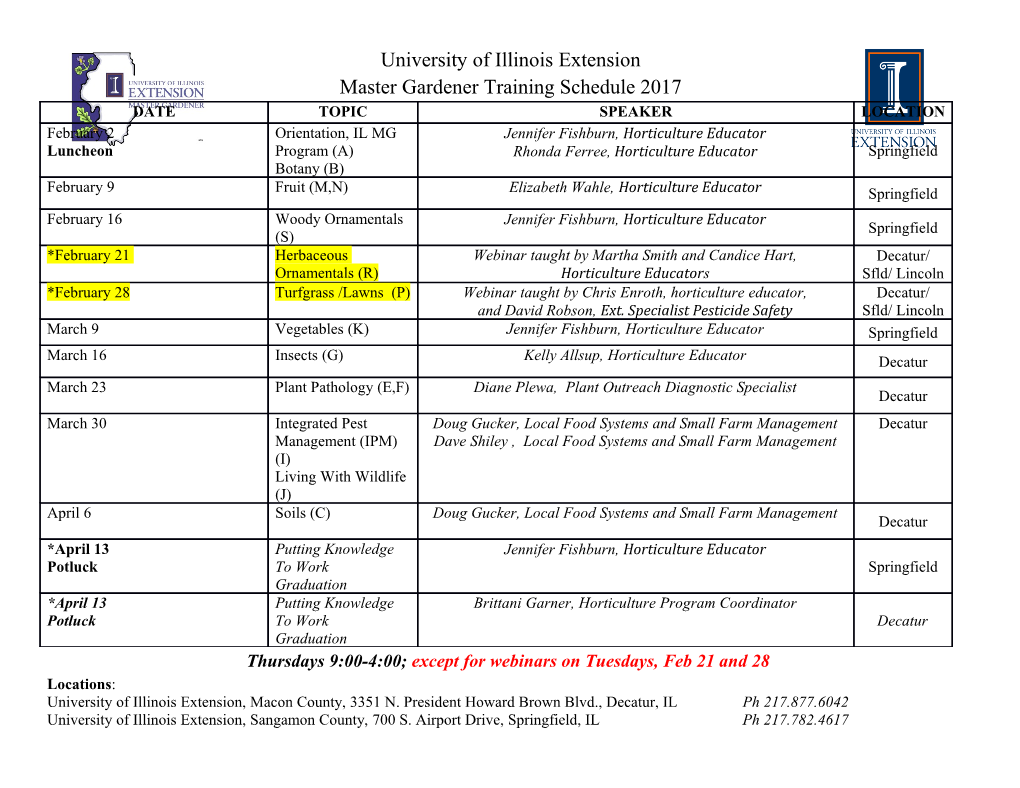
1 Cramer-Rao´ and moment-entropy inequalities for Renyi entropy and generalized Fisher information Erwin Lutwak, Deane Yang, and Gaoyong Zhang Abstract— The moment-entropy inequality shows that a con- Analogues for convex and star bodies of the moment- tinuous random variable with given second moment and maximal entropy, Fisher information-entropy, and Cramer-Rao´ inequal- Shannon entropy must be Gaussian. Stam’s inequality shows that ities had been established earlier by the authors [3], [4], [5], a continuous random variable with given Fisher information and minimal Shannon entropy must also be Gaussian. The Cramer-´ [6], [7] Rao inequality is a direct consequence of these two inequalities. In this paper the inequalities above are extended to Renyi II. DEFINITIONS entropy, p-th moment, and generalized Fisher information. Gen- Throughout this paper, unless otherwise indicated, all inte- eralized Gaussian random densities are introduced and shown to be the extremal densities for the new inequalities. An extension of grals are with respect to Lebesgue measure over the real line the Cramer–Rao´ inequality is derived as a consequence of these R. All densities are probability densities on R. moment and Fisher information inequalities. Index Terms— entropy, Renyi entropy, moment, Fisher infor- A. Entropy mation, information theory, information measure The Shannon entropy of a density f is defined to be Z h[f] = − f log f, (1) I. INTRODUCTION R provided that the integral above exists. For λ > 0 the λ-Renyi HE moment-entropy inequality shows that a continuous entropy power of a density is defined to be random variable with given second moment and maximal T 1 Shannon entropy must be Gaussian (see, for example, Theorem Z 1−λ λ 9.6.5 in [1]). This follows from the nonnegativity of the f if λ 6= 1, N [f] = (2) relative entropy of two continuous random variables. In this λ R h[f] paper we introduce the notion of relative Renyi entropy for two e if λ = 1, random variables and show that it is always nonnegative. We provided that the integral above exists. Observe that identify the probability distributions that have maximal Renyi entropy with given p–th moment and call them generalized lim Nλ[f] = N1[f]. λ→1 Gaussians. The λ–Renyi entropy of a density f is defined to be In his proof of the Shannon entropy power inequality Stam [2] shows that a continuous random variable with given Fisher hλ[f] = log Nλ[f]. information and minimal Shannon entropy must be Gaussian. We introduce below a generalized form of Fisher information The entropy hλ[f] is continuous in λ and, by the Holder¨ associated with Renyi entropy and that is, in some sense, dual inequality, decreasing in λ. It is strictly decreasing, unless f to the p–th moment. A generalization of Stam’s inequality is is a uniform density. established. The probability distributions that have maximal Renyi entropy with given generalized Fisher information are B. Relative entropy the generalized Gaussians. Given two densities f, g : R → R, their relative Shannon The Cramer-Rao´ inequality (see, for example, Theorem entropy or Kullback–Leibler distance [11], [12], [13] (also, see 12.11.1 in [1]) states that the second moment of a continuous page 231 in [1]) is defined by random variable is bounded from below by the reciprocal Z f of its Fisher information. We use the moment and Fisher h1[f, g] = f log , (3) g information inequalities to establish a generalization of the R Cramer–Rao´ inequality, where a lower bound is obtained for provided that the integral above exists. Given λ > 0 and the p-th moment of a continuous random variable in terms two densities f and g, we define the relative λ–Renyi entropy of its generalized Fisher information. Again, the generalized power of f and g as follows. If λ 6= 1, then 1 1 Gaussians are the extremal distributions. Z 1−λ Z λ gλ−1f gλ E. Lutwak ([email protected]), D. Yang ([email protected]), and N [f, g] = R R , G. Zhang ([email protected]) are with the Department of Mathematics, λ 1 (4) Z λ(1−λ) Polytechnic University, Brooklyn, New York and were supported in part by f λ NSF Grant DMS-0104363. R 2 and, if λ = 1, then information of a density f by φp,λ[f] and define it as follows. If p ∈ (1, ∞), let q ∈ (1, ∞] satisfy p−1 +q−1 = 1, and define h1[f,g] N1[f, g] = e , Z φ [f]qλ = |f λ−2f 0|qf, (7) provided in both cases that the righthand side exists. Define p,λ R the λ–Renyi relative entropy of f and g by provided that f is absolutely continuous, and the norm above λ is finite. If p = 1, then φp,λ[f] is defined to be the essential hλ[f, g] = log Nλ[f, g]. supremum of |f λ−2f 0| on the support of f, provided f is Observe that hλ[f, g] is continuous in λ. absolutely continuous, and the essential supremum is finite. λ Lemma 1: If f and g are densities such that hλ[f], hλ[g], If p = ∞, then φp,λ[f] is defined to be the total variation λ λ and hλ[f, g] are finite, then of f /λ, provided that f has bounded variation. (see, for example, [17] for a definition of “bounded variation”). hλ[f, g] ≥ 0. Note that our definition of generalized Fisher information Equality holds if and only if f = g. has a different normalization than the standard definition. In particular, the classical Fisher information corresponds to the Proof: The case λ = 1 is well–known (see, for example, square of (2, 1)-th Fisher information, as defined above. page 234 in [1]). The remaining cases are a direct consequence The Fisher information φ [f] is continuous in (p, λ). For of the Holder¨ inequality. If λ > 1, then we have p,λ a given λ it is, by the Holder¨ inequality, decreasing in p. λ−1 1 Z Z λ Z λ λ−1 λ λ g f ≤ g f , E. Generalized Gaussian densities R R R Given t ∈ , let and if λ < 1, then we have R Z Z t+ = max{t, 0}. f λ = (gλ−1f)λgλ(1−λ) Let R R Z ∞ Z λ Z 1−λ t−1 −x λ−1 λ Γ(t) = x e dx ≤ g f g . 0 R R denote the Gamma function, and let The equality conditions follow from the equality conditions of Γ(a)Γ(b) the Holder¨ inequality. β(a, b) = Γ(a + b) denote the Beta function. C. The p-th moment For each p ∈ [0, ∞] and λ > 1 − p, we define the For p ∈ (0, ∞) define p-th moment of a density f to be corresponding generalized Gaussian density G : R → [0, ∞) Z as follows. If p ∈ (0, ∞), then G is defined by p µp[f] = |x| f(x) dx, (5) ( 1 a (1 + (1 − λ)|x|p) λ−1 if λ 6= 1, R G(x) = p,λ + (8) −|x|p provided that the integral above exists. For p ∈ [0, ∞] define ap,1e if λ = 1, the p-th deviation by where 1 Z p(1 − λ) p if λ < 1, exp f(x) log |x| dx if p = 0, 1 1 1 2β( p , 1−λ − p ) R 1 p σ [f] = p (6) if λ = 1, p (µp[f]) if 0 < p < ∞, ap,λ = 1 2Γ( p ) 1 ess sup{|x| : f(x) > 0} if p = ∞, p(λ − 1) p if λ > 1. 2β( 1 , λ ) provided in each case that the right side is finite. The deviation p λ−1 σp[f] is continuous in p and, by the Holder¨ inequality, strictly If p = 0 and λ > 1, then G is defined for almost every x ∈ R increasing in p. by 1 λ−1 G(x) = a0,λ(− log |x|)+ , D. The (p, λ)-th Fisher information where 1 Recall that the classical Fisher information [14], [15], [16] a0,λ = . 2Γ( λ ) of a density f : R → R is given by λ−1 Z If p = ∞ and λ > 0, then G is defined by 2 −1 0 2 φ2,1[f] = f |f | , 1 if |x| ≤ 1 R 2 provided f is absolutely continuous, and the integral exists. G(x) = If p ∈ [1, ∞] and λ ∈ R, we denote the (p, λ)-th Fisher 0 if |x| > 1. 3 1 For consistency we shall also denote a∞,λ = 2 . We will also need the following simple scaling identities: For t > 0, define G : → [0, ∞) by t R Z Z Gλ = t1−λ Gλ, (14) Gt(x) = G(x/t)/t. (9) t R R Sz. Nagy [8] established a family of sharp Gagliardo- and Nirenberg inequalities on R and their equality conditions. His results can be used to prove Theorem 3 and identify σp[Gt] = tσp[G]. (15) the generalized Gaussians as the extremal densities for the inequalities proved in this paper. Later, Barenblatt [9] showed that the generalized Gaussians are also the self–similar so- III. THE MOMENT INEQUALITY lutions of the Lp porous media and fast diffusion equations. Generalized Gaussians are also the 1-dimensional versions of It is well known that among all probability distributions with the extremal functions for sharp Sobolev, log-Sobolev, and given second moment, the Gaussian is the unique distribution Gagliardo-Nirenberg inequalities (see, for example, [10]). that maximizes the Shannon entropy. This follows from the positivity of the relative entropy of a given distribution and F.
Details
-
File Typepdf
-
Upload Time-
-
Content LanguagesEnglish
-
Upload UserAnonymous/Not logged-in
-
File Pages6 Page
-
File Size-